Pearson Correlation Between Weather Variables And Yield
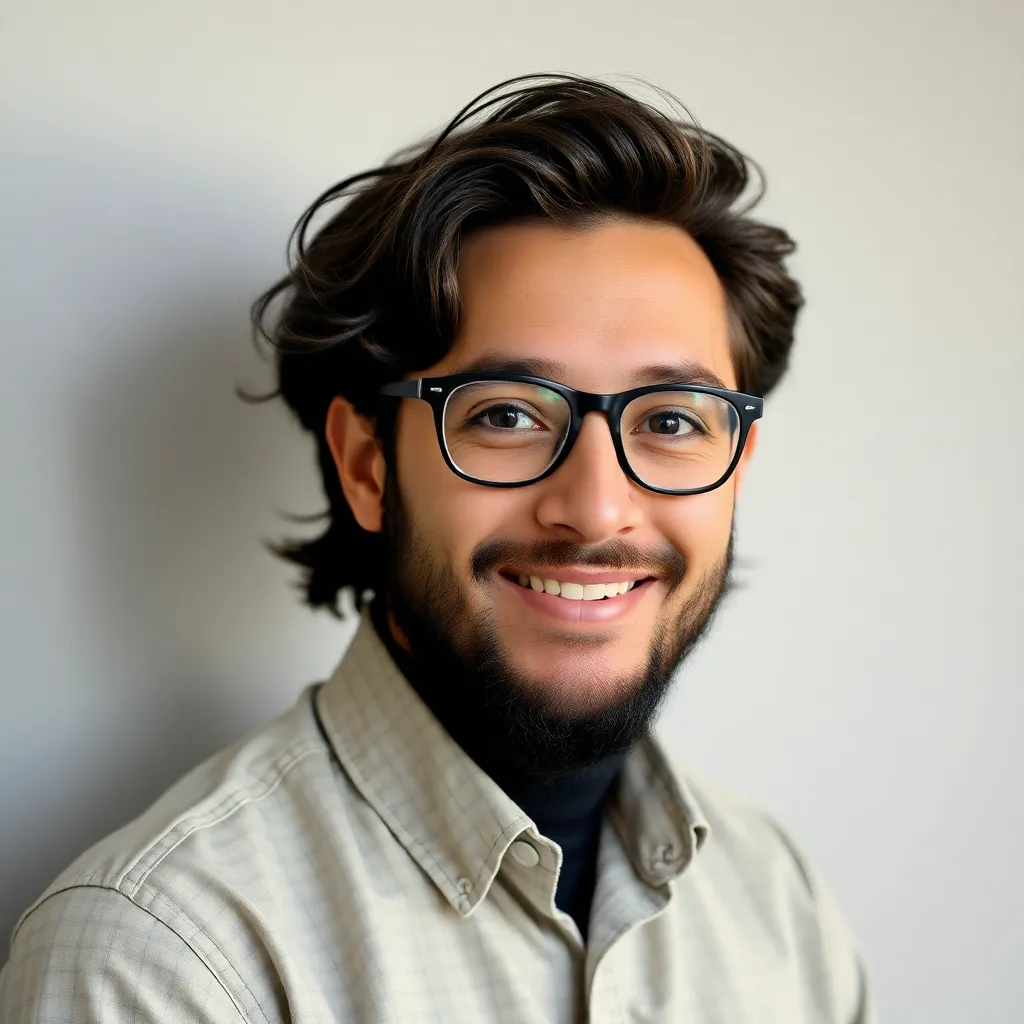
listenit
May 27, 2025 · 6 min read
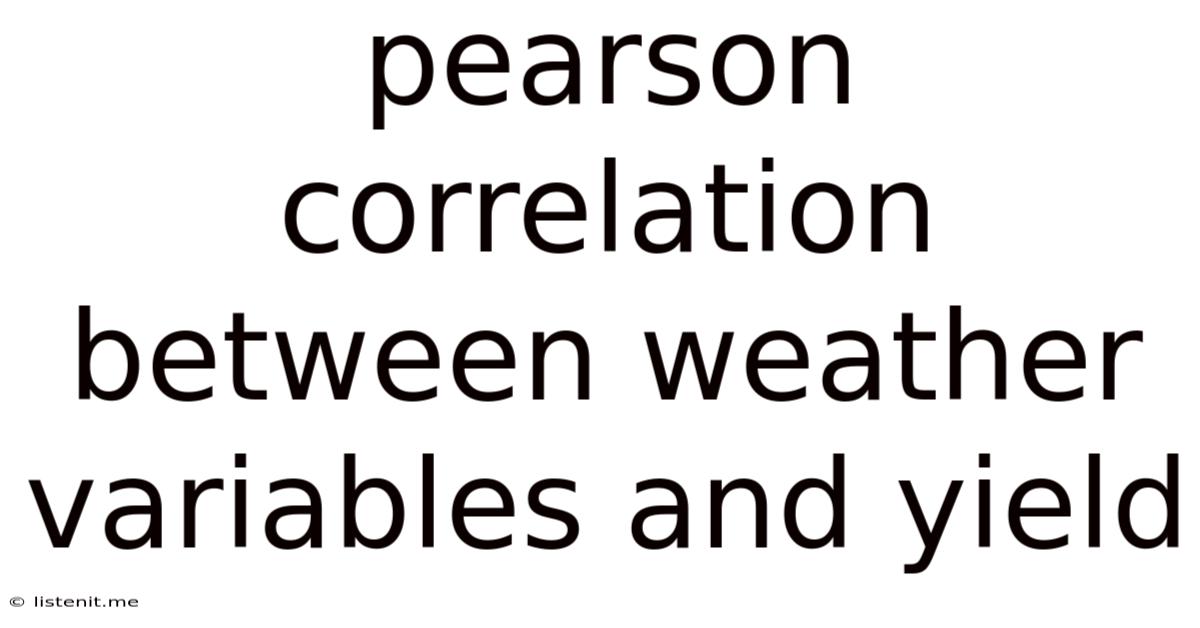
Table of Contents
Understanding the Pearson Correlation Between Weather Variables and Crop Yield
The relationship between weather and crop yield is a complex and crucial aspect of agriculture. Accurate prediction of yield is essential for efficient resource allocation, market forecasting, and ensuring food security. One powerful statistical tool used to analyze this relationship is the Pearson correlation coefficient. This article delves into the intricacies of using Pearson correlation to understand the association between various weather variables and crop yield, highlighting its strengths, limitations, and applications.
What is Pearson Correlation?
The Pearson correlation coefficient (often denoted as r) measures the linear association between two continuous variables. It ranges from -1 to +1, where:
- +1: Indicates a perfect positive linear correlation (as one variable increases, the other increases proportionally).
- 0: Indicates no linear correlation.
- -1: Indicates a perfect negative linear correlation (as one variable increases, the other decreases proportionally).
It's crucial to remember that correlation doesn't imply causation. A strong correlation simply suggests a relationship; it doesn't prove that one variable causes changes in the other. Other factors may be at play.
Weather Variables and Their Impact on Yield
Numerous weather variables significantly influence crop yield. These include:
1. Temperature:
Optimal Temperature Ranges: Each crop has an optimal temperature range for growth and development. Temperatures outside this range can lead to reduced yields. For example, excessively high temperatures can cause heat stress, leading to reduced photosynthesis, flower abortion, and grain filling issues. Conversely, excessively low temperatures can cause chilling injury, delaying growth and reducing overall productivity.
Correlation Analysis: Pearson correlation can be used to quantify the relationship between daily mean temperature (or specific temperature thresholds like maximum and minimum temperatures) and final yield. A positive correlation might be expected up to a certain temperature threshold, beyond which a negative correlation could be observed.
2. Rainfall:
Water Availability: Water is crucial for plant growth and development. Insufficient rainfall (drought) can severely stress crops, leading to reduced yields. Conversely, excessive rainfall (flooding) can damage crops, hinder nutrient uptake, and promote the spread of diseases.
Correlation Analysis: Correlation analysis can explore the relationship between total rainfall during the growing season or rainfall during critical growth stages (e.g., flowering, grain filling) and final yield. A positive correlation is generally expected within an optimal rainfall range, but excessive rainfall may lead to a negative correlation.
3. Solar Radiation (Sunlight):
Photosynthesis: Sunlight is the primary energy source for photosynthesis, the process by which plants convert light energy into chemical energy for growth. Insufficient sunlight can limit photosynthesis and reduce yield.
Correlation Analysis: Correlation analysis can investigate the relationship between total solar radiation during the growing season or daily sunlight hours and yield. A positive correlation is typically anticipated.
4. Humidity:
Disease and Pest Development: High humidity can favor the development of fungal diseases and pest infestations, negatively impacting yield.
Correlation Analysis: Correlation between humidity levels (relative humidity) and yield can be explored, likely showing a negative correlation when humidity consistently exceeds optimal ranges.
5. Wind Speed:
Physical Damage: Strong winds can cause physical damage to plants, leading to reduced yields. They can also increase transpiration rates, leading to water stress.
Correlation Analysis: Correlation analysis can assess the relationship between wind speed and yield, potentially revealing a negative correlation, particularly if high wind speeds are frequent.
Conducting Pearson Correlation Analysis for Crop Yield
Conducting a proper Pearson correlation analysis involves several key steps:
-
Data Collection: Gather accurate and reliable data on weather variables (temperature, rainfall, solar radiation, humidity, wind speed) and crop yield for the period under investigation. Data should be collected consistently, ideally at daily or weekly intervals throughout the growing season.
-
Data Preprocessing: Clean and preprocess the data to handle missing values and outliers. Missing values can be addressed using imputation techniques (e.g., mean imputation, regression imputation). Outliers can be identified and handled based on their potential impact on the analysis.
-
Correlation Calculation: Use statistical software (e.g., R, SPSS, Python with libraries like SciPy) to calculate the Pearson correlation coefficient (r) and its associated p-value for each weather variable against crop yield. The p-value indicates the statistical significance of the correlation. A p-value below a chosen significance level (commonly 0.05) suggests that the correlation is statistically significant.
-
Interpretation: Interpret the correlation coefficient and its p-value. A positive r indicates a positive linear relationship, while a negative r indicates a negative linear relationship. The magnitude of r indicates the strength of the relationship (closer to +1 or -1 indicates a stronger relationship). The p-value helps determine whether the observed correlation is likely due to chance or a real relationship.
-
Visualization: Create scatter plots to visualize the relationship between each weather variable and crop yield. This provides a visual representation of the correlation and helps identify any non-linear patterns or outliers.
Limitations of Pearson Correlation
While Pearson correlation is a valuable tool, it has limitations:
-
Linearity Assumption: Pearson correlation assumes a linear relationship between variables. If the relationship is non-linear, Pearson correlation may not accurately reflect the association.
-
Sensitivity to Outliers: Outliers can significantly influence the correlation coefficient.
-
Causation vs. Correlation: Correlation doesn't imply causation. Other factors can influence both weather variables and crop yield, leading to a correlation without a direct causal link.
-
Ignoring Temporal Dynamics: Traditional Pearson correlation doesn't explicitly account for the temporal dynamics of weather variables and their effects on yield throughout the growing season. More sophisticated time-series analyses may be needed.
Advanced Techniques and Considerations
To overcome some limitations of simple Pearson correlation, consider these advanced techniques:
-
Non-parametric correlations (e.g., Spearman's rank correlation): These methods are less sensitive to outliers and don't assume linearity.
-
Multiple regression analysis: This allows for assessing the combined effect of multiple weather variables on crop yield.
-
Time series analysis: This can capture the temporal dynamics of the relationship between weather and yield.
-
Spatial analysis: This considers the spatial variation in weather and yield, especially important in large-scale studies.
Applications and Conclusion
Understanding the correlation between weather variables and crop yield has significant practical applications:
-
Crop Yield Forecasting: Developing more accurate yield forecasts for better resource management and market planning.
-
Climate Change Impact Assessment: Assessing the potential impacts of climate change on crop yields and developing adaptation strategies.
-
Precision Agriculture: Optimizing irrigation, fertilization, and pest management practices based on weather conditions and their impact on yield.
-
Crop Insurance: Developing more effective crop insurance schemes that account for the variability in yield due to weather conditions.
In conclusion, Pearson correlation offers a valuable initial step in understanding the relationship between weather variables and crop yield. However, it's crucial to consider its limitations and employ more sophisticated techniques when necessary to gain a comprehensive and nuanced understanding of this complex interplay. Combining statistical analysis with agricultural knowledge and expert judgment is essential for accurate prediction and effective decision-making in agriculture. Further research incorporating diverse data sources and advanced analytical methods promises to enhance our ability to forecast and manage crop production in the face of climate variability and change.
Latest Posts
Latest Posts
-
How To Calculate Average Molar Mass
May 28, 2025
-
Collection Of Nerve Cell Bodies In The Pns
May 28, 2025
-
What Happens If A Needle Breaks Off In Your Arm
May 28, 2025
-
What Is Insitu And Exsitu Conservation
May 28, 2025
-
How To Enter Global Configuration Mode
May 28, 2025
Related Post
Thank you for visiting our website which covers about Pearson Correlation Between Weather Variables And Yield . We hope the information provided has been useful to you. Feel free to contact us if you have any questions or need further assistance. See you next time and don't miss to bookmark.