The Sum Of The Probabilities In The Distribution Is .
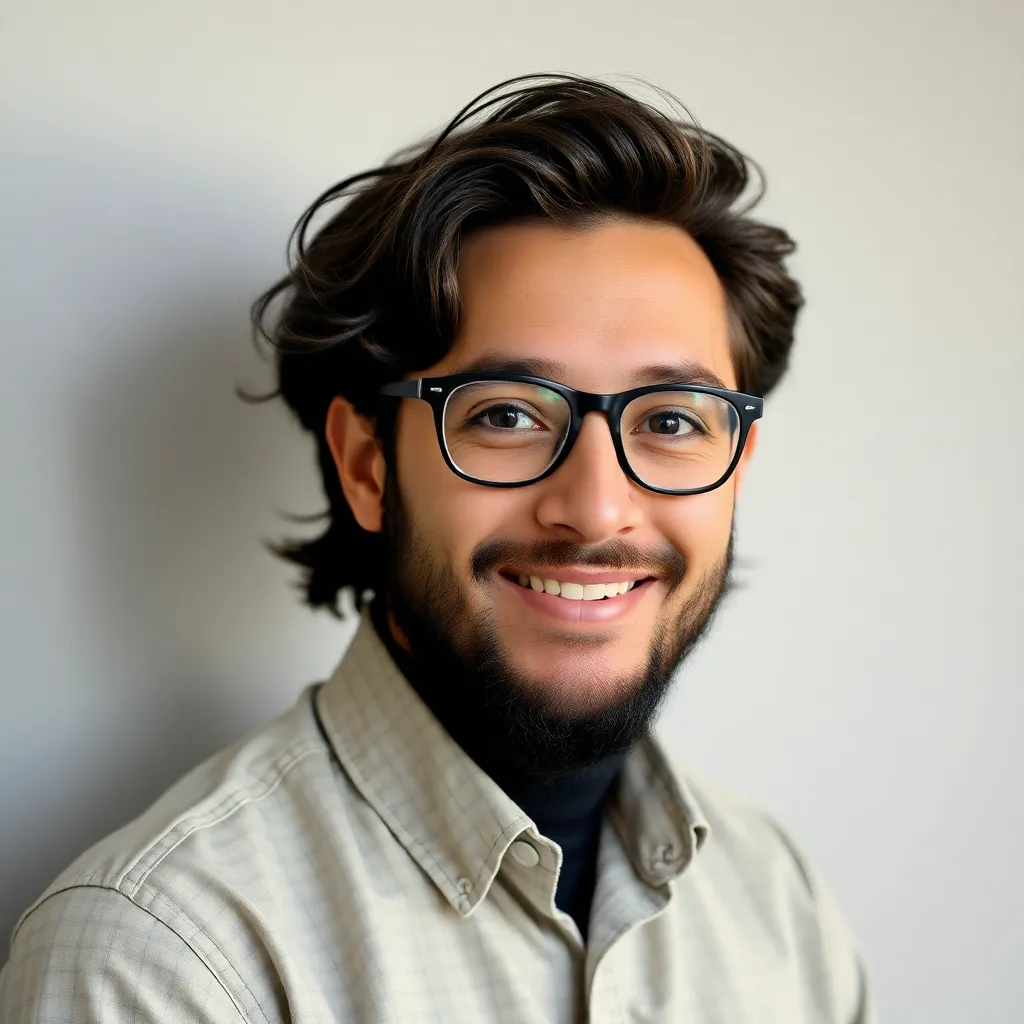
listenit
Mar 30, 2025 · 6 min read
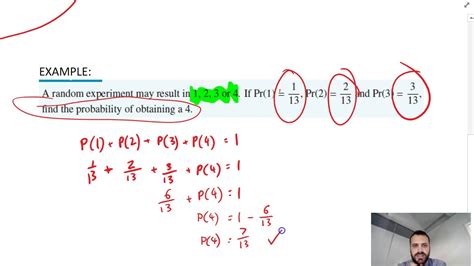
Table of Contents
The Sum of Probabilities in a Distribution: A Comprehensive Guide
The fundamental principle underlying probability distributions is that the sum of all individual probabilities within the distribution must equal one. This seemingly simple statement holds profound implications for statistical analysis, modeling, and interpretation. This article delves deep into this critical concept, exploring its theoretical basis, practical applications, and the consequences of deviations from this fundamental rule. We'll cover various types of distributions, discuss how to verify this principle, and examine scenarios where the sum might not be exactly one, and how to handle such situations.
Understanding Probability Distributions
Before diving into the sum of probabilities, let's establish a clear understanding of probability distributions. A probability distribution is a mathematical function that provides the probabilities of occurrence of different possible outcomes for a random variable. The random variable could represent anything from the height of individuals in a population to the number of heads observed in multiple coin tosses. The distribution describes the likelihood of each possible value of this variable.
There are numerous types of probability distributions, each with its own unique characteristics and applications. Some of the most common include:
-
Discrete Probability Distributions: These distributions describe discrete random variables, meaning the variable can only take on a finite number of values or a countably infinite number of values. Examples include the binomial distribution (modeling the number of successes in a fixed number of trials), the Poisson distribution (modeling the number of events occurring in a fixed interval of time or space), and the hypergeometric distribution (modeling sampling without replacement).
-
Continuous Probability Distributions: These distributions describe continuous random variables, meaning the variable can take on any value within a given range. Examples include the normal distribution (the ubiquitous bell curve), the exponential distribution (modeling the time until an event occurs), and the uniform distribution (where all values within a range have equal probability).
Regardless of the type of distribution, the core principle remains the same: the sum of all probabilities must equal one. This reflects the certainty that one of the possible outcomes must occur.
The Sum of Probabilities: The Axiom of Probability
The statement that the sum of probabilities in a distribution equals one is not merely an empirical observation; it's a fundamental axiom of probability theory. This axiom, often expressed mathematically as:
∑P(X = xᵢ) = 1 (for discrete distributions)
or
∫f(x)dx = 1 (for continuous distributions)
where:
- P(X = xᵢ) represents the probability of the random variable X taking on the value xᵢ.
- f(x) represents the probability density function (pdf) for the continuous random variable X.
- The summation (∑) is over all possible values of xᵢ.
- The integration (∫) is over the entire range of possible values of x.
This axiom ensures that the probability distribution is properly normalized. A normalized distribution guarantees that the total probability mass is distributed across all possible outcomes, leaving no gaps or overlaps. It represents the completeness of the model: all possibilities are accounted for.
Verifying the Sum of Probabilities
Verifying that the sum of probabilities equals one is crucial when working with probability distributions. This verification serves as a check for the accuracy and validity of the distribution's parameters and calculations. The methods for verification vary depending on the type of distribution:
For Discrete Distributions: The verification is straightforward. Simply sum all the individual probabilities. If the sum is exactly 1, the distribution is properly normalized. For example, consider a simple distribution representing the outcome of rolling a fair six-sided die:
P(X=1) = 1/6 P(X=2) = 1/6 P(X=3) = 1/6 P(X=4) = 1/6 P(X=5) = 1/6 P(X=6) = 1/6
The sum: 1/6 + 1/6 + 1/6 + 1/6 + 1/6 + 1/6 = 1
For Continuous Distributions: Verification involves integrating the probability density function over its entire range. This often requires calculus. For example, the standard normal distribution's probability density function is:
f(x) = (1/√(2π)) * e^(-x²/2)
Integrating this function from negative infinity to positive infinity yields 1, confirming its normalization. However, for many continuous distributions, the integral can be complex and may require specialized software or numerical methods for accurate evaluation.
Consequences of a Sum Not Equal to One
If the sum of probabilities in a distribution doesn't equal one, it indicates a problem with the model. The most common causes include:
-
Incorrect Calculation: Simple calculation errors are the most frequent culprits. Double-checking your calculations is essential.
-
Incomplete Model: The model might not account for all possible outcomes. There might be missing scenarios or overlooked possibilities that should be included.
-
Incorrect Parameters: The parameters of the distribution (e.g., mean, variance) might have been incorrectly estimated or specified.
-
Sampling Bias: If the distribution is derived from a sample, sampling bias could lead to inaccurate probabilities.
If the sum is less than one, it signifies that some probability mass is missing. If it's greater than one, it indicates an invalid probability distribution, as probabilities cannot exceed one. Addressing these issues requires careful review of the model's assumptions, calculations, and data sources. In some cases, adjustments to the parameters might be necessary; in others, a complete re-evaluation of the model might be required.
Handling Situations Where the Sum is Not Exactly One
In practice, due to rounding errors in calculations or limitations in numerical methods, the sum might not be exactly one, but very close to it (e.g., 0.9999 or 1.0001). In such cases, a small discrepancy is usually acceptable. However, large deviations from one require investigation and correction. Techniques for handling these minor discrepancies include:
-
Normalization: The probabilities can be normalized by dividing each probability by the sum of all probabilities. This scales the probabilities proportionally to ensure they sum to one.
-
Iterative Refinement: In situations involving complex models or simulations, iterative refinement of parameters or algorithms can gradually improve the accuracy and bring the sum of probabilities closer to one.
-
Error Analysis: Performing a thorough error analysis to identify the source of the discrepancy can guide in finding the solution.
Practical Applications
The principle that the sum of probabilities equals one has far-reaching consequences across various fields:
-
Statistical Inference: In hypothesis testing and confidence interval construction, the normalization of probability distributions is crucial for making valid inferences.
-
Risk Management: In financial modeling and risk assessment, properly normalized probability distributions are essential for accurate risk quantification and decision-making.
-
Machine Learning: Many machine learning algorithms rely on probability distributions, and the proper normalization of these distributions is crucial for the algorithm's performance and accuracy.
-
Simulation and Modeling: In simulations involving random processes, the accurate representation of probability distributions is essential for the reliability and validity of the simulation results.
Conclusion
The fundamental principle that the sum of probabilities in a distribution equals one is a cornerstone of probability theory and statistics. It ensures that probability models are complete, consistent, and provide a coherent representation of uncertainty. Verifying this principle and understanding the consequences of deviations are vital for the accuracy and validity of statistical analyses, simulations, and modeling efforts across diverse disciplines. The ability to identify and address instances where the sum deviates from one demonstrates a solid understanding of probability distributions and the necessary analytical rigor for accurate results. Remembering this simple yet powerful principle is crucial for anyone working with probability and statistics.
Latest Posts
Latest Posts
-
What Is The Molar Mass Of No2
Apr 01, 2025
-
27 To The Power Of 1 3
Apr 01, 2025
-
How Many Centimeters Are Equal To 4 Meters
Apr 01, 2025
-
9 To The 3 2 Power
Apr 01, 2025
-
What Element Is Found In Carbohydrates
Apr 01, 2025
Related Post
Thank you for visiting our website which covers about The Sum Of The Probabilities In The Distribution Is . . We hope the information provided has been useful to you. Feel free to contact us if you have any questions or need further assistance. See you next time and don't miss to bookmark.