How Does An Outlier Affect The Mean
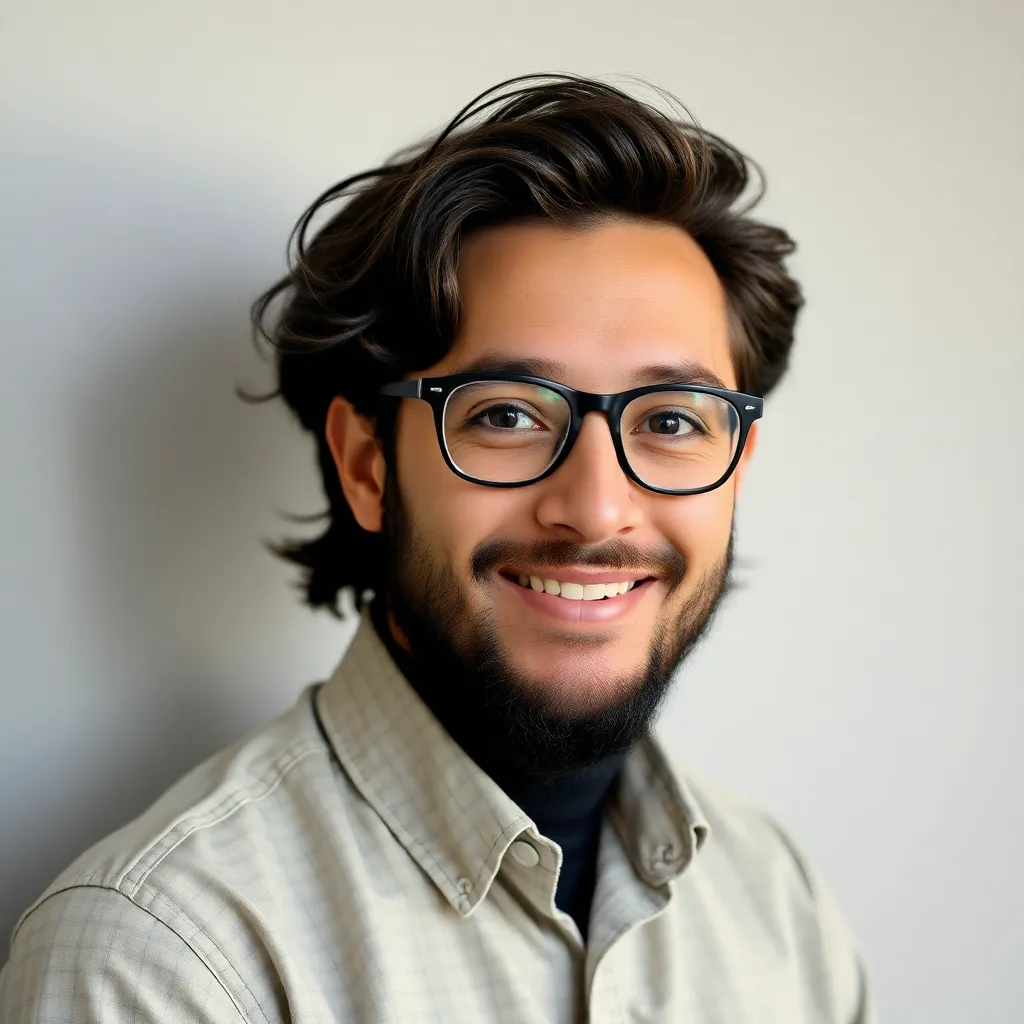
listenit
Apr 05, 2025 · 6 min read
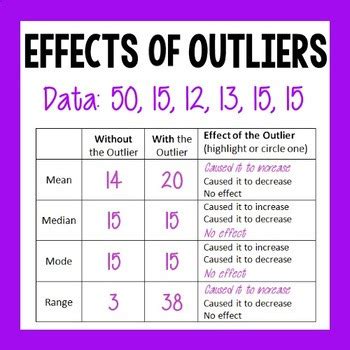
Table of Contents
How Does an Outlier Affect the Mean? Understanding the Impact of Extreme Values
Outliers. Those pesky data points that seem to defy the norm, residing far from the rest of the data set. While they might seem like anomalies to be ignored, understanding how outliers affect the mean is crucial for accurate data analysis and interpretation. This comprehensive guide delves into the impact of outliers on the mean, exploring various scenarios and providing practical strategies to handle them.
What is an Outlier?
Before we dive into the effects, let's clearly define what constitutes an outlier. An outlier is a data point that significantly deviates from the other observations in a dataset. This deviation is typically measured in terms of its distance from the mean or median. There's no single, universally accepted definition of an outlier; its identification often relies on context and the chosen statistical method. Common methods for detecting outliers include:
-
Visual inspection: Plotting your data (e.g., using histograms, box plots, scatter plots) can visually reveal data points that appear far removed from the central tendency.
-
Z-score: This method calculates how many standard deviations a data point lies from the mean. A commonly used threshold is ±3 standard deviations. Points beyond this threshold are often considered outliers.
-
Interquartile Range (IQR): This robust method uses the difference between the 75th and 25th percentiles (Q3 - Q1) to identify outliers. Data points falling below Q1 - 1.5 * IQR or above Q3 + 1.5 * IQR are typically flagged as outliers.
The Mean: A Sensitive Measure
The arithmetic mean (or simply the mean) is the average of a dataset. It's calculated by summing all the values and dividing by the number of values. While the mean is a widely used measure of central tendency, it possesses a critical vulnerability: it's highly sensitive to outliers.
This sensitivity arises because the mean considers every data point equally in its calculation. A single extreme value can disproportionately inflate or deflate the mean, making it a less representative measure of the central tendency when outliers are present.
How Outliers Inflate or Deflate the Mean
Let's illustrate this with an example. Consider the following dataset representing the daily income of a small business:
$100, $110, $120, $115, $105, $1,000,000
The mean income is approximately $143,014. However, this value is heavily skewed by the extreme value of $1,000,000. Without this outlier, the mean income would be approximately $110, a much more representative value of the typical daily income. This example clearly demonstrates how a single outlier can drastically inflate the mean, making it misleading as a measure of central tendency.
Conversely, a very low outlier can significantly deflate the mean. Imagine the same scenario but with a daily income of -$100,000 instead of $1,000,000. The mean would be dramatically lower than the actual typical income.
Understanding the Impact on Data Interpretation
The distortion caused by outliers can lead to several problems in data interpretation:
-
Misleading conclusions: A skewed mean can lead to inaccurate conclusions about the central tendency of the data. Decisions based on such a distorted mean could be flawed.
-
Poor model performance: In statistical modeling, outliers can significantly impact the model's accuracy and predictive power. Outliers can unduly influence the model's parameters, leading to poor fit and unreliable predictions.
-
Distorted visualizations: Graphical representations of data, such as histograms and box plots, can be affected by outliers, potentially obscuring the underlying distribution of the data.
Strategies for Handling Outliers
When dealing with outliers, you don't automatically discard them. Careful consideration and investigation are needed. Here's a breakdown of common strategies:
1. Investigate the Outlier
Before taking any action, thoroughly investigate the source of the outlier. Is it a data entry error? Is it a genuine observation representing a unique event or population? Understanding the origin of the outlier is critical in deciding how to handle it.
2. Winsorizing
This method replaces extreme values with less extreme ones. Instead of removing the outlier, it's replaced with a value closer to the rest of the data, such as the 95th or 5th percentile. This retains the information while mitigating the outlier's impact.
3. Trimming
This approach involves removing a fixed percentage of the most extreme values from each end of the data set. For example, you could remove the top and bottom 5% of the data. However, this method can lead to the loss of valuable information.
4. Using Robust Statistics
Robust statistics are less susceptible to the influence of outliers. Instead of the mean, consider using the median, which is the middle value when the data is sorted. The median is far less sensitive to extreme values. Similarly, the interquartile range (IQR) is a robust measure of variability.
5. Transforming the Data
Transforming the data (e.g., using logarithmic or square root transformations) can sometimes reduce the impact of outliers. This method compresses the range of values, making the outliers less influential. However, this should be done with careful consideration of the data's underlying distribution.
6. Separate Analysis
If you determine that the outlier represents a truly distinct population or event, you may consider analyzing the data with and without the outlier to compare the results and draw more nuanced conclusions. This approach helps to understand both the general trend and the impact of the unique event.
Choosing the Right Approach
The optimal strategy for dealing with outliers depends on several factors:
-
The nature of the data: Is the data normally distributed? Are there likely to be errors in data collection?
-
The goal of the analysis: What insights are you trying to extract from the data?
-
The size of the dataset: The impact of a single outlier is more significant in smaller datasets.
There is no one-size-fits-all solution. Careful consideration of these factors, combined with a sound understanding of the potential impacts of outliers, is essential for accurate and meaningful data analysis.
Conclusion: Outliers and the Pursuit of Accurate Insights
Outliers represent a common challenge in data analysis. Their presence can significantly affect the mean, leading to misleading interpretations and poor model performance. Therefore, understanding how outliers impact the mean is critical for accurate data analysis. Through careful investigation, application of appropriate statistical techniques, and judicious selection of data handling strategies, the impact of outliers can be effectively managed, ensuring that the analysis yields accurate and valuable insights. Remember, the key is not to simply remove outliers, but to understand their context and make informed decisions about their role in your analysis. This approach ensures the integrity and reliability of your findings and fosters a deeper understanding of the data you are working with.
Latest Posts
Latest Posts
-
Why Do Contour Lines Never Cross
Apr 05, 2025
-
Is Boron A Gas Solid Or Liquid
Apr 05, 2025
-
How Do You Factor 2x 2 7x 3
Apr 05, 2025
-
Which Group Of Metals Are The Most Reactive
Apr 05, 2025
-
How To Find The Perimeter Of A Right Angle Triangle
Apr 05, 2025
Related Post
Thank you for visiting our website which covers about How Does An Outlier Affect The Mean . We hope the information provided has been useful to you. Feel free to contact us if you have any questions or need further assistance. See you next time and don't miss to bookmark.