The Is The Best Point Estimate Of The Population Mean
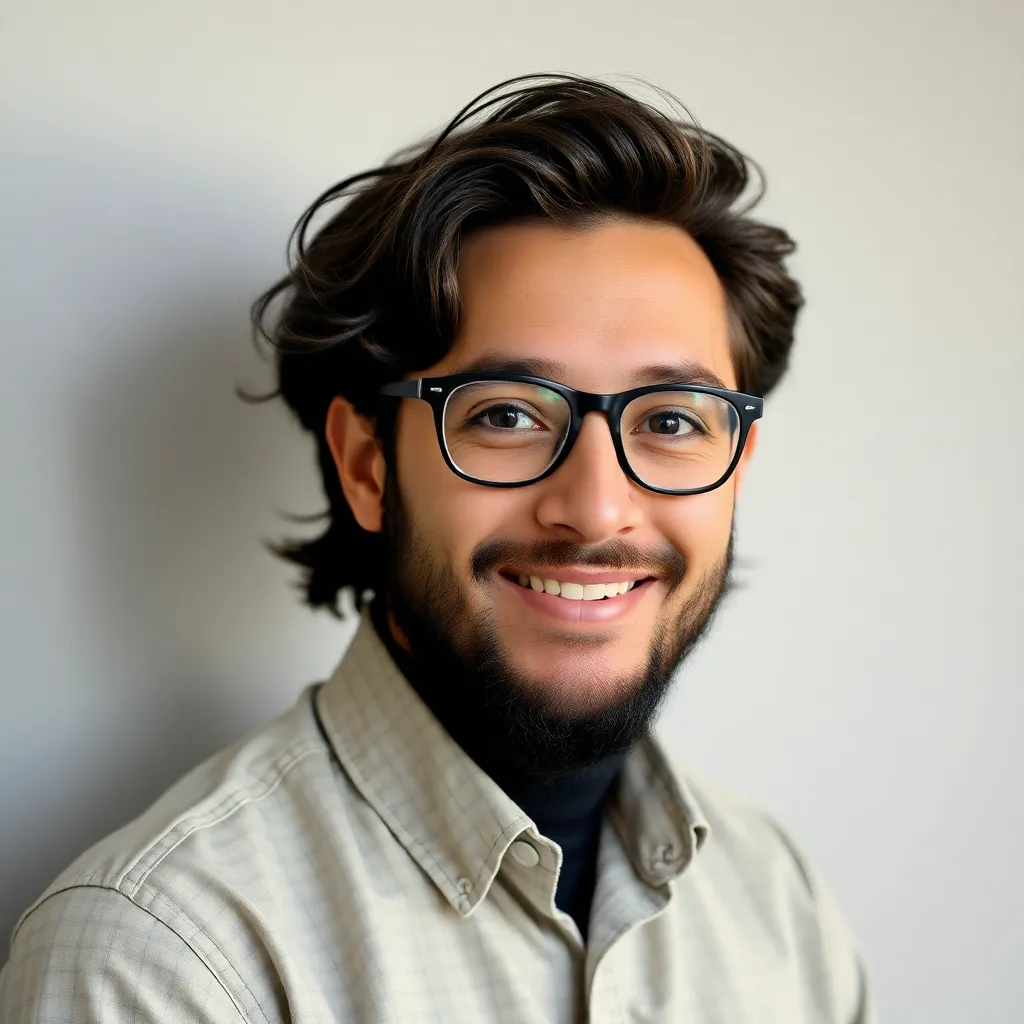
listenit
May 09, 2025 · 6 min read
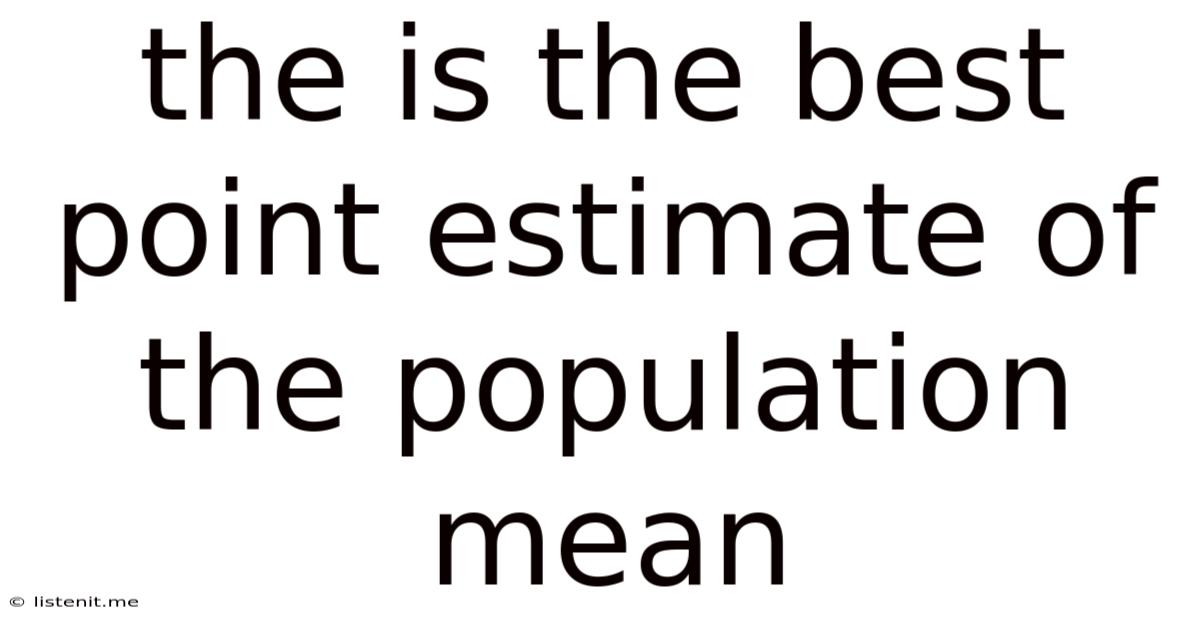
Table of Contents
The Sample Mean: The Best Point Estimate of the Population Mean
Understanding population parameters is crucial in statistics, but directly measuring them is often impractical or impossible. This is where point estimation comes in, allowing us to infer population characteristics from sample data. Among various point estimators, the sample mean (x̄) stands out as the best point estimate of the population mean (μ). This article will delve deep into why this is the case, exploring the underlying statistical principles and demonstrating its superiority through various properties and comparisons with alternative estimators.
Why the Sample Mean Reigns Supreme
The sample mean's dominance as the best point estimator for the population mean stems from several key properties:
1. Unbiasedness: Hitting the Bullseye on Average
An unbiased estimator is one whose expected value equals the true population parameter. In simpler terms, if we were to repeatedly sample from the population and calculate the sample mean each time, the average of all these sample means would converge to the true population mean. This is a critical characteristic. A biased estimator consistently overestimates or underestimates the true value, leading to inaccurate inferences. The sample mean, however, boasts this crucial property of unbiasedness: E(x̄) = μ.
2. Efficiency: Minimizing the Variability
While unbiasedness ensures that our estimate is centered around the true value on average, we also want our estimator to be precise. Efficiency measures how closely our estimates cluster around the true value. The sample mean is the most efficient estimator among all unbiased linear estimators of the population mean. This means it has the smallest variance compared to other unbiased estimators. A smaller variance implies that our estimates are less spread out, making them more reliable and closer to the true population mean. This efficiency is mathematically proven and is a cornerstone of its superiority. This is particularly important when dealing with limited sample sizes, as a more efficient estimator provides a more precise estimate despite the limited data.
3. Consistency: Accuracy Improves with More Data
A consistent estimator's accuracy improves as the sample size increases. As we collect more data, the sample mean converges to the true population mean with increasing probability. This is intuitive; the more data we have, the better our representation of the population. The Law of Large Numbers formally supports this, ensuring that as the sample size (n) approaches infinity, the sample mean (x̄) converges to the population mean (μ). This property is vital for ensuring that our estimate becomes increasingly reliable as our data grows.
4. Sufficiency: Utilizing All Available Information
A sufficient statistic summarizes all the relevant information about the population parameter contained within the sample data. The sample mean utilizes all the individual data points to generate a single estimate. No other statistic derived from the same sample can provide additional information about the population mean beyond what the sample mean already encapsulates. This means that discarding any data point would lead to a loss of information, highlighting the comprehensiveness of the sample mean as an estimator.
Comparing the Sample Mean to Other Estimators
Let's compare the sample mean to other potential estimators of the population mean to further solidify its superiority:
1. The Sample Median: A Robust but Less Efficient Alternative
The sample median, the middle value in a sorted dataset, is a robust estimator, less sensitive to outliers than the sample mean. However, in the case of a normally distributed population (a common assumption in many statistical analyses), the sample mean is considerably more efficient. The median's robustness is advantageous when dealing with skewed distributions or the presence of outliers, but this robustness comes at the cost of efficiency. In a symmetric distribution like a normal distribution, the mean and median coincide; however, in skewed distributions, the median is often a better representation of the central tendency, albeit with a loss in efficiency compared to the mean. The choice between the mean and median often depends on the nature of the data and the desired properties of the estimator.
2. Trimmed Means: A Compromise Between Robustness and Efficiency
Trimmed means involve removing a certain percentage of the highest and lowest values before calculating the mean. This method aims to strike a balance between the robustness of the median and the efficiency of the mean. By discarding extreme values, trimmed means reduce the influence of outliers while maintaining a reasonable level of efficiency. The optimal trimming percentage depends on the specifics of the data and the desired level of robustness. While trimmed means can be useful in specific contexts, they still don't match the overall efficiency of the sample mean in normally distributed datasets.
3. Weighted Averages: Prioritizing Certain Data Points
Weighted averages assign different weights to individual data points, reflecting their perceived importance or reliability. This approach can be useful when some data points are considered more informative than others. For example, data from more reliable sources might receive higher weights. However, the choice of weights is subjective and might introduce bias. The simple sample mean avoids this subjectivity and maintains unbiasedness. Using a weighted average efficiently requires in-depth knowledge of data sources and their reliability.
Assumptions and Limitations
While the sample mean is generally considered the best point estimate of the population mean, certain assumptions and limitations are worth noting:
-
Random Sampling: The sample mean's properties rely on the assumption that the data is obtained through a random sampling method. Non-random sampling can introduce biases that invalidate the estimator's properties.
-
Normality (for inference): While the sample mean's unbiasedness and efficiency hold regardless of the distribution, inferences about the population mean (like constructing confidence intervals) often rely on the assumption of normality or a large sample size (due to the Central Limit Theorem).
-
Outliers: Extreme outliers can significantly influence the sample mean, potentially leading to a misleading estimate. Robust estimators like the median or trimmed mean might be preferred in such scenarios.
Conclusion: The Sample Mean's Enduring Importance
In summary, the sample mean (x̄) stands out as the best point estimate of the population mean (μ) due to its unbiasedness, efficiency, consistency, and sufficiency. While alternative estimators exist, each comes with its own trade-offs. The sample mean's superiority is particularly pronounced in normally distributed populations or large samples where the Central Limit Theorem applies. However, understanding the assumptions and limitations of the sample mean is essential to ensure appropriate application and interpretation of results. Choosing the right estimator depends on the characteristics of the data and the goals of the analysis, but for many common statistical applications, the sample mean remains the gold standard. Its mathematical properties, consistently proven through decades of statistical research, solidify its place as a fundamental tool in statistical inference and data analysis. Its enduring importance underscores its power and reliability in extracting meaningful insights from data.
Latest Posts
Latest Posts
-
Countercurrent Multiplier In Loop Of Henle
May 09, 2025
-
What Are The Biomes In Australia
May 09, 2025
-
Greatest Common Factor Of 15 And 6
May 09, 2025
-
Elements That Are Named After Places
May 09, 2025
-
What Is The Gcf Of 18 And 12
May 09, 2025
Related Post
Thank you for visiting our website which covers about The Is The Best Point Estimate Of The Population Mean . We hope the information provided has been useful to you. Feel free to contact us if you have any questions or need further assistance. See you next time and don't miss to bookmark.