What Is The Point Estimate For The Population Mean
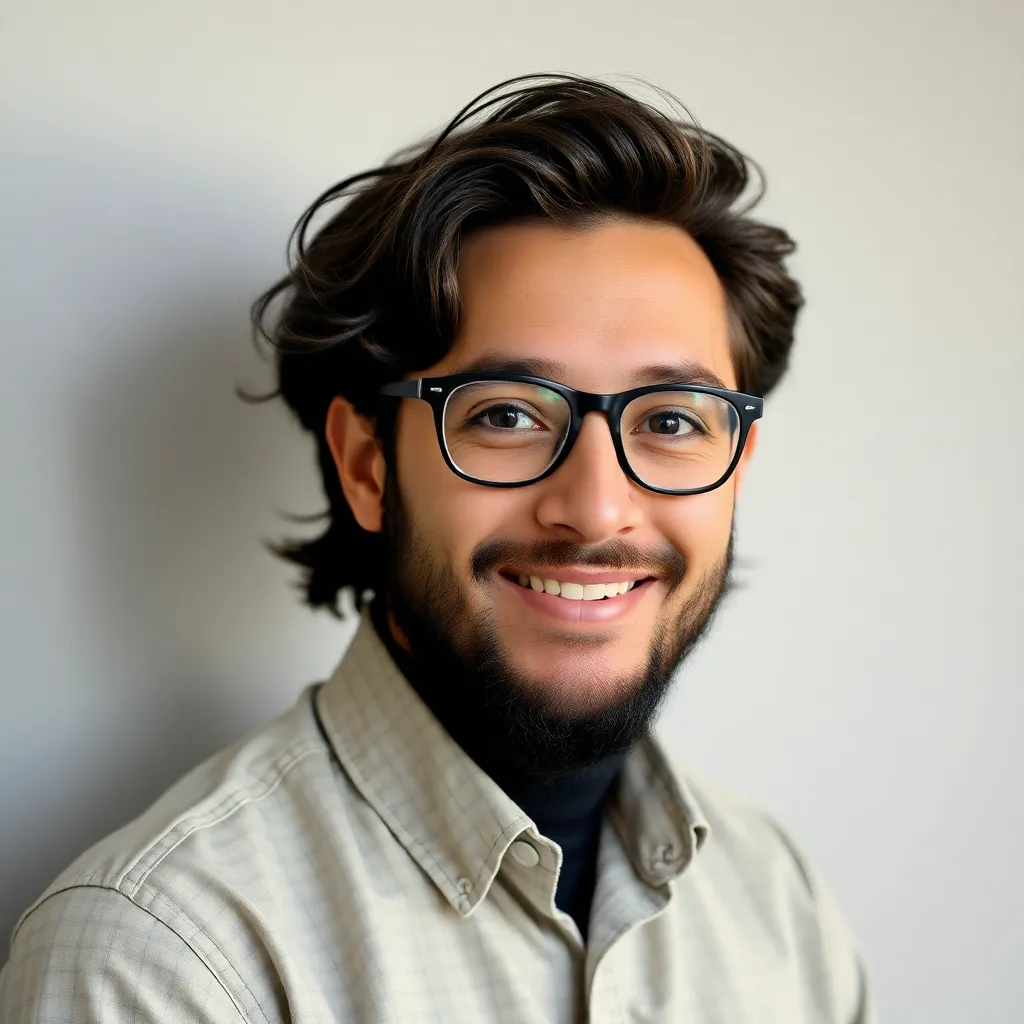
listenit
Apr 15, 2025 · 6 min read

Table of Contents
What is the Point Estimate for the Population Mean? A Comprehensive Guide
Understanding population parameters is crucial in statistics. Often, we can't directly measure the entire population due to cost, time, or practical limitations. Instead, we rely on samples to estimate these parameters. One of the most important parameters is the population mean (µ), representing the average value of a variable across the entire population. Estimating this mean from a sample leads us to the concept of a point estimate. This article delves deep into what a point estimate for the population mean is, how it's calculated, its limitations, and different scenarios where it's applied.
Understanding Point Estimates
A point estimate is a single value derived from sample data that serves as the best guess or prediction for a population parameter. In the context of the population mean, the point estimate is a single number that represents our best estimate of the true, but unknown, population mean. It's the "best guess" based on the information available in our sample.
Think of it like this: you want to know the average height of all students in a large university. It's impractical to measure every student. Instead, you take a sample of, say, 100 students, measure their heights, and calculate the average height of that sample. This sample average is your point estimate for the population mean height.
Calculating the Point Estimate for the Population Mean
The most common point estimate for the population mean (µ) is the sample mean (x̄). The sample mean is simply the average of the values in your sample. The formula for calculating the sample mean is:
x̄ = Σxᵢ / n
Where:
- x̄ is the sample mean (our point estimate)
- Σxᵢ is the sum of all values in the sample
- n is the sample size
This formula is straightforward to apply. You sum all the observed values in your sample and divide by the number of observations.
Example: Calculating the Sample Mean
Let's say we have a sample of five students' exam scores: 85, 92, 78, 88, and 95. To calculate the point estimate for the population mean exam score, we follow these steps:
- Sum the values: 85 + 92 + 78 + 88 + 95 = 438
- Divide by the sample size: 438 / 5 = 87.6
Therefore, our point estimate for the population mean exam score is 87.6.
Properties of a Good Point Estimator
While the sample mean is the most commonly used point estimate for the population mean, it's important to understand the properties that make a good estimator. A desirable point estimator should be:
-
Unbiased: An unbiased estimator's expected value is equal to the true population parameter. The sample mean is an unbiased estimator of the population mean. This means that if you were to repeatedly take samples and calculate the sample mean for each, the average of all those sample means would be very close to the true population mean.
-
Efficient: An efficient estimator has a smaller variance than other estimators. This means that the sample means from different samples are clustered more tightly around the true population mean, leading to a more precise estimate. The sample mean is generally considered to be an efficient estimator, particularly when the population is normally distributed.
-
Consistent: A consistent estimator's accuracy improves as the sample size increases. As you collect more data (increase your sample size), the sample mean gets closer and closer to the true population mean.
Limitations of Point Estimates
While point estimates provide a concise summary of the data, they have limitations:
-
Single Value: A point estimate is just a single number; it doesn't provide any information about the uncertainty or variability associated with the estimate. It doesn't tell us how close our estimate is likely to be to the true population mean.
-
Sampling Error: Point estimates are subject to sampling error. This means that the estimate obtained from a single sample may differ from the true population parameter due to random chance. Different samples will likely yield slightly different point estimates.
Addressing the Limitations: Confidence Intervals
To address the limitations of point estimates, statisticians often use confidence intervals. A confidence interval provides a range of values within which the true population parameter is likely to fall. It incorporates the uncertainty associated with the point estimate and provides a more complete picture.
A confidence interval is typically expressed as a percentage, such as a 95% confidence interval. A 95% confidence interval means that if we were to repeatedly take samples and calculate confidence intervals for each sample, 95% of those intervals would contain the true population mean.
Different Sampling Methods and Their Impact on Point Estimates
The method used to collect the sample can significantly impact the quality of the point estimate. Different sampling techniques have varying levels of bias and precision. Some common sampling methods include:
-
Simple Random Sampling: Every member of the population has an equal chance of being selected. This is often considered the gold standard and minimizes bias.
-
Stratified Sampling: The population is divided into strata (subgroups), and random samples are taken from each stratum. This is useful when there's substantial variability within the population.
-
Cluster Sampling: The population is divided into clusters, and a random sample of clusters is selected. All members of the selected clusters are then included in the sample. This is cost-effective when dealing with geographically dispersed populations.
-
Systematic Sampling: Members of the population are selected at regular intervals. For example, selecting every 10th person from a list.
Using an appropriate sampling method is critical for obtaining a reliable point estimate. Bias in the sampling method can lead to a point estimate that is far from the true population mean.
Point Estimates in Different Statistical Contexts
Point estimates are fundamental to various statistical analyses:
-
Hypothesis Testing: Point estimates are used to calculate test statistics, which are used to determine whether to reject a null hypothesis.
-
Regression Analysis: In regression analysis, the coefficients are point estimates of the relationship between variables.
-
Estimation of Proportions: The sample proportion is a point estimate for the population proportion.
-
Estimating Variance: The sample variance is used as a point estimate for the population variance.
Conclusion
The point estimate for the population mean, typically the sample mean, is a crucial concept in statistics. While it provides a concise summary of the data, it's important to understand its limitations, especially the lack of information about the uncertainty associated with the estimate. Combining point estimates with confidence intervals offers a more robust and informative approach to estimating population parameters. Choosing the appropriate sampling method is also critical for obtaining a reliable and unbiased point estimate. Remember that the accuracy of your point estimate is directly related to the quality and size of your sample. Larger, more representative samples generally lead to more accurate estimates. Always consider the context of your data and choose the statistical methods that best suit your needs.
Latest Posts
Latest Posts
-
How To Find Perimeter Of An Equilateral Triangle
Apr 16, 2025
-
Square Root Of 128 In Radical Form
Apr 16, 2025
-
How To Go From Alkane To Alkene
Apr 16, 2025
-
Matter Is Anything That Has And Occupies
Apr 16, 2025
-
The Members Of The Triple Alliance Were
Apr 16, 2025
Related Post
Thank you for visiting our website which covers about What Is The Point Estimate For The Population Mean . We hope the information provided has been useful to you. Feel free to contact us if you have any questions or need further assistance. See you next time and don't miss to bookmark.