What Is The Value Of R 2 3 4 5
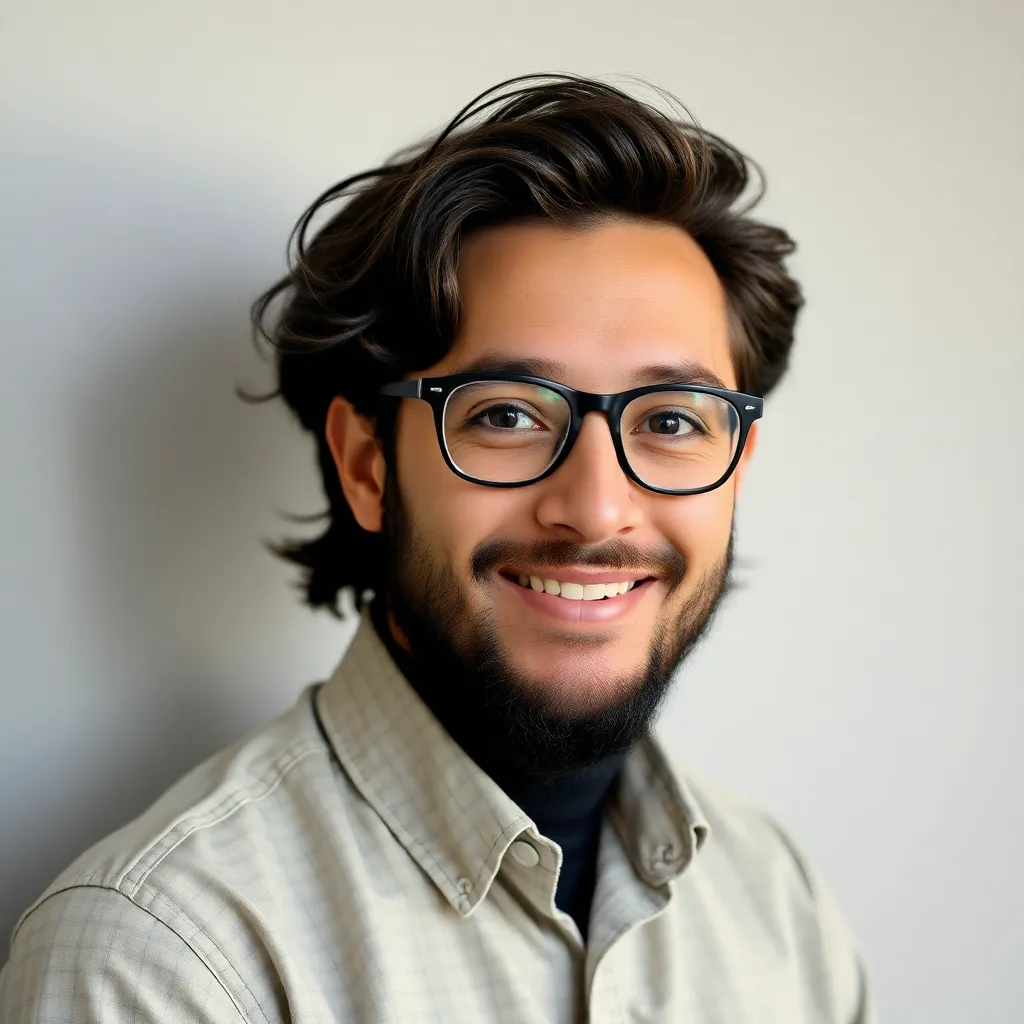
listenit
Mar 28, 2025 · 6 min read
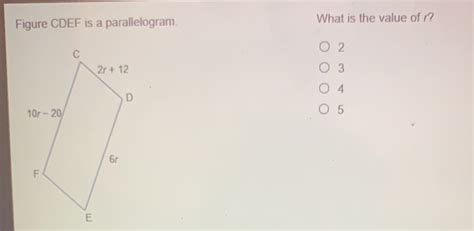
Table of Contents
What is the Value of R^2, R^3, R^4, and R^5? Understanding Higher-Order Correlation Measures
The familiar R-squared (R²) statistic, a cornerstone of regression analysis, measures the goodness of fit of a model. It quantifies the proportion of variance in the dependent variable explained by the independent variables. However, the concept of R-squared doesn't stop at the second power. While less commonly used, higher-order R values like R³, R⁴, and R⁵ offer potentially valuable insights, albeit with caveats and limitations. This article delves into the meaning, interpretation, and application of these higher-order correlation measures.
Understanding R-Squared (R²)
Before venturing into the complexities of R³, R⁴, and R⁵, it's crucial to firmly grasp the foundation: R². R² represents the coefficient of determination, indicating the percentage of the total variation in the dependent variable that's predictable from the independent variables. A higher R² generally suggests a better model fit, implying the independent variables explain a larger portion of the dependent variable's variability. However, it's crucial to remember that a high R² doesn't automatically equate to a good model. Overfitting, where a model fits the training data too closely but generalizes poorly to new data, can lead to artificially inflated R² values.
Limitations of R²
- Overfitting: As mentioned, overfitting is a significant concern. A complex model with numerous independent variables might achieve a very high R² on the training data but perform poorly when encountering new, unseen data.
- Ignoring Model Complexity: R² doesn't inherently penalize model complexity. Adding more independent variables will generally increase R², even if those variables are irrelevant. This is why adjusted R² is often preferred, as it accounts for the number of predictors in the model.
- Non-linear Relationships: R² is most effective for linear relationships. If the relationship between the variables is non-linear, R² might not accurately reflect the model's goodness of fit.
Exploring Higher-Order R Values: R³, R⁴, and R⁵
The concept of extending R² to higher powers – R³, R⁴, R⁵, and so on – arises from the potential to capture more nuanced aspects of the relationship between variables. While not standard practice, these higher-order values can provide additional insights, particularly in situations where simple linear relationships are insufficient.
R³ (R Cubed): A Deeper Dive into Variance Explained
R³ isn't directly interpretable as a percentage of variance explained in the same way as R². Instead, it represents the cube of the correlation coefficient (R). This means it amplifies the effect of the correlation. A small positive correlation can result in a very small R³, while a strong positive correlation will result in a much larger R³. Similarly, a strong negative correlation will result in a large negative R³. Therefore, R³ helps in identifying the strength and direction of the correlation more emphatically than R².
Practical Applications: R³ could be relevant when analyzing situations with strong non-linear relationships or when dealing with skewed data distributions. The amplified effect can highlight subtle differences in correlation strength that might be missed with R².
R⁴ (R to the Power of Four): Emphasizing Strength of Association
Similar to R³, R⁴ further amplifies the effects of the correlation. Its value is the fourth power of the correlation coefficient. This means that even small changes in the correlation coefficient are magnified substantially. This magnification can be particularly useful in identifying very strong or very weak correlations more explicitly. However, interpreting the absolute value of R⁴ in terms of variance explained becomes increasingly complex and less intuitive.
Practical Applications: In fields like finance or risk assessment, where even small differences in correlation can have significant implications, R⁴ might provide a more sensitive measure of the association between variables.
R⁵ (R to the Power of Five): Extreme Amplification and Its Limitations
R⁵ takes the amplification effect to an extreme. The fifth power significantly magnifies even minor changes in the correlation coefficient. This extreme magnification, while potentially revealing subtle patterns, also makes it highly sensitive to outliers and prone to misinterpretation. The interpretation in terms of variance explained is even less straightforward than for R³ and R⁴.
Practical Applications and Caveats: While potentially useful in niche applications involving highly sensitive data, the extreme amplification of R⁵ makes it highly susceptible to noise and outliers. It's crucial to exercise caution when using R⁵ and to carefully validate the results with other statistical measures.
Comparing R², R³, R⁴, and R⁵: A Summary Table
R Value | Interpretation | Strengths | Weaknesses |
---|---|---|---|
R² | Proportion of variance explained | Widely understood, widely used | Sensitive to overfitting, doesn't account for model complexity |
R³ | Cubed correlation coefficient; amplifies effects | Highlights strength and direction; useful for non-linearity | Less intuitive than R²; no direct variance explanation |
R⁴ | Fourth power of correlation; extreme amplification | Extremely sensitive to correlation strength | Extremely sensitive to outliers; little direct interpretation |
R⁵ | Fifth power of correlation; extreme amplification | Can reveal very subtle patterns | Highly susceptible to noise and outliers; complex interpretation |
Practical Considerations and Alternatives
While higher-order R values might offer additional insights in specific contexts, their practical application is limited due to the challenges in interpretation and sensitivity to outliers. It's vital to carefully consider the following points:
- Data Quality: Ensure the data is clean, accurate, and free from significant outliers. Outliers can disproportionately influence higher-order R values.
- Model Selection: The choice of model plays a crucial role. A poorly chosen model will yield unreliable results regardless of the R value used.
- Contextual Interpretation: The meaning and significance of higher-order R values are highly context-dependent. Carefully consider the specific application and the implications of using these less conventional metrics.
- Alternative Approaches: Instead of relying on higher-order R values, consider exploring alternative methods like non-linear regression techniques, robust regression, or techniques that address multicollinearity. These methods offer more robust and interpretable results.
Conclusion
While R², R³, R⁴, and R⁵ all stem from the correlation coefficient, their interpretations and applications diverge significantly. R² remains the dominant and readily interpretable measure of goodness of fit in linear regression. Higher-order R values offer a potential avenue for examining more nuanced relationships, particularly in situations with strong non-linearity or when subtle correlation differences are critical. However, their sensitivity to outliers and challenges in interpretation necessitate caution in their application. Careful consideration of data quality, model selection, and contextual understanding are paramount when venturing beyond the well-established framework of R². Prioritizing robust statistical methods and a thorough understanding of the data remain crucial for drawing reliable and meaningful conclusions. In many cases, focusing on refining model selection and utilizing appropriate regression techniques provides a more robust and interpretable approach than relying on the less common higher-order R values.
Latest Posts
Latest Posts
-
What Are The Four Agents Of Erosion
Mar 31, 2025
-
What Is The Gcf Of 72 And 60
Mar 31, 2025
-
Finding The Radius Of A Circle From The Circumference
Mar 31, 2025
-
In Which Layer Does Weather Occur
Mar 31, 2025
-
What Is The Opposite Of 9
Mar 31, 2025
Related Post
Thank you for visiting our website which covers about What Is The Value Of R 2 3 4 5 . We hope the information provided has been useful to you. Feel free to contact us if you have any questions or need further assistance. See you next time and don't miss to bookmark.