Given The Discrete Probability Distribution Above Determine The Following
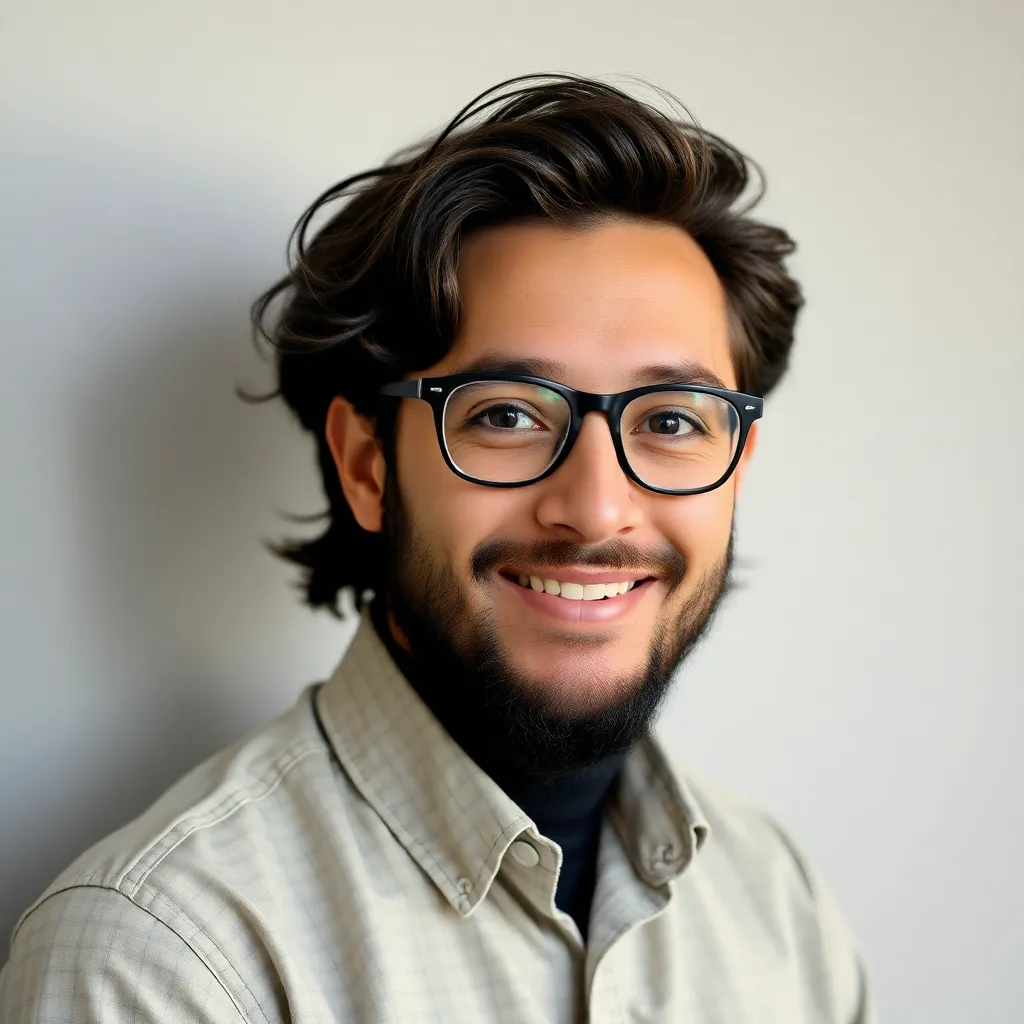
listenit
May 12, 2025 · 5 min read
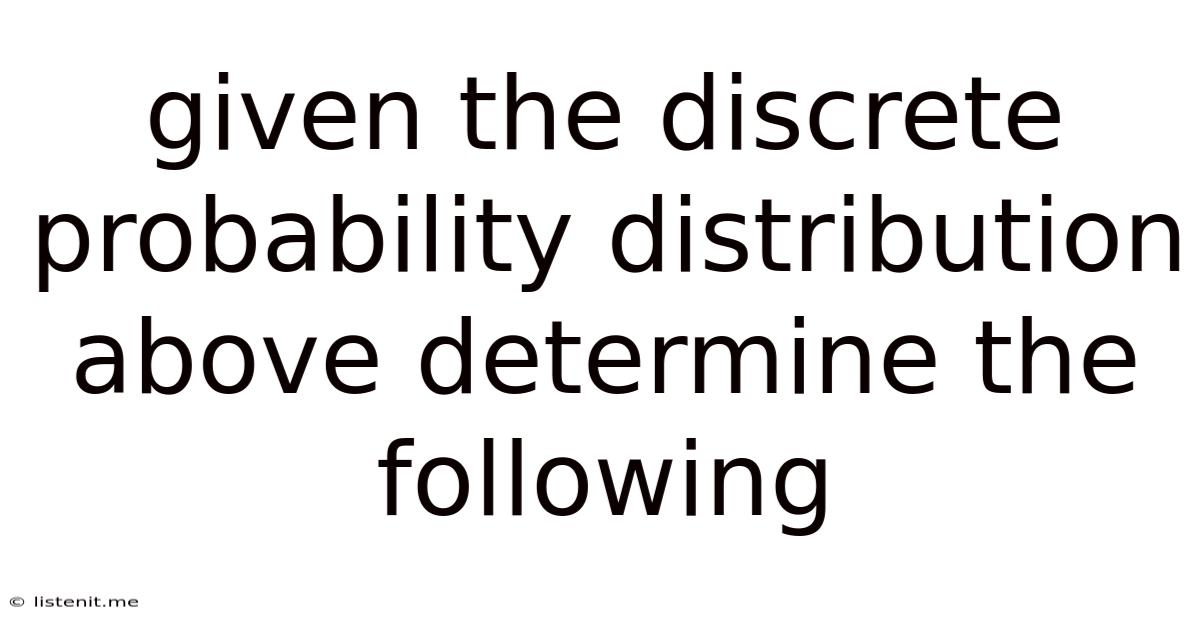
Table of Contents
Given the Discrete Probability Distribution Above, Determine the Following: A Comprehensive Guide
Determining key characteristics from a discrete probability distribution is a fundamental concept in statistics. This guide provides a comprehensive walkthrough, explaining the process step-by-step and offering practical examples. We'll cover how to calculate expected value, variance, standard deviation, and more, equipping you with the knowledge to confidently tackle similar problems. This guide also incorporates SEO best practices, using relevant keywords and semantic variations to ensure better search engine visibility.
Understanding Discrete Probability Distributions
Before delving into calculations, let's solidify our understanding of discrete probability distributions. A discrete probability distribution describes the probability of occurrence of each value of a discrete random variable. "Discrete" means the variable can only take on specific, separate values (like the number of heads in three coin tosses: 0, 1, 2, or 3). It's contrasted with a continuous probability distribution, where the variable can take on any value within a range (like the height of a student).
A discrete probability distribution is often represented in a table, listing each possible value of the random variable (X) and its corresponding probability, P(X). The sum of all probabilities in a valid probability distribution must always equal 1.
Key Characteristics of a Discrete Probability Distribution
Several key characteristics help us understand the behavior and properties of a discrete probability distribution. These include:
1. Expected Value (E(X) or μ)
The expected value, also known as the mean, represents the average value we expect the random variable to take on over many trials. It's calculated by summing the product of each value and its corresponding probability:
Formula: E(X) = Σ [x * P(X = x)]
where:
- x represents each value of the random variable.
- P(X = x) is the probability of the random variable taking on the value x.
- Σ denotes summation across all possible values of x.
2. Variance (Var(X) or σ²)
The variance measures the spread or dispersion of the data around the expected value. A higher variance indicates greater variability. It's calculated as the expected value of the squared differences between each value and the expected value:
Formula: Var(X) = E[(X - μ)²] = Σ [(x - μ)² * P(X = x)]
3. Standard Deviation (SD(X) or σ)
The standard deviation is the square root of the variance. It's expressed in the same units as the random variable, making it easier to interpret than the variance. It provides a more readily understandable measure of the spread of the data.
Formula: SD(X) = √Var(X) = √Σ [(x - μ)² * P(X = x)]
4. Higher Moments: Skewness and Kurtosis
While expected value, variance, and standard deviation are the most commonly used characteristics, higher moments like skewness and kurtosis provide further insights into the distribution's shape. Skewness measures the asymmetry of the distribution, while kurtosis describes the "tailedness" or the concentration of data in the tails of the distribution. These are more advanced statistical concepts and are often not necessary for basic understanding of a distribution.
Example Calculation: A Step-by-Step Guide
Let's illustrate these calculations with an example. Consider the following discrete probability distribution:
X | P(X) |
---|---|
1 | 0.2 |
2 | 0.3 |
3 | 0.4 |
4 | 0.1 |
1. Calculate the Expected Value (E(X)):
E(X) = (1 * 0.2) + (2 * 0.3) + (3 * 0.4) + (4 * 0.1) = 0.2 + 0.6 + 1.2 + 0.4 = 2.4
Therefore, the expected value is 2.4.
2. Calculate the Variance (Var(X)):
First, we need to calculate (x - μ)² for each value:
- (1 - 2.4)² = 1.96
- (2 - 2.4)² = 0.16
- (3 - 2.4)² = 0.36
- (4 - 2.4)² = 2.56
Now, we calculate the variance:
Var(X) = (1.96 * 0.2) + (0.16 * 0.3) + (0.36 * 0.4) + (2.56 * 0.1) = 0.392 + 0.048 + 0.144 + 0.256 = 0.84
Therefore, the variance is 0.84.
3. Calculate the Standard Deviation (SD(X)):
SD(X) = √Var(X) = √0.84 ≈ 0.92
Therefore, the standard deviation is approximately 0.92.
Interpreting the Results
In our example, the expected value of 2.4 suggests that if we were to repeat this experiment many times, the average value of X would be around 2.4. The variance of 0.84 and the standard deviation of 0.92 indicate the degree of variability in the data. A smaller standard deviation would imply that the data points are clustered closer to the mean, while a larger standard deviation suggests more spread out data.
Advanced Applications and Further Exploration
The concepts of expected value, variance, and standard deviation are crucial in various statistical applications, including:
-
Risk Assessment: In finance, expected value helps assess the potential return of an investment, while the standard deviation measures its risk. A higher standard deviation indicates higher volatility and risk.
-
Quality Control: In manufacturing, these metrics are used to monitor the consistency of products. A low standard deviation implies that products are consistently meeting quality standards.
-
Predictive Modeling: In machine learning, these statistical measures are fundamental for evaluating the performance of predictive models.
-
Decision Making under Uncertainty: In various fields, understanding the probability distribution of potential outcomes allows for better decision-making.
Conclusion
Mastering the analysis of discrete probability distributions is essential for anyone working with statistical data. This guide has provided a comprehensive overview of how to calculate and interpret key characteristics like expected value, variance, and standard deviation. By understanding these concepts, you can gain valuable insights from your data and make more informed decisions. Remember to always consider the context of your data and choose the appropriate statistical measures to answer your specific research questions. Continuous practice and exploration of different datasets will solidify your understanding and ability to apply these concepts effectively. This in-depth guide will enable you to confidently tackle various statistical problems and build a strong foundation in probability theory.
Latest Posts
Latest Posts
-
Distance From Jupiter To The Sun In Au
May 12, 2025
-
3x 4y 12 Slope Intercept Form
May 12, 2025
-
How Many Electrons Does Chlorine Have In Its Outer Shell
May 12, 2025
-
Which Gas Has The Slowest Rate Of Diffusion
May 12, 2025
-
How To Do Limits On Ti 84 Plus
May 12, 2025
Related Post
Thank you for visiting our website which covers about Given The Discrete Probability Distribution Above Determine The Following . We hope the information provided has been useful to you. Feel free to contact us if you have any questions or need further assistance. See you next time and don't miss to bookmark.