Real Life Examples Of Normal Distribution
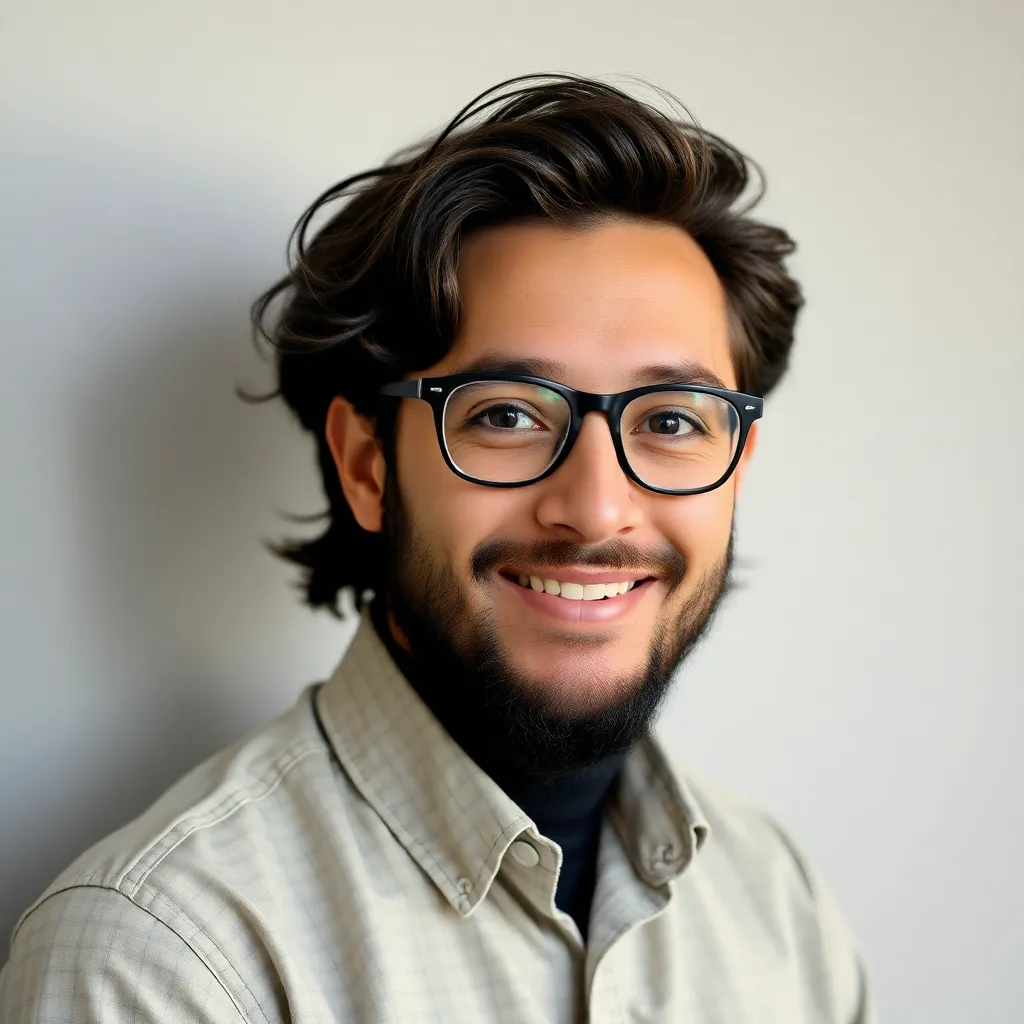
listenit
Apr 17, 2025 · 6 min read

Table of Contents
Real-Life Examples of the Normal Distribution: Understanding the Bell Curve's Impact
The normal distribution, also known as the Gaussian distribution or bell curve, is a fundamental concept in statistics and probability. Its characteristic bell shape arises from the concentration of data around a central mean, with symmetrical tapering towards the extremes. While a perfectly normal distribution is rarely observed in real-world scenarios, many natural and man-made phenomena approximate this distribution closely enough to be modeled effectively using its properties. Understanding the normal distribution is crucial for analyzing data, making predictions, and drawing meaningful conclusions in numerous fields. This article will explore a wide range of real-life examples showcasing the prevalence and applications of the normal distribution.
Natural Phenomena Following a Normal Distribution
Nature often exhibits patterns that surprisingly resemble the normal distribution. This is often due to the Central Limit Theorem, which states that the average of many independent and identically distributed random variables tends towards a normal distribution, regardless of the original distribution's shape.
1. Human Height and Weight: A Classic Example
One of the most cited examples is human height and weight. Within a specific population (e.g., adult males in a particular country), the distribution of heights and weights tends to follow a normal distribution. Most individuals cluster around the average height and weight, with fewer people at significantly shorter or taller, lighter or heavier extremes. Factors like genetics, nutrition, and environmental influences contribute to the variation, but the overall pattern approximates a bell curve.
2. Blood Pressure and Cholesterol Levels: Vital Health Indicators
Similar to height and weight, blood pressure and cholesterol levels within a population often exhibit a normal distribution. While individual readings fluctuate due to various factors, the average readings within a large population tend to be normally distributed. This characteristic allows healthcare professionals to establish normal ranges, identify outliers who may be at increased risk, and tailor interventions accordingly. Deviations from the normal distribution can signal underlying health issues.
3. Measurement Errors in Scientific Experiments: Minimizing Bias
In scientific experiments, measurement errors are often normally distributed. These errors can arise from various sources, including instrument imprecision, observer bias, and random fluctuations in the environment. Because these errors are often random and independent, their distribution tends toward normality. Understanding this allows scientists to assess the accuracy and reliability of their experimental data. Statistical techniques can help to filter out these errors and reveal the underlying signal.
4. IQ Scores: A Standardized Measure of Intelligence
IQ scores are designed to be normally distributed, with a mean of 100 and a standard deviation of 15. While the exact distribution may not be perfectly normal across all populations, the standardization ensures a consistent benchmark for comparing intellectual capabilities across individuals and groups. This normality allows for easy interpretation and comparison of scores.
Man-Made Phenomena Approximating Normal Distribution
The normal distribution isn't confined to natural phenomena; many man-made processes and outcomes also exhibit a normal or near-normal distribution.
5. Manufacturing and Production Processes: Ensuring Quality Control
In manufacturing, the dimensions of products often follow a normal distribution. For example, the diameter of manufactured bolts or the weight of packaged goods will typically exhibit slight variations due to manufacturing tolerances. Statistical process control (SPC) utilizes the normal distribution to set acceptable limits and detect anomalies that signal production issues. Continuous monitoring and adjustment of the manufacturing process aim to maintain a tight, normally distributed output, thereby ensuring product quality and consistency.
6. Exam Scores: Gauging Student Performance
In large-scale standardized tests, the distribution of scores often approximates a normal distribution. This is due to the large number of students taking the exam and the various factors influencing their performance. The normal distribution allows for the creation of percentile rankings, allowing for fair comparison among students. Educators use this distribution to evaluate curriculum effectiveness, identify areas for improvement, and assess individual student strengths and weaknesses.
7. Stock Prices and Market Returns: Predicting Financial Fluctuations
While stock prices exhibit volatility and don't strictly follow a normal distribution (especially during periods of market turbulence), their short-term returns often approximate normality. This assumption is often made in financial modeling, although it is essential to acknowledge its limitations, especially during extreme events like market crashes or significant economic shifts. The normal distribution provides a framework for assessing risk and return, although more sophisticated models are often necessary to capture the true complexity of financial markets.
8. Error Rates in Data Transmission: Ensuring Reliable Communication
In digital communication systems, random noise introduces errors during data transmission. This error rate often follows a normal distribution, with the majority of transmissions being error-free and a smaller number experiencing errors. Understanding this normal distribution allows for the design of error-correction codes and techniques to enhance data reliability. This is crucial for ensuring seamless communication across various platforms.
9. Actuarial Science: Assessing Risk and Setting Premiums
Actuarial science heavily relies on statistical models, frequently employing the normal distribution. Actuaries assess risk and determine insurance premiums by considering various factors that influence the occurrence of insured events. The normal distribution, even with its limitations when modeling extreme events, helps calculate the likelihood of different claim scenarios, assisting in setting premiums that accurately reflect the risk level.
Limitations and Considerations: When the Normal Distribution Doesn't Apply
It's crucial to understand that while the normal distribution is a powerful tool, its applicability is not universal. Many real-world phenomena deviate significantly from normality, requiring alternative statistical approaches.
Skewed Distributions: Income and Wealth Inequality
Income and wealth distributions, for instance, are often highly skewed, with a few individuals possessing significantly more wealth than the majority. These distributions are not well-represented by the normal distribution, necessitating alternative statistical models that account for asymmetry and heavy tails.
Bimodal or Multimodal Distributions: Data with Multiple Peaks
Data that has two or more distinct peaks (bimodal or multimodal) cannot be effectively modeled with a single normal distribution. Examples include distributions of heights in a mixed population of adult men and women, or the age distribution of a population which has undergone significant demographic changes.
Extreme Events: Black Swan Events and Outliers
The normal distribution struggles to capture the probability of extreme events, also known as “black swan” events. These events are rare, impactful occurrences that fall far outside the typical range of variation. The normal distribution underestimates the likelihood of such outliers, which is why other distributions, such as heavy-tailed distributions, are more appropriate in these contexts.
Conclusion: The Versatility and Importance of the Normal Distribution
Despite its limitations, the normal distribution remains a cornerstone of statistical analysis. Its symmetrical bell shape and well-defined mathematical properties make it exceptionally versatile. Its applicability across various fields, ranging from natural phenomena to man-made processes, highlights its importance in understanding, predicting, and interpreting data. While it's essential to recognize when the normal distribution is an inadequate model, its widespread use underscores its significant role in quantitative analysis and decision-making. Understanding its strengths and limitations empowers us to apply it appropriately and effectively in a multitude of contexts. Remembering that the real world is complex, and while approximations are useful, a critical and nuanced approach is vital for robust and reliable analyses.
Latest Posts
Latest Posts
-
How To Convert Mmhg To Pa
Apr 19, 2025
-
Convert From Rectangular To Polar Coordinates
Apr 19, 2025
-
What Is The Lcm Of 4 And 14
Apr 19, 2025
-
Which Quantities Are Conserved In All Chemical Reactions
Apr 19, 2025
-
How Many Atoms Are In 3 5 Moles Of Arsenic Atoms
Apr 19, 2025
Related Post
Thank you for visiting our website which covers about Real Life Examples Of Normal Distribution . We hope the information provided has been useful to you. Feel free to contact us if you have any questions or need further assistance. See you next time and don't miss to bookmark.