What Is The Best Point Estimate Of The Population Mean
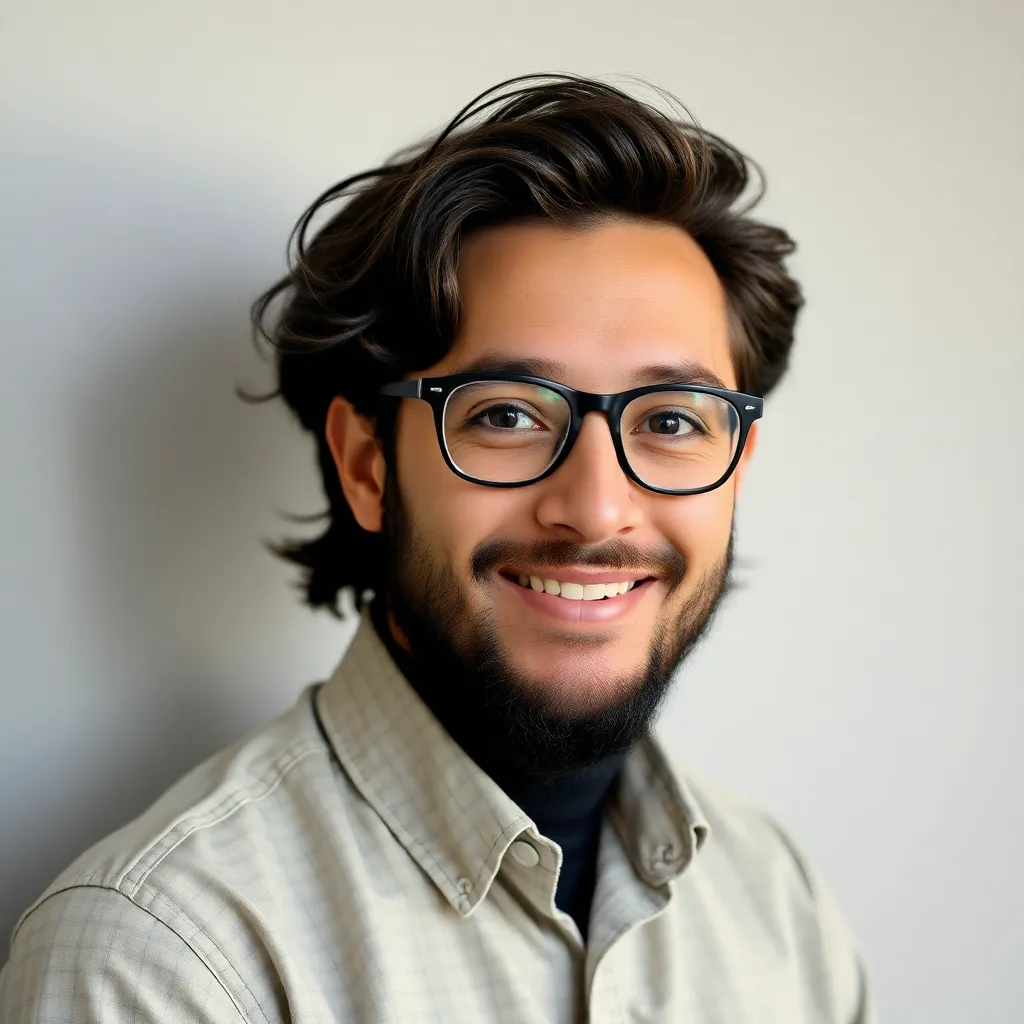
listenit
May 09, 2025 · 5 min read
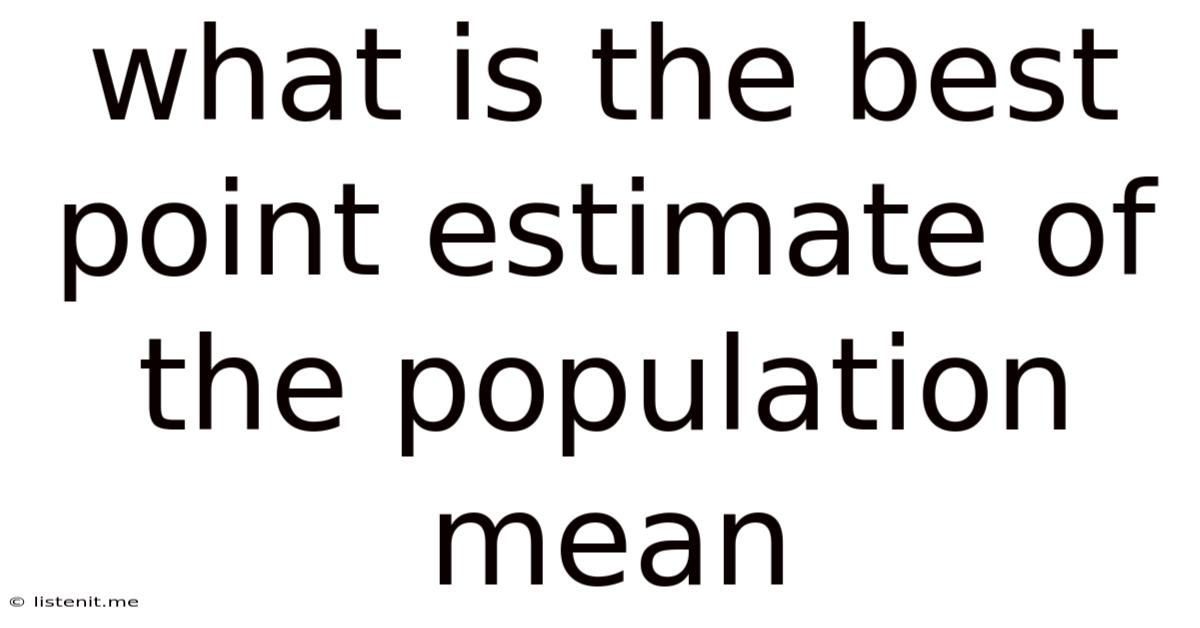
Table of Contents
What is the Best Point Estimate of the Population Mean?
Choosing the best point estimate for a population mean is a crucial aspect of statistical inference. The goal is to use a sample statistic to provide the most accurate and reliable single value representing the unknown population mean. While several estimators exist, the sample mean consistently emerges as the best option due to its desirable statistical properties. This article delves deep into why the sample mean reigns supreme, examining its strengths, comparing it to other estimators, and addressing potential limitations.
Understanding Point Estimation
Before diving into the specifics, let's clarify what point estimation entails. Point estimation involves using a single value calculated from sample data to estimate an unknown population parameter. In our case, the parameter of interest is the population mean (μ). The chosen value from the sample is called the point estimate. The quality of a point estimate depends on its accuracy and precision. Ideally, we want an estimate that is both unbiased (centered around the true value) and efficient (having the smallest variance among all unbiased estimators).
The Sample Mean: A Superior Estimator
The sample mean (denoted as x̄, pronounced "x-bar"), calculated by summing all sample observations and dividing by the number of observations (n), is the most commonly used point estimate for the population mean. Its dominance stems from several key properties:
1. Unbiasedness
An estimator is unbiased if its expected value is equal to the true population parameter. Mathematically, for an unbiased estimator θ̂ of parameter θ, E(θ̂) = θ. The sample mean satisfies this condition. The expected value of the sample mean is equal to the population mean: E(x̄) = μ. This means that, on average, the sample mean will accurately reflect the population mean. Repeated sampling will yield sample means that, on average, converge to the true population mean.
2. Minimum Variance (Efficiency)
Among all unbiased estimators, the sample mean possesses the minimum variance. Variance measures the spread or dispersion of the estimator's sampling distribution. A lower variance indicates that the sample means from repeated sampling will cluster more tightly around the true population mean, resulting in more precise estimation. This property is particularly crucial when dealing with smaller sample sizes where the variability of the sample mean is more pronounced. The Central Limit Theorem further supports this, stating that the sampling distribution of the sample mean approaches a normal distribution as the sample size increases, regardless of the population's distribution. This convergence enhances the precision of the sample mean as an estimator.
3. Consistency
As the sample size (n) increases, the sample mean converges in probability to the true population mean. This means that the probability of the sample mean being arbitrarily close to the population mean approaches 1 as the sample size grows. This consistency property assures us that with sufficiently large sample sizes, we can achieve a highly accurate estimate of the population mean.
Comparing the Sample Mean to Other Estimators
While the sample mean is the preferred estimator, it's important to acknowledge other potential estimators and why they are less desirable:
1. Sample Median:
The sample median is the middle value when the data is ordered. While robust to outliers, it's less efficient than the sample mean. Its variance is generally higher than the sample mean's variance, meaning it provides a less precise estimate, especially with normally distributed data. For data with a symmetric distribution, the median and mean will be approximately equal, but the sample mean will still offer better precision.
2. Sample Mode:
The sample mode is the most frequent value in the data. It's highly sensitive to outliers and only useful for describing the most common observation, not representing the central tendency effectively. It's generally not recommended as a point estimate for the population mean.
3. Trimmed Mean:
A trimmed mean is calculated by removing a certain percentage of the highest and lowest values before computing the average. This method is robust to outliers, but it sacrifices some information. The efficiency of a trimmed mean is less than that of the sample mean for normally distributed data, making the sample mean still the preferred choice in most scenarios.
Considerations and Limitations
While the sample mean is the best point estimate under ideal conditions, certain scenarios warrant careful consideration:
1. Non-Normal Distributions:
The efficiency of the sample mean is most pronounced when the population data is normally distributed. With highly skewed or non-normal distributions, the sample mean may be less efficient than other robust estimators, such as the trimmed mean or median. However, even with non-normal distributions, the Central Limit Theorem ensures the sample mean's distribution approaches normality with increasing sample size, mitigating this limitation to a considerable extent.
2. Outliers:
Outliers disproportionately affect the sample mean, potentially pulling the estimate away from the true population mean. If outliers are present and suspected to be erroneous, robust estimators like the median or trimmed mean might be more appropriate. However, careful investigation should always be conducted to determine the validity of outliers before removing or altering data.
3. Sample Size:
The accuracy and precision of the sample mean improve with increasing sample size. Small sample sizes can lead to larger sampling error and less reliable estimates. Therefore, striving for larger, representative samples is crucial for reliable point estimation.
Conclusion: The Sample Mean's Undisputed Reign
In conclusion, despite some limitations, the sample mean stands as the best point estimate for the population mean under most circumstances. Its unbiasedness, minimum variance (efficiency), and consistency make it a superior estimator. While alternative estimators exist and may be preferable under specific conditions like the presence of outliers or non-normal distributions, the sample mean's overall statistical properties solidify its position as the default and most reliable choice for estimating the population mean. Understanding its strengths and limitations allows for informed decision-making in selecting the most appropriate point estimate for a given situation. Remember, always carefully consider the nature of your data and the context of your analysis when choosing a point estimate. The sample mean, while optimal in many cases, is not a one-size-fits-all solution. Combining it with robust statistical practices and careful data exploration ensures more reliable and meaningful insights.
Latest Posts
Latest Posts
-
Is 56 Cm Greater Than 6m
May 09, 2025
-
Plot X As A Function Of Y
May 09, 2025
-
What Is The Lcm Of 10 And 18
May 09, 2025
-
Are The Most Numerous Type Of Neuron In The Cns
May 09, 2025
-
How Many Valence Electrons Are In He
May 09, 2025
Related Post
Thank you for visiting our website which covers about What Is The Best Point Estimate Of The Population Mean . We hope the information provided has been useful to you. Feel free to contact us if you have any questions or need further assistance. See you next time and don't miss to bookmark.