The Random Variable X Is Normally Distributed
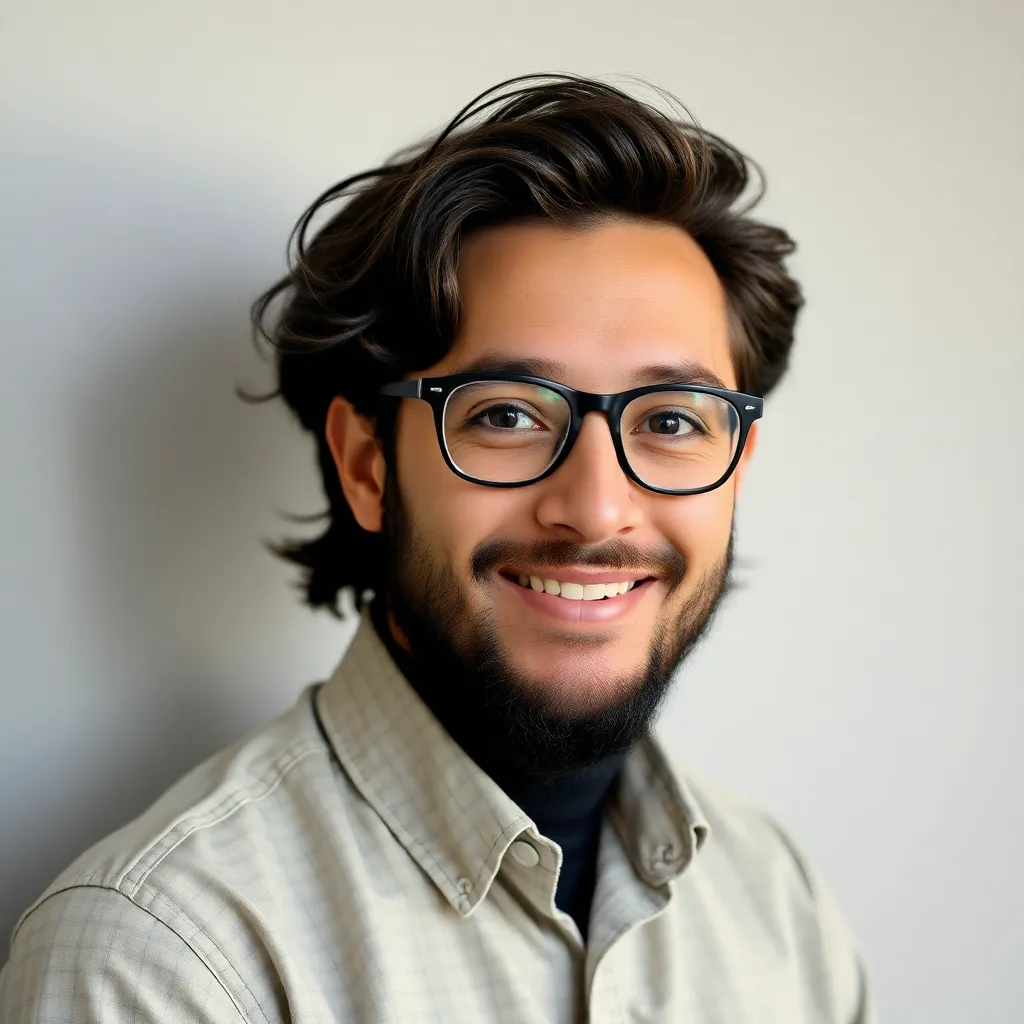
listenit
May 12, 2025 · 6 min read
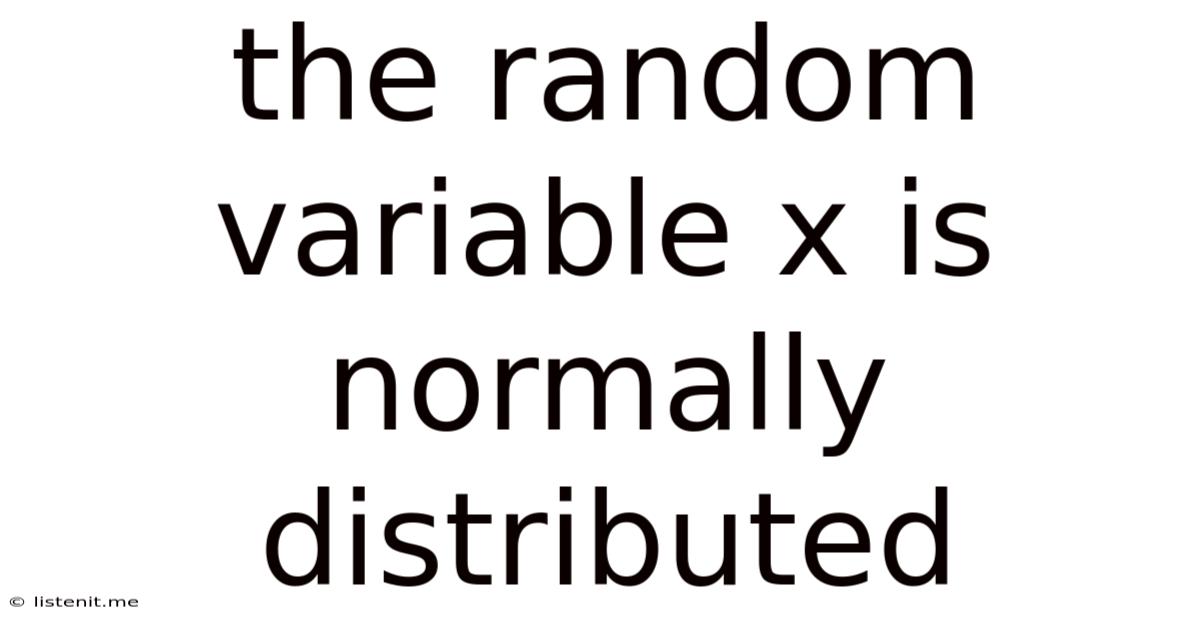
Table of Contents
The Random Variable X is Normally Distributed: A Comprehensive Guide
The normal distribution, often called the Gaussian distribution, is arguably the most important probability distribution in statistics. Its prevalence stems from the Central Limit Theorem, which states that the average of many independent and identically distributed random variables, regardless of their original distribution, will approximately follow a normal distribution. This makes it incredibly useful for modeling a vast range of phenomena in various fields, from finance and engineering to biology and social sciences. This article will delve into the characteristics, applications, and importance of the normal distribution when applied to a random variable X.
Understanding the Normal Distribution
A random variable X is said to be normally distributed if its probability density function (PDF) is given by:
f(x; μ, σ) = (1 / (σ√(2π))) * exp(-(x - μ)² / (2σ²))
where:
- μ (mu) represents the mean (average) of the distribution. This is also the location parameter, determining the center of the distribution.
- σ (sigma) represents the standard deviation, a measure of the spread or dispersion of the data. A larger σ indicates greater variability. σ² is the variance.
- exp denotes the exponential function (e^x).
- π is the mathematical constant pi (approximately 3.14159).
This seemingly complex formula describes a bell-shaped curve, symmetrical around its mean (μ). The area under this curve represents the probability of X falling within a given range. The total area under the curve is always equal to 1, reflecting the certainty that X will take on some value.
Key Properties of the Normal Distribution
Several crucial properties define the normal distribution:
- Symmetry: The distribution is perfectly symmetrical around its mean. The probability of observing a value a certain distance below the mean is identical to the probability of observing a value the same distance above the mean.
- Unimodality: It possesses only one mode (peak), which coincides with the mean and median.
- Mean, Median, and Mode: In a normal distribution, the mean, median, and mode are all equal.
- Empirical Rule (68-95-99.7 Rule): Approximately 68% of the data falls within one standard deviation of the mean (μ ± σ), 95% within two standard deviations (μ ± 2σ), and 99.7% within three standard deviations (μ ± 3σ). This rule provides a quick estimate of probabilities associated with different ranges.
- Infinite Range: The normal distribution theoretically extends from negative infinity to positive infinity, although the probability of observing extremely large or small values diminishes rapidly.
The Standard Normal Distribution (Z-Distribution)
A special case of the normal distribution is the standard normal distribution, also known as the Z-distribution. This distribution has a mean (μ) of 0 and a standard deviation (σ) of 1. Any normally distributed random variable X can be standardized by transforming it into a Z-score using the following formula:
Z = (X - μ) / σ
This Z-score represents the number of standard deviations X is away from the mean. The standard normal distribution simplifies calculations and allows us to use readily available Z-tables or statistical software to determine probabilities.
Applications of the Normal Distribution
The normal distribution's versatility makes it applicable across numerous fields:
1. Statistical Inference
- Hypothesis Testing: Many statistical tests assume that the data follows a normal distribution or a close approximation. Examples include t-tests, ANOVA, and regression analysis.
- Confidence Intervals: Constructing confidence intervals for population parameters often relies on the normal distribution, particularly when the sample size is large enough.
2. Quality Control
- Process Capability Analysis: The normal distribution is used to assess the capability of a manufacturing process to produce products within specified limits. Control charts based on the normal distribution help monitor process stability.
3. Finance
- Portfolio Management: The normal distribution is often (though sometimes controversially) used to model the returns of financial assets, aiding in portfolio optimization and risk management.
- Option Pricing: The Black-Scholes model, a cornerstone of option pricing, relies on the assumption of normally distributed asset returns.
4. Engineering
- Reliability Analysis: The normal distribution can model the lifetime of components or systems, enabling engineers to predict failure rates and design more reliable products.
- Signal Processing: Noise in many systems can be approximated by a normal distribution.
5. Natural Sciences
- Biological Data: Many biological measurements, such as height, weight, and blood pressure, approximately follow a normal distribution.
- Experimental Design: Normal distribution assumptions are often made in designing experiments and analyzing their results.
6. Social Sciences
- Psychological Testing: Scores on many psychological tests are designed to follow a normal distribution, allowing for standardized comparisons.
- Survey Data: Some survey responses can be modeled using the normal distribution, particularly when measuring continuous variables like attitudes or opinions.
Limitations of the Normal Distribution
While incredibly useful, it's crucial to acknowledge the limitations of applying the normal distribution:
- Real-world data is not always normally distributed: Many datasets exhibit skewness (asymmetry), kurtosis (heavy tails or peakedness), or other deviations from normality. Blindly assuming normality when it's not present can lead to inaccurate conclusions.
- Outliers: Extreme values (outliers) can heavily influence the mean and standard deviation, potentially distorting the distribution and the inferences drawn from it.
- Assumptions of independence: The central limit theorem, which underpins many applications of the normal distribution, requires the underlying random variables to be independent. If this assumption is violated, the results might be unreliable.
Assessing Normality
Several methods exist to determine whether a dataset reasonably approximates a normal distribution:
- Histograms and Q-Q plots: Visual inspection of histograms can provide a qualitative assessment of normality. Q-Q plots (quantile-quantile plots) compare the quantiles of the data to the quantiles of a normal distribution, providing a more formal visual check.
- Statistical Tests: Various statistical tests, such as the Shapiro-Wilk test, Kolmogorov-Smirnov test, and Anderson-Darling test, provide a quantitative assessment of normality. These tests produce p-values that help determine if the deviation from normality is statistically significant.
If the data significantly deviates from normality, transformations (like logarithmic or square root transformations) can sometimes be applied to improve the approximation, or alternative statistical methods that don't require normality assumptions might be necessary.
Conclusion: The Enduring Importance of the Normal Distribution
Despite its limitations, the normal distribution remains a fundamental tool in statistics and many scientific disciplines. Its mathematical elegance, combined with its frequent appearance in real-world data (either directly or through the Central Limit Theorem), ensures its continued relevance. Understanding its properties, applications, and limitations is essential for anyone working with data analysis and statistical inference. Remembering to assess the normality of your data and applying appropriate transformations or alternative methods when necessary is crucial for maintaining the validity and reliability of your statistical conclusions. By acknowledging both its power and its constraints, you can harness the utility of the normal distribution effectively and responsibly.
Latest Posts
Latest Posts
-
How Many Terms Are In The Expression
May 12, 2025
-
Find The Quotient And Remainder For 52 8
May 12, 2025
-
Greatest Common Factor Of 42 28 And 70
May 12, 2025
-
Molar Mass Of A Volatile Liquid
May 12, 2025
-
Lewis Dot Diagram For Ionic Compounds
May 12, 2025
Related Post
Thank you for visiting our website which covers about The Random Variable X Is Normally Distributed . We hope the information provided has been useful to you. Feel free to contact us if you have any questions or need further assistance. See you next time and don't miss to bookmark.