If The Median Is Greater Than The Mean
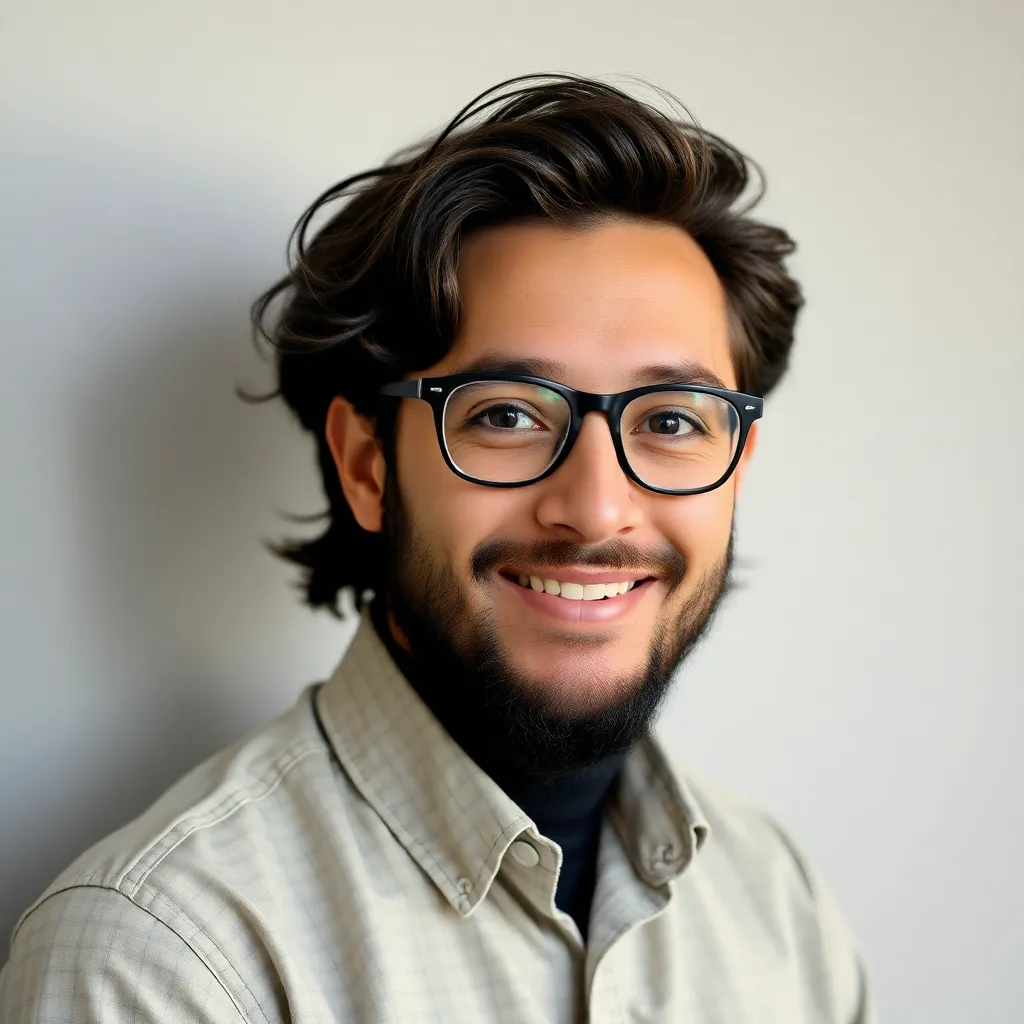
listenit
Mar 28, 2025 · 5 min read
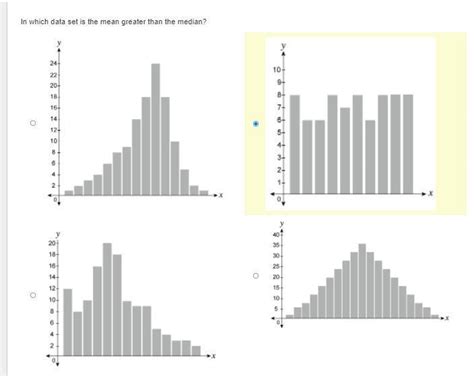
Table of Contents
If the Median is Greater Than the Mean: Understanding Skewed Distributions
The relationship between the mean and the median of a dataset provides valuable insights into its underlying distribution. While a symmetrical distribution will have a mean and median that are equal, an asymmetrical, or skewed, distribution will show a difference. This article delves into the implications when the median is greater than the mean, exploring the characteristics of such distributions, the reasons behind this disparity, and the practical applications of understanding this phenomenon.
Understanding Mean, Median, and Skewness
Before we dive into the specifics of a median greater than the mean, let's refresh our understanding of these fundamental statistical measures:
-
Mean: The average of a dataset, calculated by summing all values and dividing by the number of values. It's sensitive to outliers—extreme values that can significantly influence the mean.
-
Median: The middle value in a dataset when it's ordered from least to greatest. It's less sensitive to outliers than the mean.
-
Skewness: A measure of the asymmetry of a probability distribution. A distribution is skewed if one tail is longer than the other. We have:
- Positive Skew (Right Skew): The tail extends towards larger values. The mean is typically greater than the median.
- Negative Skew (Left Skew): The tail extends towards smaller values. The mean is typically less than the median.
When the Median is Greater Than the Mean: The Significance of Negative Skew
When the median exceeds the mean, it indicates a negative skew or left skew in the data distribution. This means that the distribution has a longer tail extending towards the lower values. The presence of a few extremely low values pulls the mean down, while the median, being less affected by these outliers, remains relatively higher.
Think of it visually: imagine a histogram representing the data. In a negatively skewed distribution, the majority of the data points cluster towards the higher end of the scale, with a few data points scattered far to the left, creating a long left tail. The median, representing the central point, sits above the mean, which is pulled down by these low-value outliers.
Visualizing Negative Skew
Imagine a dataset representing the income distribution of a small town. Most residents earn a moderate income, creating a peak in the distribution. However, a few residents have very low incomes (perhaps due to unemployment or underemployment). These low incomes create a long left tail, resulting in a negatively skewed distribution where the median income is higher than the average income.
Reasons for Negative Skewness and a Higher Median
Several factors can contribute to a negatively skewed distribution where the median surpasses the mean:
-
Presence of Outliers: As discussed, a few exceptionally low values significantly impact the mean, pulling it downwards. The median, less susceptible to these extremes, remains relatively unaffected.
-
Censored Data: In some scenarios, data collection methods might lead to censored data, where values below a certain threshold are not recorded. This can artificially inflate the median relative to the mean.
-
Natural Phenomena: Some natural phenomena inherently exhibit negative skewness. For instance, consider the distribution of rainfall in a region. Most years receive a relatively similar amount of rainfall, but occasional years with extremely low rainfall can create a long left tail, resulting in a higher median rainfall compared to the mean.
-
Specific Data Types: Certain data types, like reaction times in experiments, frequently display negative skewness. Most individuals react within a similar timeframe, but a few exceptionally slow reactions create a long left tail.
-
Distribution Limitations: Some distributions, like the log-normal distribution, are naturally negatively skewed, leading to a median greater than the mean under certain conditions.
Practical Applications and Interpretations
Understanding negative skewness and the relationship between the mean and median has practical applications across various fields:
-
Finance: In analyzing investment returns, a negatively skewed distribution might suggest potential risks associated with significant losses. The median return could be a better indicator of typical performance than the mean, which can be distorted by extreme negative returns.
-
Healthcare: Analyzing patient recovery times, the mean might be skewed by a few patients with exceptionally long recovery periods. The median recovery time would offer a more robust measure of typical recovery.
-
Environmental Science: In assessing water quality parameters, the presence of a few severely polluted samples could pull down the mean. The median would give a more reliable representation of typical water quality.
-
Social Sciences: Studying income inequality, negative skewness highlights the concentration of wealth at the higher end, with a few individuals or households having significantly lower incomes.
Distinguishing Negative Skew from Other Distributions
It's crucial to differentiate negatively skewed distributions from other types of distributions. A negatively skewed distribution should not be confused with:
-
Bimodal Distribution: A bimodal distribution has two peaks, indicating two distinct clusters of data. While it might show a difference between mean and median, the cause is different from negative skewness.
-
Uniform Distribution: A uniform distribution has a constant probability density across its range. The mean and median will be equal in this case.
Analyzing Data: Tools and Techniques
Several statistical tools and techniques can help identify and analyze negatively skewed distributions:
-
Histograms: Visual inspection of histograms can reveal the shape of the distribution and the presence of a long left tail.
-
Box Plots: Box plots display the median, quartiles, and outliers, providing a visual summary of the distribution's central tendency and spread.
-
Skewness Coefficient: This statistical measure quantifies the degree of skewness, with negative values indicating negative skew.
-
Statistical Software: Packages like R, Python (with libraries like NumPy and SciPy), and SPSS provide tools for calculating descriptive statistics, including the mean, median, and skewness coefficient.
Conclusion: The Importance of Context
When the median is greater than the mean, it's a strong indicator of negative skewness. This knowledge is invaluable for accurate interpretation of data across diverse fields. Understanding the underlying reasons for this disparity, whether due to outliers, data limitations, or inherent characteristics of the phenomenon under study, allows for a more nuanced and informed analysis. Remember to always consider the context of the data and choose appropriate statistical measures to avoid misleading conclusions. By employing appropriate visualization techniques and statistical analysis, one can effectively understand and communicate the implications of a negatively skewed distribution where the median surpasses the mean. This contributes to robust data interpretation and supports informed decision-making in various applications.
Latest Posts
Latest Posts
-
What Is 3 4 Equivalent To In Fractions
Mar 31, 2025
-
Where Is Most Freshwater Found On Earth
Mar 31, 2025
-
Simplify The Square Root Of 512
Mar 31, 2025
-
2 1 6 As An Improper Fraction
Mar 31, 2025
-
Explain One Major Difference Between Purines And Pyrimidines
Mar 31, 2025
Related Post
Thank you for visiting our website which covers about If The Median Is Greater Than The Mean . We hope the information provided has been useful to you. Feel free to contact us if you have any questions or need further assistance. See you next time and don't miss to bookmark.