What Is A Factor In An Experiment
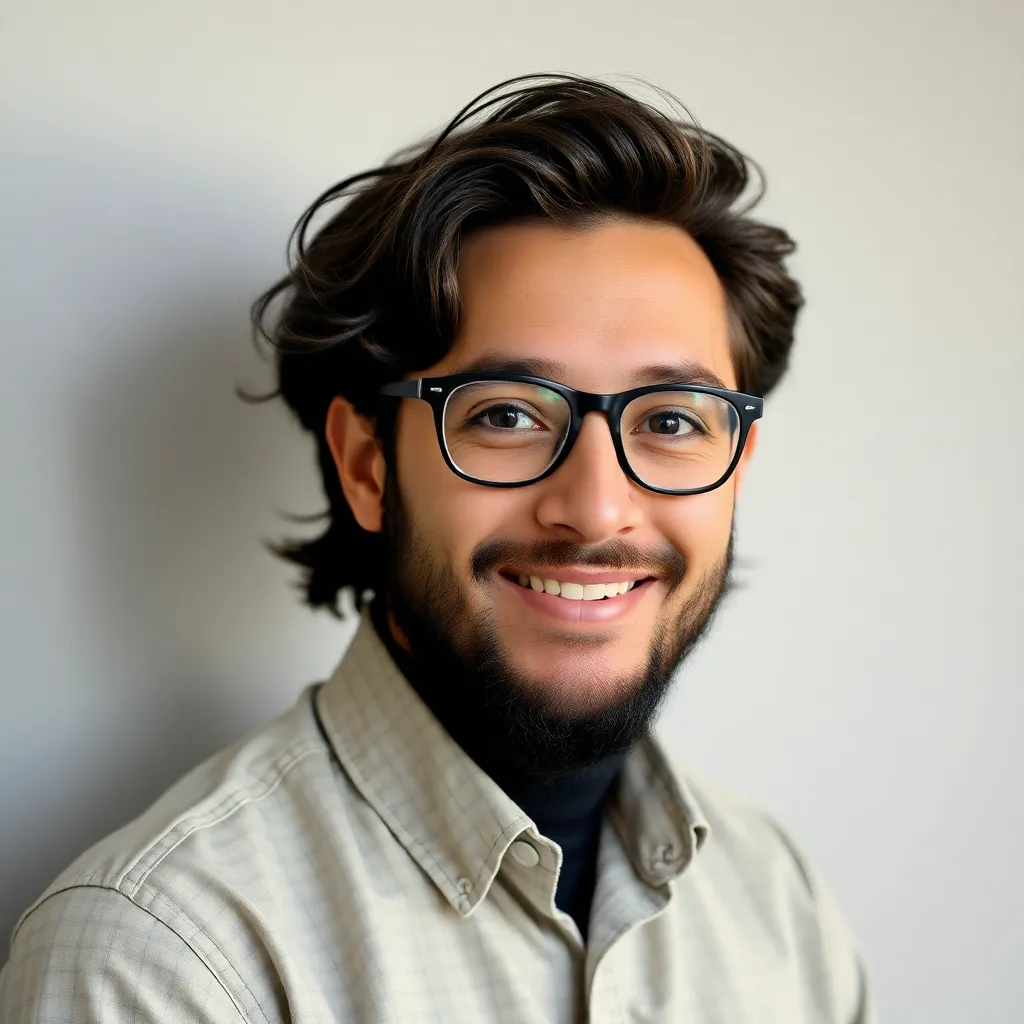
listenit
Mar 29, 2025 · 7 min read
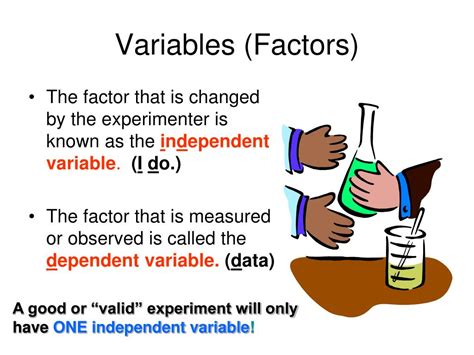
Table of Contents
What is a Factor in an Experiment? A Deep Dive into Experimental Design
Understanding the intricacies of experimental design is crucial for conducting robust and reliable research. A cornerstone of this design is the concept of a factor. This seemingly simple term holds significant weight in determining the validity and interpretability of your findings. This comprehensive guide will delve into the definition of a factor, explore its different types, and illuminate its crucial role in various experimental designs. We'll also examine how understanding factors enhances the strength of your conclusions and the impact of your research.
Defining a Factor in an Experiment
In the context of experimental design, a factor refers to an independent variable that is manipulated or controlled by the researcher to observe its effect on a dependent variable. Think of it as the cause you're investigating. It's the element you actively change or introduce to see how it influences the outcome of your experiment. Factors are also sometimes called independent variables or explanatory variables.
For instance, if you're testing the effectiveness of different fertilizers on plant growth, the factor would be the type of fertilizer. The dependent variable, or the effect, would be the plant growth (height, weight, etc.).
It's essential to differentiate a factor from a level. A level represents a specific value or category of a factor. In our fertilizer example, each type of fertilizer (e.g., fertilizer A, fertilizer B, fertilizer C) would represent a different level of the factor "type of fertilizer."
Types of Factors in Experimental Design
Factors can be categorized in several ways, each impacting the complexity and interpretation of the experiment:
1. Qualitative vs. Quantitative Factors
-
Qualitative Factors: These factors represent categories or groups that are not numerically measurable. Examples include gender (male/female), color (red/blue/green), or type of treatment (drug A/drug B/placebo). Analyzing the effects of qualitative factors often involves comparing group means or proportions.
-
Quantitative Factors: These factors are measurable on a numerical scale. Examples include temperature (measured in Celsius or Fahrenheit), dosage of a medication (measured in milligrams), or time (measured in hours or minutes). Analyzing the effects of quantitative factors frequently involves examining trends, correlations, or regression relationships.
2. Controlled vs. Uncontrolled Factors
-
Controlled Factors: These are the factors that the researcher directly manipulates and controls during the experiment. In a well-designed experiment, the researcher should strive to control as many factors as possible to isolate the effect of the factor of interest. This ensures that any observed changes in the dependent variable are primarily attributable to the manipulated factor, rather than confounding variables.
-
Uncontrolled Factors (Confounding Variables): These are factors that could potentially influence the dependent variable but are not directly controlled by the researcher. Confounding variables can significantly compromise the validity of an experiment by obscuring the true effect of the factor of interest. Researchers employ various techniques, such as randomization and statistical control, to minimize the impact of confounding variables.
3. Fixed vs. Random Factors
-
Fixed Factors: These factors have levels that are specifically chosen by the researcher, and the results of the experiment are only intended to apply to those specific levels. For example, if you are testing the effectiveness of three specific brands of fertilizer, these brands represent a fixed factor. The conclusions drawn would only apply to those three brands.
-
Random Factors: These factors have levels that are randomly selected from a larger population of possible levels. The results of the experiment are then intended to generalize to the larger population from which the levels were sampled. For instance, if you randomly selected five different farms to test fertilizer effectiveness on, "farm" would be a random factor. The results could then be generalized to other farms with similar characteristics.
The Importance of Factors in Different Experimental Designs
The role of factors is central to various experimental designs:
1. Completely Randomized Design (CRD)
In a CRD, the treatments (different levels of the factor) are randomly assigned to the experimental units. This design is straightforward and efficient for comparing the effects of different levels of a single factor. It's essential to ensure proper randomization to minimize bias and confounding variables.
2. Randomized Block Design (RBD)
RBD is used when there's a known source of variability that could affect the dependent variable. This variability is accounted for by blocking. Blocks are groups of experimental units that are relatively homogeneous with respect to this source of variation. The treatments are then randomly assigned within each block. This design increases the precision of the experiment by reducing the error variance. Consider blocking as a way to control for a nuisance factor.
3. Factorial Designs
Factorial designs involve investigating the effects of two or more factors simultaneously. These designs allow researchers to assess not only the main effects of each factor but also their interactions. Interactions occur when the effect of one factor depends on the level of another factor. Factorial designs are powerful for uncovering complex relationships between variables.
Identifying and Controlling Factors: Best Practices
The careful identification and control of factors are paramount for conducting a successful experiment. Here's how to approach it:
-
Clearly Define Your Research Question: A well-defined research question will guide you in identifying the key factors that need to be investigated.
-
Identify Potential Confounding Variables: Brainstorm all factors that could potentially influence your dependent variable, even if they are not your main focus.
-
Control Confounding Variables: Employ strategies like randomization, blocking, and statistical control to minimize the influence of confounding variables.
-
Replicate Your Experiment: Replication involves repeating the experiment multiple times with different sets of experimental units. Replication increases the precision of the estimates and allows for assessing the variability of the results.
-
Document Your Methods Thoroughly: A detailed record of your experimental design, including the factors, levels, and procedures, is critical for reproducibility and transparency.
Analyzing the Results: Interpreting the Effects of Factors
After conducting your experiment, analyzing the results involves determining the effects of the factors on the dependent variable. Statistical analysis techniques, such as analysis of variance (ANOVA), t-tests, and regression analysis, are commonly used to assess the statistical significance of the effects.
The analysis will reveal whether the differences observed between the levels of the factor are statistically significant or simply due to random chance. It is essential to interpret the results within the context of your experimental design and potential limitations.
The Significance of Factors in Real-World Applications
The concept of factors extends beyond the confines of a laboratory setting. Its application is vast and critical across numerous fields:
-
Medicine: Clinical trials use factors to assess the effectiveness of new drugs or treatments. Factors might include dosage, treatment duration, or patient characteristics.
-
Agriculture: Agricultural research employs factors to evaluate the impact of different fertilizers, irrigation techniques, or crop varieties on yield.
-
Engineering: Engineers use factors to optimize the design and performance of products, investigating the effects of different materials, manufacturing processes, or operating conditions.
-
Marketing: Marketing campaigns often test different factors such as advertising channels, messaging, or pricing strategies to maximize effectiveness.
Conclusion: Mastering the Factor in Experimental Design
The factor is an indispensable element in experimental design. Understanding its definition, types, and role in various experimental designs is crucial for planning and executing robust and reliable research. By carefully identifying, controlling, and analyzing the effects of factors, researchers can draw meaningful conclusions and contribute valuable knowledge to their respective fields. A deep understanding of factors empowers researchers to design experiments that are not only scientifically sound but also impactful and contribute to a broader understanding of the world around us. Remember, a well-defined and controlled factor is the cornerstone of a strong and reliable experiment, leading to robust and impactful results. By mastering the principles presented here, you can elevate the quality of your research and confidently contribute to your chosen field.
Latest Posts
Latest Posts
-
How To Find Inverse Of A Quadratic Function
Apr 01, 2025
-
Oz In A Fifth Of Alcohol
Apr 01, 2025
-
What Is To The 1 2 Power
Apr 01, 2025
-
Is Water Evaporating A Chemical Or Physical Change
Apr 01, 2025
-
Which Two Elements Make Up Water
Apr 01, 2025
Related Post
Thank you for visiting our website which covers about What Is A Factor In An Experiment . We hope the information provided has been useful to you. Feel free to contact us if you have any questions or need further assistance. See you next time and don't miss to bookmark.