The Symbol For Population Variance Is
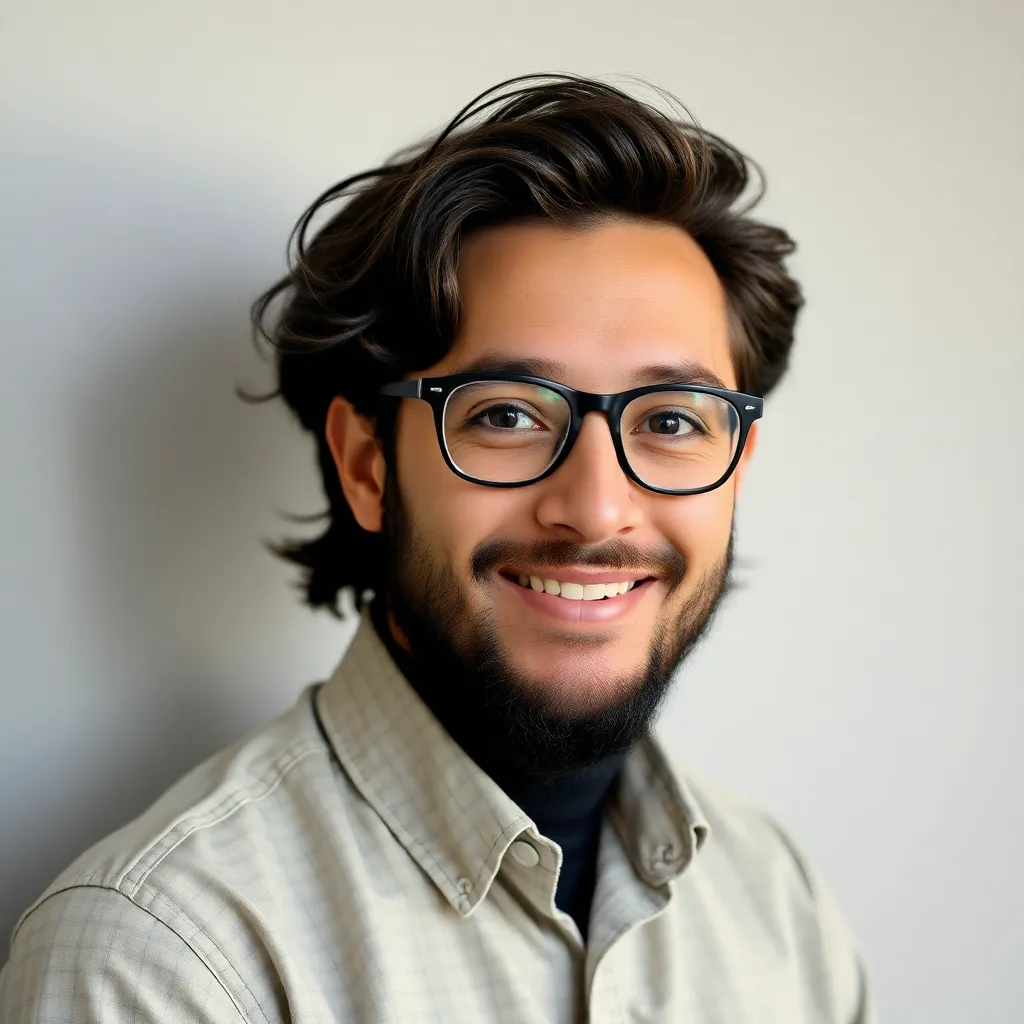
listenit
Apr 25, 2025 · 5 min read

Table of Contents
The Symbol for Population Variance: A Deep Dive into Statistical Concepts
Understanding the symbol for population variance is crucial for anyone working with statistical data. This seemingly simple symbol – σ² (sigma squared) – represents a fundamental concept in statistics that allows us to quantify the spread or dispersion of data points within a population. This article will delve into the intricacies of population variance, explaining its meaning, calculation, significance, and relationship to other statistical measures. We'll also explore the nuances that differentiate it from sample variance.
Understanding Population Variance: What Does it Mean?
Before diving into the symbol itself, let's clarify what population variance actually represents. In simple terms, population variance measures the average squared deviation of each data point from the population mean. This means it quantifies how far, on average, each individual data point is spread out from the center of the data. A higher variance indicates a greater spread, signifying more variability within the population. Conversely, a lower variance suggests data points are clustered closely around the mean, demonstrating less variability.
Imagine two datasets representing the heights of students in two different classes. One class shows heights clustered around the average height, while the other class has a wide range of heights, from very short to very tall. The class with the wider range of heights would have a significantly higher population variance.
The use of squared deviations is intentional. Squaring ensures that both positive and negative deviations contribute positively to the overall variance. This prevents positive and negative deviations from canceling each other out, providing a more accurate representation of the overall spread.
Why is Population Variance Important?
Understanding population variance is crucial for several reasons:
- Risk Assessment: In finance, variance is used to assess the risk associated with an investment. A higher variance indicates higher volatility and thus, higher risk.
- Process Control: In manufacturing, variance helps monitor the consistency of a production process. High variance suggests inconsistencies and potential quality control issues.
- Data Analysis: In research, variance helps assess the reliability and validity of data. Low variance indicates more precise and consistent measurements.
- Predictive Modeling: Variance plays a vital role in various statistical models, including regression analysis, where it helps estimate the accuracy of predictions.
The Symbol: σ² (Sigma Squared)
The symbol for population variance is σ² (sigma squared). Let's break this down:
-
σ (sigma): This lowercase Greek letter represents the population standard deviation. The standard deviation is the square root of the variance, providing a measure of spread in the original units of the data.
-
² (squared): The superscript '2' indicates that the variance is the square of the standard deviation. This is crucial because the variance is calculated using squared deviations.
Therefore, σ² directly represents the population variance, providing a concise and universally understood symbol within the field of statistics.
Calculating Population Variance: A Step-by-Step Guide
The formula for calculating population variance is:
σ² = Σ(xi - μ)² / N
Where:
- σ²: Population variance
- Σ: Summation (add up all the values)
- xi: Individual data points
- μ: Population mean (average of all data points)
- N: Total number of data points in the population
Here's a breakdown of the calculation process:
-
Calculate the population mean (μ): Add up all the data points and divide by the total number of data points.
-
Calculate the deviation of each data point from the mean (xi - μ): Subtract the mean from each individual data point.
-
Square each deviation [(xi - μ)²]: Square each of the deviations calculated in step 2. This ensures all values are positive.
-
Sum the squared deviations [Σ(xi - μ)²]: Add up all the squared deviations.
-
Divide by the total number of data points (N): Divide the sum of squared deviations by the total number of data points in the population. This provides the average squared deviation, which is the population variance.
Example Calculation:
Let's say we have the following data points representing the ages of five individuals in a family: 25, 28, 30, 32, 35.
-
Mean (μ): (25 + 28 + 30 + 32 + 35) / 5 = 30
-
Deviations:
- 25 - 30 = -5
- 28 - 30 = -2
- 30 - 30 = 0
- 32 - 30 = 2
- 35 - 30 = 5
-
Squared Deviations:
- (-5)² = 25
- (-2)² = 4
- (0)² = 0
- (2)² = 4
- (5)² = 25
-
Sum of Squared Deviations: 25 + 4 + 0 + 4 + 25 = 58
-
Population Variance (σ²): 58 / 5 = 11.6
Therefore, the population variance of the ages in this family is 11.6.
Population Variance vs. Sample Variance: Key Differences
It's crucial to distinguish between population variance (σ²) and sample variance (s²). While both measure the spread of data, they differ in their application and calculation:
-
Population Variance (σ²): Calculated using data from the entire population. It represents the true variance of the entire group being studied.
-
Sample Variance (s²): Calculated using data from a sample taken from the population. It's an estimate of the population variance, used when it's impossible or impractical to collect data from the entire population.
The key difference in calculation lies in the denominator:
-
Population Variance: Divided by the total number of data points (N)
-
Sample Variance: Divided by the number of data points minus 1 (N-1) – this is known as Bessel's correction, which helps to provide a less biased estimate of the population variance when using a sample.
The symbol for sample variance is s², clearly differentiating it from the population variance symbol σ².
The Significance of Understanding Variance
Understanding variance, and its associated symbol σ², is fundamental to many areas of statistical analysis. It allows for a quantitative assessment of data spread, providing insights into data variability, risk assessment, process control, and the reliability of research findings. The distinction between population and sample variance is equally crucial for accurate statistical inference. Mastering these concepts is essential for anyone working with data analysis and statistical modeling. This knowledge empowers informed decision-making based on a solid understanding of data characteristics. The ability to interpret and utilize variance calculations significantly enhances critical thinking skills and improves the overall quality of analyses. A deep understanding of variance is a key component of becoming a proficient data analyst.
Latest Posts
Latest Posts
-
The Atoms Along The Staircase Are Called
Apr 25, 2025
-
The Three Main Groups Of Carbohydrates Are
Apr 25, 2025
-
How Many Unpaired Electrons Does Nickel Have
Apr 25, 2025
-
Can An Atom Be Broken Down
Apr 25, 2025
-
Specific Heat Is Measured In What Units
Apr 25, 2025
Related Post
Thank you for visiting our website which covers about The Symbol For Population Variance Is . We hope the information provided has been useful to you. Feel free to contact us if you have any questions or need further assistance. See you next time and don't miss to bookmark.