How Do Outliers Affect The Mean
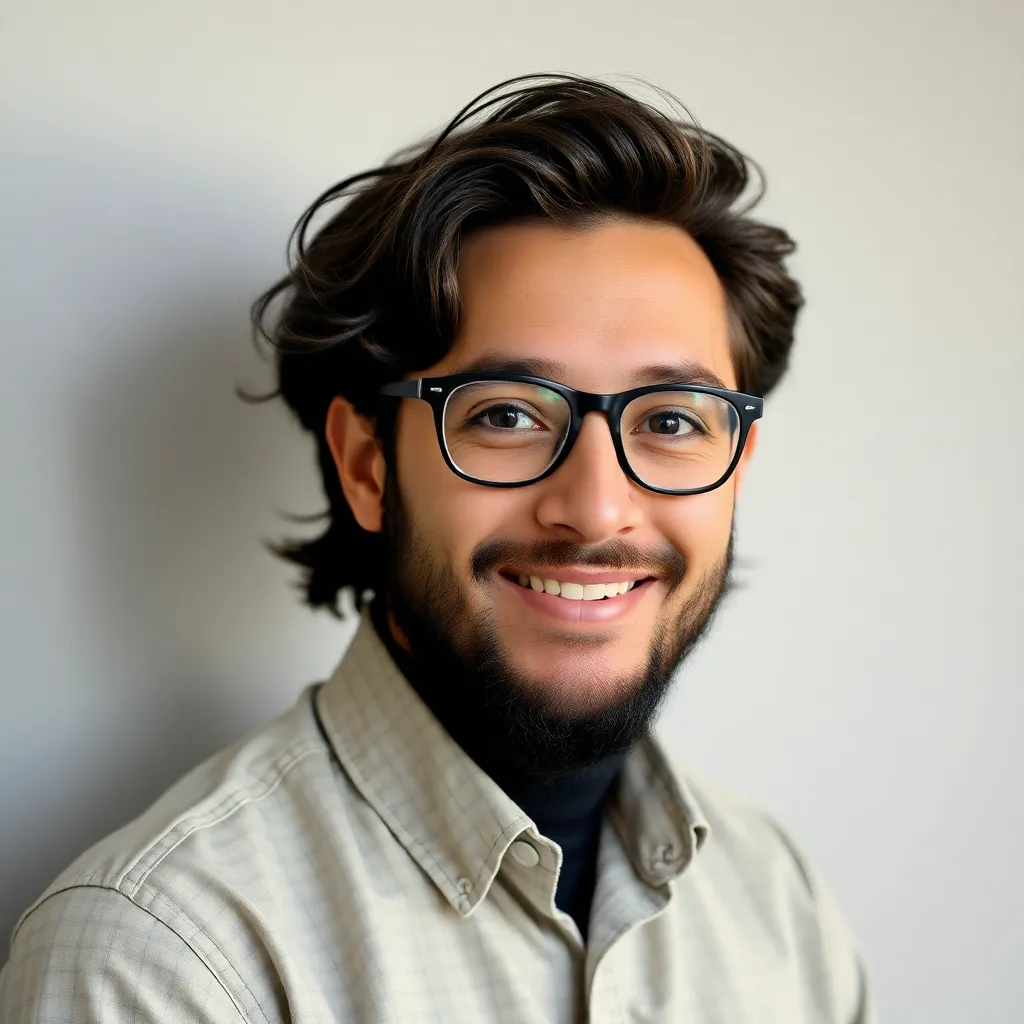
listenit
Apr 03, 2025 · 6 min read
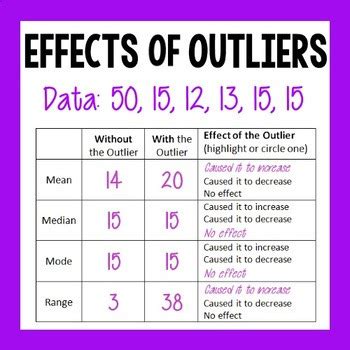
Table of Contents
How Do Outliers Affect the Mean? A Deep Dive into Statistical Influence
Outliers. Those pesky data points that seem to defy the norm, existing far from the rest of the data set. While often dismissed as anomalies or errors, outliers hold significant power to influence statistical analyses, especially when calculating the mean (average). Understanding how outliers affect the mean is crucial for accurate data interpretation and informed decision-making across various fields, from finance and healthcare to social sciences and engineering.
What are Outliers?
Before diving into their impact on the mean, let's clearly define outliers. An outlier is a data point that significantly differs from other observations in a dataset. This difference is typically measured in terms of its distance from the central tendency (mean, median, or mode) or its deviation from the overall pattern of the data. It's important to note that the definition of "significant" is context-dependent and often requires subjective judgment or the application of specific statistical methods.
There are two main types of outliers:
-
Univariate Outliers: These outliers deviate significantly from the central tendency of a single variable. For instance, in a dataset of salaries, a single extremely high salary would be a univariate outlier.
-
Multivariate Outliers: These outliers deviate significantly from the overall pattern of multiple variables. They might not be extreme in any single variable, but their combination of values makes them stand out from the rest of the data. Consider a dataset analyzing student performance; a student scoring low in all subjects could be a multivariate outlier.
Identifying outliers isn't always straightforward. While visual inspection using scatter plots, box plots, or histograms can provide initial insights, more sophisticated statistical methods are often necessary for reliable detection. These methods include the Z-score, modified Z-score, and Interquartile Range (IQR) methods.
The Mean: A Susceptible Statistic
The mean, or arithmetic average, is calculated by summing all data points and dividing by the number of data points. It's a widely used measure of central tendency, offering a simple and easily interpretable summary of the data. However, the mean's very nature makes it highly susceptible to the influence of outliers.
Why is the mean so sensitive to outliers?
The mean considers every data point in the calculation. A single extremely high or low outlier can drastically inflate or deflate the mean, pulling it away from a true representation of the central tendency for the majority of the data. This distortion can lead to misleading conclusions and inaccurate inferences.
Let's illustrate with a simple example:
Consider the following dataset representing the ages of individuals in a group: 25, 27, 28, 29, 30, 32, 35, 100.
The mean age is (25 + 27 + 28 + 29 + 30 + 32 + 35 + 100) / 8 = 39.5.
However, removing the outlier (100), the mean becomes (25 + 27 + 28 + 29 + 30 + 32 + 35) / 7 = 29.4. Notice the significant difference – a single outlier dramatically increased the mean.
Consequences of Outlier Influence on the Mean
The impact of outliers on the mean extends beyond simple misrepresentation of central tendency. It can lead to several critical consequences:
1. Biased Estimates:
Outliers can create biased estimates of the population mean. If the sample containing outliers is used to estimate the population mean, the resulting estimate will be inaccurate and misleading. This bias can affect downstream analyses and decision-making processes.
2. Misleading Statistical Tests:
Outliers can significantly influence the results of statistical tests, leading to incorrect conclusions. For instance, outliers can inflate the variance of a dataset, thereby reducing the power of statistical tests like t-tests or ANOVA. This can lead to a failure to reject a false null hypothesis (Type II error) or a false rejection of a true null hypothesis (Type I error).
3. Inaccurate Predictions:
In predictive modeling, outliers can significantly impair the accuracy of predictive models. Models trained on data with outliers may learn to overemphasize the outlier's influence, leading to poor predictions on new, unseen data. This is particularly problematic in applications like forecasting, risk assessment, and machine learning.
4. Distorted Visualizations:
Outliers can distort the visual representation of data, making it difficult to identify trends and patterns. Histograms and scatter plots can be skewed by outliers, obscuring the true distribution of the data and hindering visual interpretation.
5. Unreliable Confidence Intervals:
Outliers can lead to wider confidence intervals, indicating greater uncertainty in the estimated mean. This reduced precision can hamper the reliability of conclusions drawn from statistical analyses.
Mitigating the Effects of Outliers on the Mean
While outliers cannot always be ignored, it's crucial to understand their impact and consider appropriate strategies for mitigation. These strategies include:
1. Identification and Investigation:
The first and most important step is identifying potential outliers through visual inspection and statistical methods like Z-scores or IQR. Once identified, investigate the source of the outlier. Is it a genuine observation or a result of data entry error, measurement error, or other anomalies? If it's an error, correct it. If it's a genuine observation, carefully consider its impact and implications.
2. Transformation:
Data transformations, such as logarithmic or square root transformations, can reduce the influence of outliers by compressing the range of the data. This approach is particularly effective when outliers are skewed toward the higher end of the distribution.
3. Robust Statistics:
Use robust statistical methods that are less sensitive to outliers. The median, for instance, is a more robust measure of central tendency than the mean, as it is unaffected by extreme values. Other robust methods include trimmed means and Winsorized means, which involve removing or modifying the most extreme values before calculating the average.
4. Non-parametric Methods:
Consider using non-parametric statistical tests, which do not assume a specific data distribution. These methods are less sensitive to deviations from normality, including the presence of outliers. Examples include the Mann-Whitney U test and the Kruskal-Wallis test.
5. Winsorizing:
Winsorizing replaces extreme values with less extreme values—often the values at a certain percentile. For instance, you might replace the highest 5% of data points with the value at the 95th percentile. This reduces the influence of extreme values without completely removing them from the dataset.
6. Data Splitting/Subsetting:
If the source of the outliers is identifiable (e.g., a specific group or condition), consider splitting or subsetting your data to analyze different groups separately. This can allow you to investigate potential differences between groups and avoid having outliers from one group unduly influence the analysis of other groups.
7. Reporting and Transparency:
Always report the presence of outliers and how they were handled in your analysis. Transparency is crucial to allow readers to evaluate the potential impact of outliers on your conclusions.
Conclusion: Outliers – A Challenge and an Opportunity
Outliers represent a challenge in statistical analysis, but understanding their nature and effects allows you to address them effectively. While they can distort the mean and other statistics, appropriate handling techniques help in obtaining accurate results. By carefully investigating the source of outliers, employing robust statistical methods, and reporting your procedures clearly, you can ensure that your analyses are reliable and your conclusions are informed. Remember, outliers are not always simply errors; sometimes, they hold important clues about unexpected patterns and trends in your data, offering valuable insights that would otherwise be missed. Thus, careful consideration of outliers is essential for robust statistical analysis and reliable decision making.
Latest Posts
Latest Posts
-
What Is The Atomic Number Of Ne
Apr 03, 2025
-
4 Light Years In Earth Years
Apr 03, 2025
-
27 To The Power Of 1 3
Apr 03, 2025
-
What Is The Greatest Common Factor Of 54 And 45
Apr 03, 2025
-
Finding The Gradient Of A Function
Apr 03, 2025
Related Post
Thank you for visiting our website which covers about How Do Outliers Affect The Mean . We hope the information provided has been useful to you. Feel free to contact us if you have any questions or need further assistance. See you next time and don't miss to bookmark.