Probability Density Function Of Chi Square Distribution
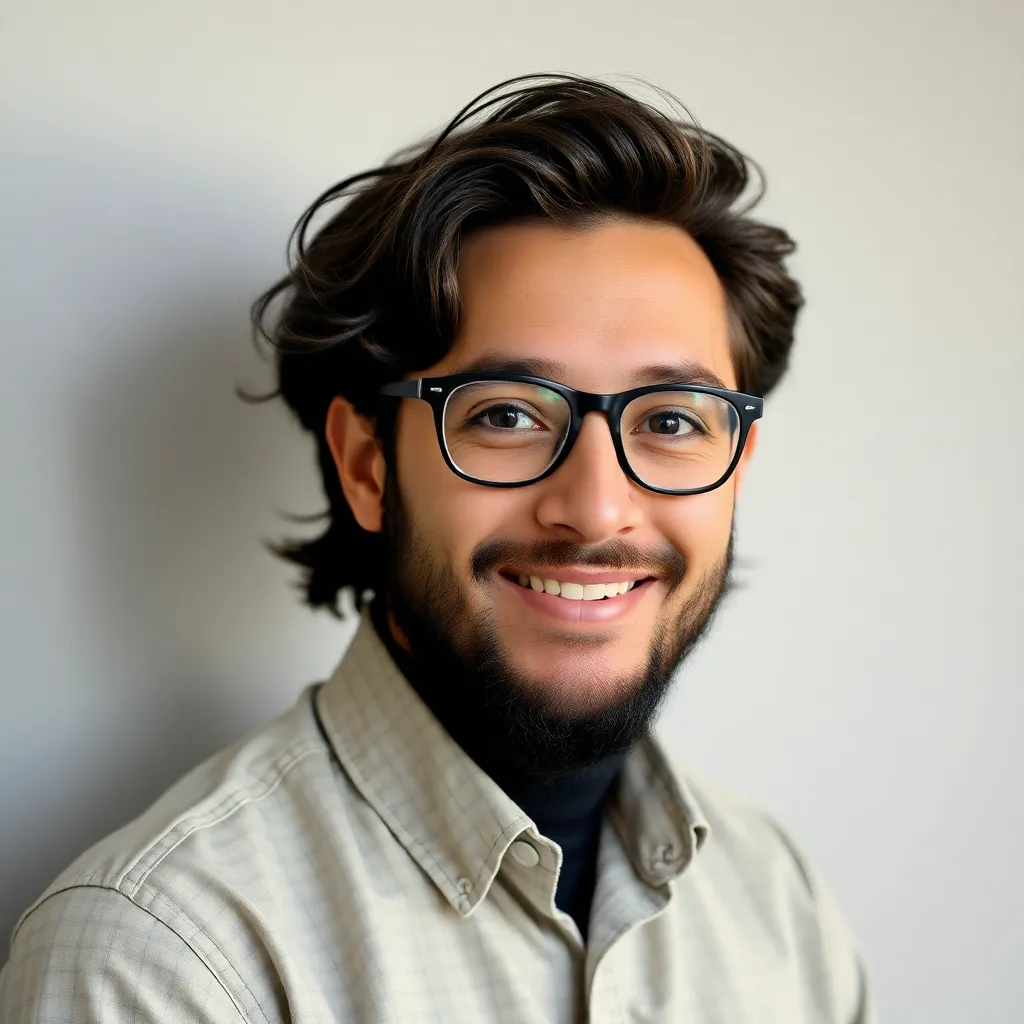
listenit
May 12, 2025 · 6 min read
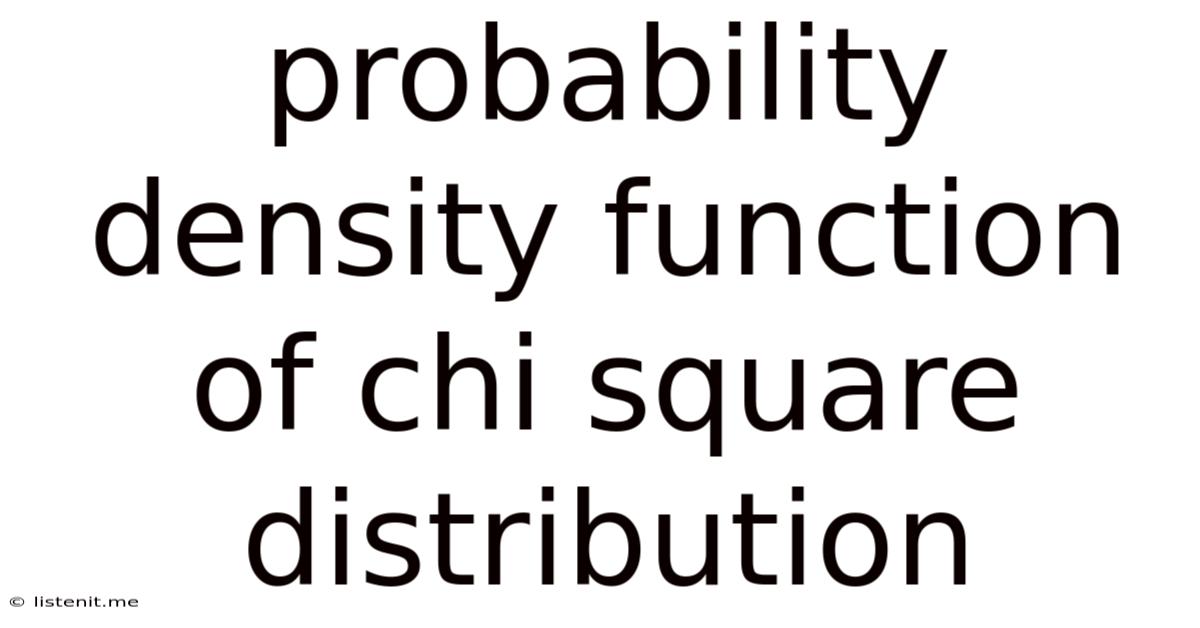
Table of Contents
Understanding the Probability Density Function of the Chi-Square Distribution
The chi-square (χ²) distribution is a widely used probability distribution in statistics, particularly in hypothesis testing and confidence interval estimation. Understanding its probability density function (PDF) is crucial for effectively applying this distribution in various statistical analyses. This article delves deep into the chi-square distribution's PDF, exploring its properties, derivation, applications, and interpretations.
What is the Chi-Square Distribution?
Before diving into the PDF, let's establish a foundational understanding of the chi-square distribution itself. It's a continuous probability distribution that arises frequently when dealing with the sum of squared independent standard normal random variables. Specifically, if you have k independent random variables, each following a standard normal distribution (mean = 0, variance = 1), then the sum of their squares follows a chi-square distribution with k degrees of freedom. This is often denoted as χ²(k).
The degrees of freedom (k) parameter is a crucial characteristic of the chi-square distribution. It determines the shape and spread of the distribution. A higher degree of freedom leads to a more symmetric and less skewed distribution, approaching a normal distribution as k becomes large.
The Probability Density Function (PDF) of the Chi-Square Distribution
The probability density function (PDF) of a chi-square distribution with k degrees of freedom is defined as:
f(x; k) = {1/[2^(k/2)Γ(k/2)]} * x^((k/2)-1) * e^(-x/2)
where:
- x ≥ 0: The chi-square variable can only take on non-negative values.
- k > 0: The degrees of freedom must be positive.
- Γ(k/2): This represents the gamma function, a generalization of the factorial function to complex numbers. The gamma function is essential for calculating the normalization constant ensuring the total area under the curve equals 1.
Understanding the Components of the PDF
Let's break down each component of the PDF:
-
1/[2^(k/2)Γ(k/2)]: This is the normalization constant. It ensures that the integral of the PDF over its entire domain (from 0 to ∞) equals 1, a fundamental property of all probability density functions. This constant scales the function to represent a valid probability distribution.
-
x^((k/2)-1): This term determines the shape of the distribution for different values of k. For small values of k, the distribution is highly skewed to the right. As k increases, this skewness diminishes.
-
e^(-x/2): This is the exponential term, which causes the PDF to decay rapidly as x increases. This ensures that the probability mass is concentrated towards lower values of x, especially when k is small.
Derivation of the Chi-Square PDF (Advanced)
Deriving the chi-square PDF involves several steps requiring a solid understanding of mathematical statistics and calculus. Here's a simplified outline:
-
Start with the joint probability density function of k independent standard normal random variables. This will be a multivariate Gaussian distribution.
-
Perform a transformation of variables. Introduce new variables representing the sum of the squares of the standard normal variables. This is the core of the derivation. The Jacobian determinant of this transformation is needed to account for the change in volume element.
-
Integrate out the nuisance variables. The integration over the nuisance variables will simplify the joint distribution, leaving only the distribution of the sum of squares.
-
Apply the Gamma function. The resulting integral will involve the Gamma function, leading to the final form of the chi-square PDF.
This derivation is mathematically intensive and beyond the scope of a detailed explanation in this article. However, understanding the core steps provides a deeper appreciation for the structure and origins of the PDF. Advanced statistical textbooks provide comprehensive derivations.
Properties of the Chi-Square Distribution and its PDF
The chi-square distribution and its PDF possess several important properties:
-
Non-negativity: The chi-square variable can only take on non-negative values (x ≥ 0). This is reflected in the PDF's definition, which is only defined for x ≥ 0.
-
Additivity: The sum of independent chi-square random variables is also a chi-square random variable. The degrees of freedom of the resulting variable is the sum of the degrees of freedom of the individual variables.
-
Asymptotic Normality: As the degrees of freedom (k) increases, the chi-square distribution approaches a normal distribution. This is a crucial property for large-sample approximations.
-
Mean and Variance: The mean of a chi-square distribution with k degrees of freedom is k, and its variance is 2k.
Applications of the Chi-Square Distribution and its PDF
The chi-square distribution finds widespread applications in various statistical contexts. Understanding its PDF is instrumental in these applications:
-
Goodness-of-fit tests: The chi-square test is commonly used to assess how well observed data fit a theoretical distribution. The PDF helps calculate the probability of observing data as extreme or more extreme than what was actually observed, enabling us to determine the significance of the discrepancy.
-
Tests of independence: This test examines whether two categorical variables are independent. The PDF is used to calculate the p-value, determining if the observed association between the variables is statistically significant.
-
Confidence intervals for variance: The chi-square distribution plays a critical role in constructing confidence intervals for population variance, particularly when the underlying data follows a normal distribution. The PDF allows for the calculation of the appropriate critical values.
-
Analysis of variance (ANOVA): ANOVA utilizes the chi-square distribution to test for differences between the means of multiple groups. The PDF aids in calculating F-statistics and p-values.
-
Survival Analysis: The chi-square distribution is also used in some aspects of survival analysis, particularly when testing for differences in survival times between groups.
Interpreting the PDF Graphically
Visualizing the chi-square PDF for different degrees of freedom is extremely helpful for understanding its behavior. The graph demonstrates:
-
Right Skewness (for small k): For small values of k, the distribution is heavily skewed to the right, indicating a higher probability of observing smaller values of χ².
-
Symmetry (for large k): As k increases, the distribution becomes more symmetric, approaching a normal distribution. The peak shifts to the right, and the spread increases.
-
Area under the curve: The total area under the curve always equals 1, representing the total probability. The area under a specific portion of the curve represents the probability of observing a χ² value within that range.
By examining the graph, you can intuitively grasp the probability of observing specific ranges of χ² values for a given number of degrees of freedom. This visual interpretation is crucial for understanding the results of hypothesis tests that utilize the chi-square distribution.
Conclusion
The probability density function of the chi-square distribution is a fundamental concept in statistics. Its understanding is essential for conducting various hypothesis tests and constructing confidence intervals. While the mathematical derivation can be complex, grasping the core components of the PDF and its properties allows for a more intuitive application of the chi-square distribution in statistical analysis. By combining the theoretical understanding with visual representations of the PDF, statisticians can effectively interpret results and draw meaningful conclusions from their data. Further exploration of this topic should involve working through practical examples and using statistical software to visualize the PDF for various degrees of freedom, solidifying comprehension and strengthening analytical skills.
Latest Posts
Latest Posts
-
How Many Combinations With 2 Numbers
May 12, 2025
-
An Element That Is A Gas At Room Temperature
May 12, 2025
-
Slope Of Line Parallel To Y Axis
May 12, 2025
-
What Is The Fraction For 20 Percent
May 12, 2025
-
Find The Value Of X And Z
May 12, 2025
Related Post
Thank you for visiting our website which covers about Probability Density Function Of Chi Square Distribution . We hope the information provided has been useful to you. Feel free to contact us if you have any questions or need further assistance. See you next time and don't miss to bookmark.