How Does Outliers Affect The Mean
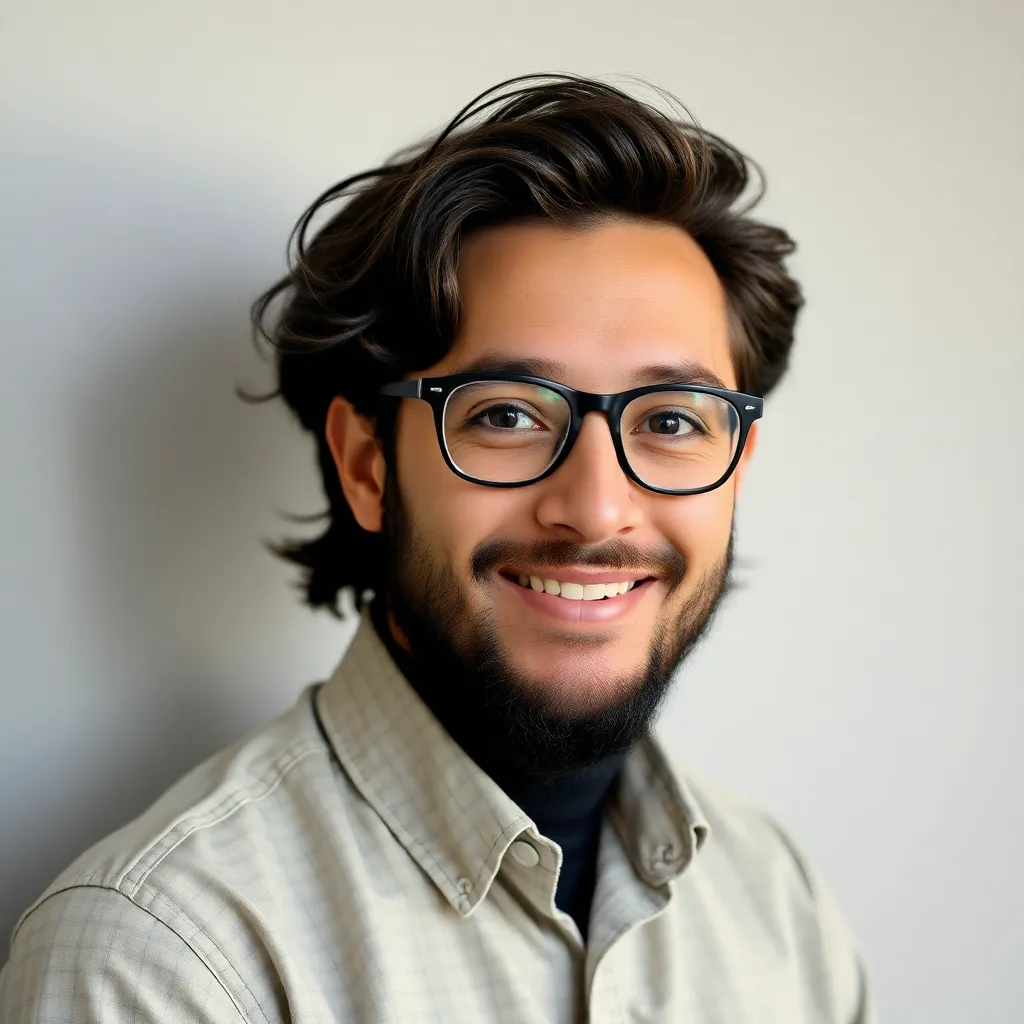
listenit
Apr 05, 2025 · 6 min read
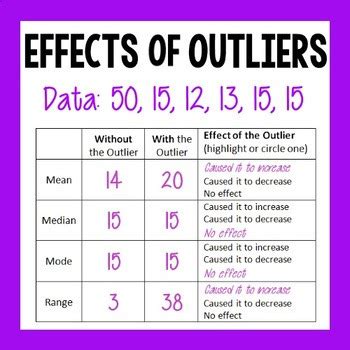
Table of Contents
How Outliers Affect the Mean: A Deep Dive into Statistical Analysis
Understanding how outliers affect the mean is crucial for accurate data interpretation and reliable statistical analysis. Outliers, those data points significantly deviating from the rest of the dataset, can severely distort the mean, leading to misleading conclusions. This comprehensive guide delves into the mechanics of this distortion, explores methods for outlier detection and handling, and discusses the implications for various statistical applications.
What are Outliers?
An outlier is a data point that significantly differs from other observations in a dataset. This difference can be due to various factors, including:
- Data entry errors: Simple mistakes in recording data can lead to outliers.
- Measurement errors: Faulty equipment or inaccurate measurement techniques can produce erroneous values.
- Sampling errors: The chosen sample might not accurately represent the entire population.
- Natural variation: In some cases, extreme values are genuinely part of the population's natural variability, although they are rare.
Identifying outliers isn't always straightforward. There's no single definitive threshold. However, several methods help in their detection, which we'll discuss later.
The Mean: A Vulnerable Statistic
The mean, or average, is calculated by summing all data points and dividing by the number of data points. While simple to calculate, the mean is highly sensitive to outliers. A single extreme value can significantly inflate or deflate the mean, making it a poor representation of the central tendency in the presence of outliers.
Example:
Consider the dataset: 10, 12, 15, 14, 16, 11, 13, 100. The mean is 22.625. However, if we remove the outlier (100), the mean becomes 12.875, a considerable difference. This illustrates how a single outlier can drastically alter the mean, making it an unreliable measure of central tendency in such cases.
Mechanisms of Outlier Influence on the Mean
Outliers exert their influence on the mean due to the arithmetic nature of its calculation. Since the mean involves summing all values, an extreme value, whether exceptionally high or low, disproportionately contributes to the sum. This effect is amplified as the magnitude of the outlier increases and the sample size decreases. In smaller datasets, a single outlier can have a more dramatic impact compared to a larger dataset where its effect is diluted somewhat.
Identifying Outliers: Common Methods
Several methods help identify potential outliers. No single method is perfect, and the best approach often involves combining multiple techniques:
-
Visual inspection (Box plots and Scatter plots): Box plots visually represent data quartiles, highlighting potential outliers beyond the "whiskers." Scatter plots can reveal outliers as isolated points far from the main data cluster.
-
Z-score: This method measures how many standard deviations a data point is from the mean. Data points with a Z-score exceeding a certain threshold (e.g., ±3) are often considered outliers. However, this method is sensitive to the distribution of the data and might not be suitable for skewed datasets.
-
Modified Z-score: This is a robust alternative to the standard Z-score, less sensitive to outliers in the calculation of the mean and standard deviation.
-
Interquartile Range (IQR): The IQR is the difference between the third quartile (Q3) and the first quartile (Q1) of the data. Outliers are often defined as data points below Q1 – 1.5 * IQR or above Q3 + 1.5 * IQR. This method is robust against skewed data.
-
DBSCAN (Density-Based Spatial Clustering of Applications with Noise): A powerful algorithm for clustering data points. Data points that don't belong to any cluster are identified as outliers. This method is particularly useful for high-dimensional data.
Handling Outliers: Strategies and Considerations
Once outliers are identified, decisions must be made on how to handle them. There's no universally correct approach; the best strategy depends on the context and the reasons behind the outliers.
-
Removal: Simply removing outliers is the most drastic approach. However, it should only be done if the outliers are clearly due to errors (e.g., data entry mistakes) and removing them doesn't significantly bias the analysis. Always justify the removal and document the process.
-
Transformation: Transforming the data (e.g., using logarithmic or square root transformations) can sometimes reduce the influence of outliers. This approach is particularly useful when the data is heavily skewed.
-
Winsorizing: This method replaces extreme values with less extreme ones (often the values at a certain percentile). This reduces the impact of outliers while retaining more information than removal.
-
Robust statistics: Employing robust statistical measures, less sensitive to outliers, such as the median instead of the mean, is a powerful alternative. The median is the middle value when data is ordered, not affected by extreme values. Other robust measures include trimmed mean and the median absolute deviation (MAD).
-
Modeling: Incorporate outliers into the model explicitly. Certain statistical models, like regression models, can account for outliers without requiring their removal or transformation. Specific techniques include robust regression methods that are less sensitive to outliers.
-
Further investigation: If the outliers represent genuine data points, explore the reasons for their extreme values. Often, these points might offer valuable insights that would otherwise be lost by simply ignoring or removing them.
Implications for Statistical Analysis
The presence of outliers has significant implications for various statistical analyses:
-
Regression analysis: Outliers can heavily influence the regression line, leading to inaccurate predictions and misleading interpretations of relationships between variables.
-
Hypothesis testing: Outliers can inflate the variance, affecting the power of statistical tests and potentially leading to incorrect conclusions about the significance of results.
-
Confidence intervals: Outliers can widen confidence intervals, making estimates less precise.
-
Data visualization: Outliers can distort the appearance of data visualizations, making it difficult to discern patterns and trends.
Case Studies: Real-World Examples
The impact of outliers is readily apparent in numerous real-world scenarios:
-
Financial data: Stock market crashes or unusual trading activity can create outliers that significantly impact average returns and risk assessments.
-
Medical research: Extreme values in patient data (e.g., blood pressure, heart rate) can significantly skew the results of clinical trials.
-
Environmental monitoring: Anomalous readings from sensors measuring pollution levels might indicate equipment malfunction or unusual environmental events.
-
Sales data: A single exceptionally large sale can distort average sales figures, potentially misleading business decisions.
Conclusion: Responsible Data Handling
Outliers are a ubiquitous phenomenon in data analysis. Understanding their influence on the mean and other statistical measures is essential for accurate and reliable results. While they might represent genuine data or errors, responsible handling requires careful consideration of the context and the potential consequences of ignoring or mishandling them. Employing appropriate detection methods, selecting suitable handling strategies, and using robust statistical techniques are crucial for drawing valid conclusions and avoiding misleading interpretations based on distorted means. The choice of handling method depends heavily on the context and the potential reasons for the outliers. Careful consideration and documentation of all decisions related to outlier handling are crucial for maintaining the integrity and trustworthiness of statistical analysis. Remember, outliers can sometimes reveal important insights and unexpected phenomena within the dataset. Don't dismiss them without a thorough investigation.
Latest Posts
Latest Posts
-
What Is 15 Percent Of 38
Apr 06, 2025
-
Write The Formula Formula Unit For The Following Compounds
Apr 06, 2025
-
21 Is 42 Of What Number
Apr 06, 2025
-
Initial Value And Rate Of Change
Apr 06, 2025
-
500 Ml Equals How Many Oz
Apr 06, 2025
Related Post
Thank you for visiting our website which covers about How Does Outliers Affect The Mean . We hope the information provided has been useful to you. Feel free to contact us if you have any questions or need further assistance. See you next time and don't miss to bookmark.