How To Know If A Linear Model Is Appropriate
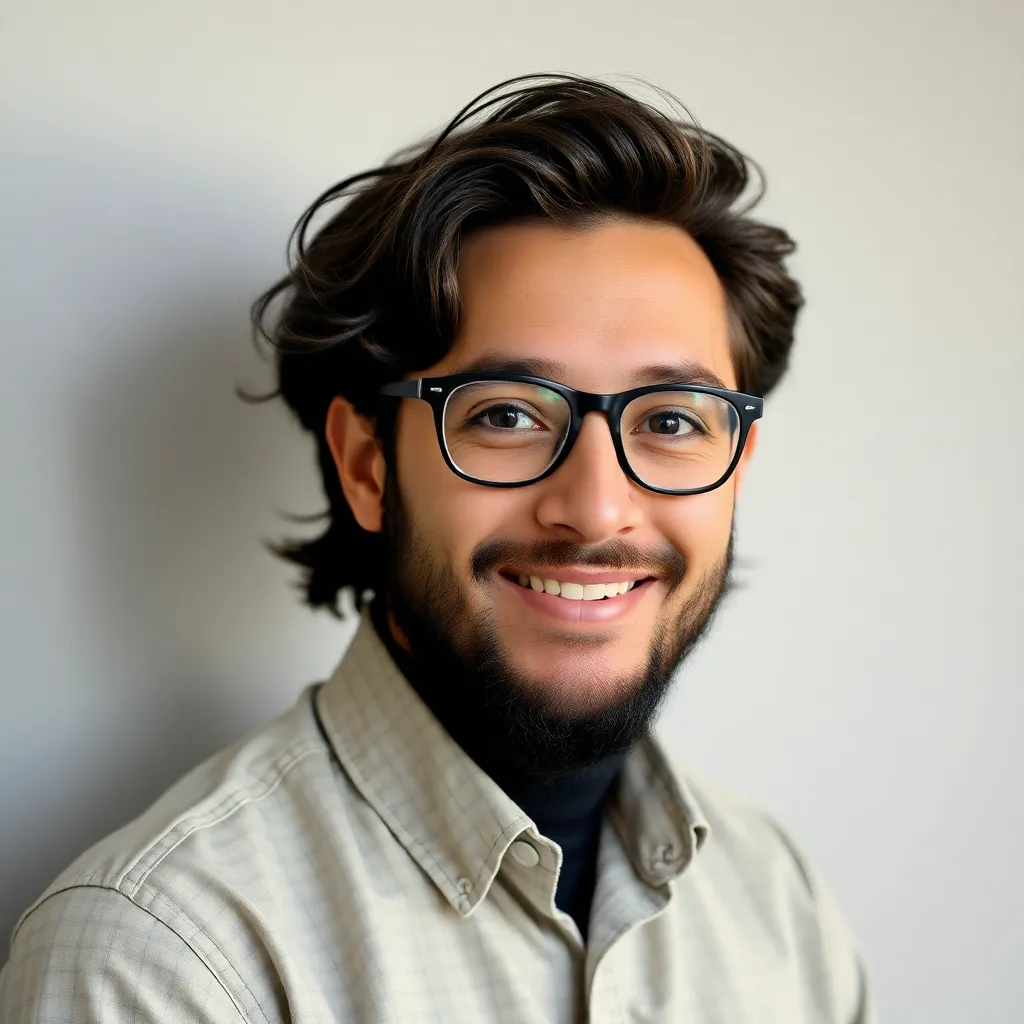
listenit
May 12, 2025 · 6 min read

Table of Contents
How to Know if a Linear Model is Appropriate: A Comprehensive Guide
Linear models are powerful tools in statistical analysis, offering a straightforward way to model the relationship between a dependent variable and one or more independent variables. However, their simplicity comes with a crucial caveat: they only work well when the underlying relationship is, in fact, linear. Applying a linear model to non-linear data can lead to inaccurate predictions, misleading interpretations, and flawed conclusions. This article provides a comprehensive guide on how to assess the appropriateness of a linear model before, during, and after its fitting.
Before Model Fitting: Exploring Your Data
Before even considering a linear model, thorough exploratory data analysis (EDA) is crucial. This stage helps you understand the nature of your data and identify potential red flags that suggest a linear model might be unsuitable.
1. Visual Inspection: Scatter Plots and Histograms
The most straightforward approach is to visually inspect your data.
-
Scatter Plots: For simple linear regression (one independent variable), create a scatter plot of your dependent variable against your independent variable. A clear linear trend suggests a linear model might be appropriate. However, non-linear patterns, such as curves or clusters, immediately raise concerns. For multiple linear regression (multiple independent variables), consider pairwise scatter plots or other visualization techniques to explore relationships between variables.
-
Histograms and Box Plots: Examine the distributions of both your dependent and independent variables. Extreme skewness or heavy-tailed distributions can affect the assumptions of a linear model and might require transformations (e.g., logarithmic, square root) before proceeding. Outliers also require careful consideration; they can disproportionately influence the model's parameters.
2. Correlation Analysis: Measuring Linear Association
Correlation coefficients (e.g., Pearson's r) quantify the linear association between variables. A strong correlation (close to +1 or -1) suggests a linear relationship, while a weak correlation (close to 0) indicates a weak or non-existent linear association. However, correlation does not imply causation, and a high correlation doesn't automatically guarantee the appropriateness of a linear model. Non-linear relationships can still show high correlations over specific ranges.
3. Domain Knowledge: Understanding the Variables
Your understanding of the underlying processes generating your data is invaluable. Does it make sense, theoretically, that the relationship between your variables should be linear? If, based on your knowledge, a non-linear relationship is expected, then forcing a linear model would be inappropriate.
During Model Fitting: Assessing Model Assumptions
Linear models rely on several key assumptions. Violations of these assumptions can invalidate the model's results. Therefore, it’s critical to assess these assumptions during and after model fitting.
1. Linearity: The Relationship Between Variables
The most fundamental assumption is that the relationship between the dependent and independent variables is linear. We already touched on this in EDA. During model fitting, you can further investigate linearity through:
-
Residual Plots: After fitting your model, create plots of the residuals (the differences between observed and predicted values) against each independent variable. Randomly scattered residuals around zero suggest linearity. Systematic patterns, like curves or cones, indicate non-linearity.
-
Partial Regression Plots (Added Variable Plots): These plots help visualize the relationship between the dependent variable and a specific independent variable, controlling for the effects of other independent variables. Non-linear patterns in these plots suggest the need for non-linear terms or transformations.
2. Independence of Errors: No Autocorrelation
The model assumes that the errors (residuals) are independent of each other. This is particularly important in time series data, where consecutive observations might be correlated. Autocorrelation can be detected through:
-
Durbin-Watson Test: This statistical test checks for autocorrelation in the residuals. A value close to 2 indicates no autocorrelation.
-
Correlogram (ACF/PACF Plots): These plots visualize the autocorrelation function (ACF) and partial autocorrelation function (PACF) of the residuals. Significant correlations at non-zero lags suggest autocorrelation.
3. Homoscedasticity: Constant Variance of Errors
Homoscedasticity implies that the variance of the errors is constant across all levels of the independent variables. Heteroscedasticity (non-constant variance) violates this assumption. It can be detected through:
-
Residual Plots: Examine the residual plots again. A funnel shape (variance increasing or decreasing with the independent variable) is a clear sign of heteroscedasticity.
-
Breusch-Pagan Test: This formal statistical test checks for heteroscedasticity.
4. Normality of Errors: Distribution of Residuals
Although not as crucial as the other assumptions, the assumption of normally distributed errors is important for inference (e.g., hypothesis testing and confidence intervals). You can check this through:
- Histograms and Q-Q Plots: Examine the distribution of residuals using histograms and quantile-quantile (Q-Q) plots. Deviations from normality can be addressed through transformations or using robust regression techniques.
After Model Fitting: Evaluating Model Performance
Even if the assumptions seem reasonably met, assessing the overall model performance is crucial to determine its appropriateness.
1. R-squared and Adjusted R-squared
-
R-squared: Represents the proportion of variance in the dependent variable explained by the model. While a high R-squared is desirable, it doesn't necessarily indicate a good model, especially with a large number of predictors.
-
Adjusted R-squared: Penalizes the inclusion of irrelevant predictors, providing a more realistic measure of the model's explanatory power.
2. Root Mean Squared Error (RMSE) and Mean Absolute Error (MAE)
These metrics quantify the average prediction error of the model. Lower values indicate better predictive accuracy.
3. Residual Analysis: Revisited
After fitting the model and evaluating the initial diagnostics, revisit residual analysis. Examine the distribution of residuals, looking for any unusual patterns or outliers. Consider investigating influential points (points that have a disproportionate effect on the model’s estimates).
Addressing Issues: Transformations and Alternatives
If your analysis reveals violations of the linear model assumptions, several strategies can be employed:
1. Data Transformations
Transforming your variables (e.g., logarithmic, square root, Box-Cox) can often address issues like non-linearity, heteroscedasticity, and non-normality. Experiment with different transformations to see what works best.
2. Non-linear Models
If the relationship between variables is inherently non-linear, consider using non-linear models such as polynomial regression, spline regression, or generalized additive models (GAMs).
3. Robust Regression
Robust regression techniques are less sensitive to outliers and violations of normality assumptions.
4. Feature Engineering
Creating new variables from existing ones (e.g., interaction terms, polynomial terms) can sometimes improve model fit and address non-linearity.
Conclusion
Determining whether a linear model is appropriate is a multifaceted process that requires careful consideration of several factors. By diligently performing EDA, assessing model assumptions, and evaluating model performance, you can make an informed decision about the suitability of a linear model for your data. Remember that no single test guarantees appropriateness; rather, a holistic evaluation of these various aspects is needed for a robust and accurate analysis. If violations are found and cannot be resolved by transformations or other remedies, adopting alternative modeling strategies becomes necessary. A thorough understanding of your data and the underlying processes is critical for selecting the most appropriate statistical model.
Latest Posts
Latest Posts
-
What Is The Net Charge Of A Nucleus
May 12, 2025
-
Do Quotes Go After The Period
May 12, 2025
-
Is 75 A Prime Or Composite Number
May 12, 2025
-
How Many Terms Are In The Expression
May 12, 2025
-
Find The Quotient And Remainder For 52 8
May 12, 2025
Related Post
Thank you for visiting our website which covers about How To Know If A Linear Model Is Appropriate . We hope the information provided has been useful to you. Feel free to contact us if you have any questions or need further assistance. See you next time and don't miss to bookmark.