Is The Standard Deviation Resistant To Outliers
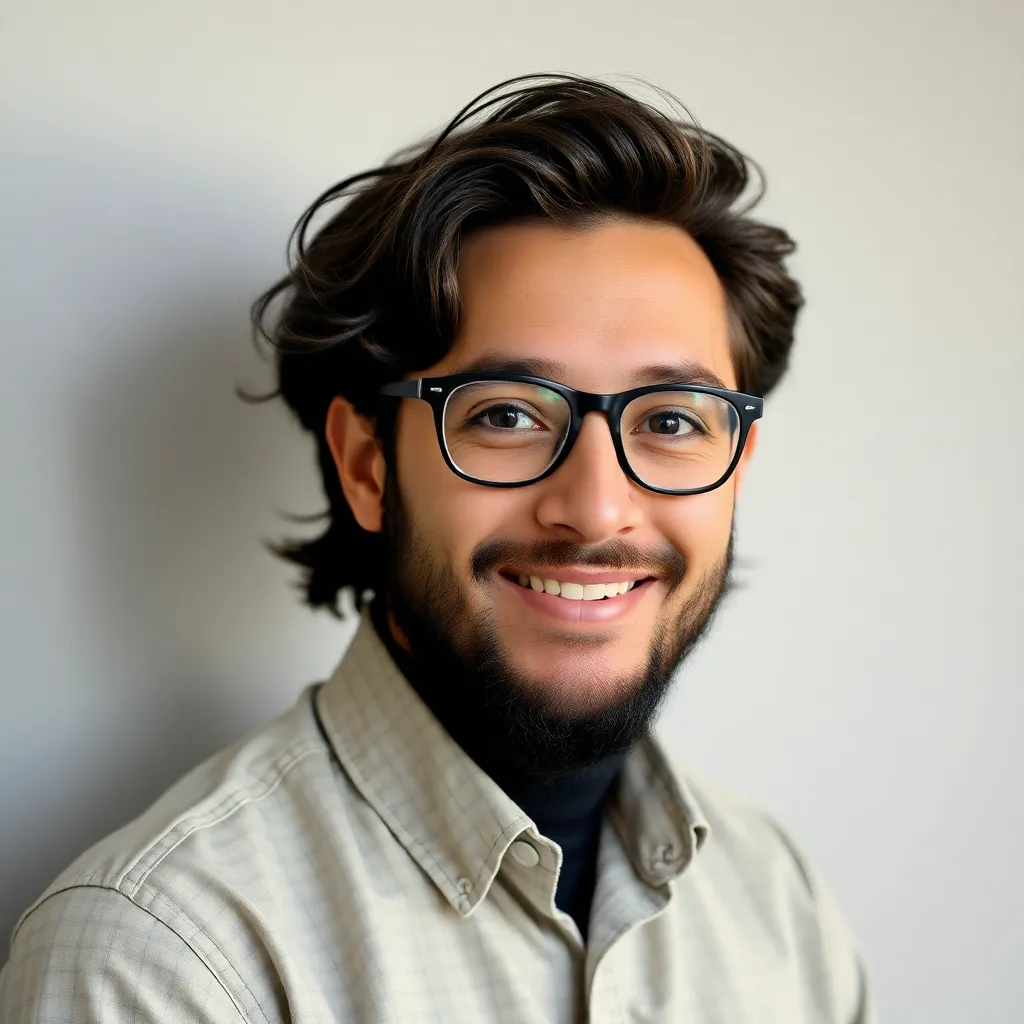
listenit
May 10, 2025 · 6 min read
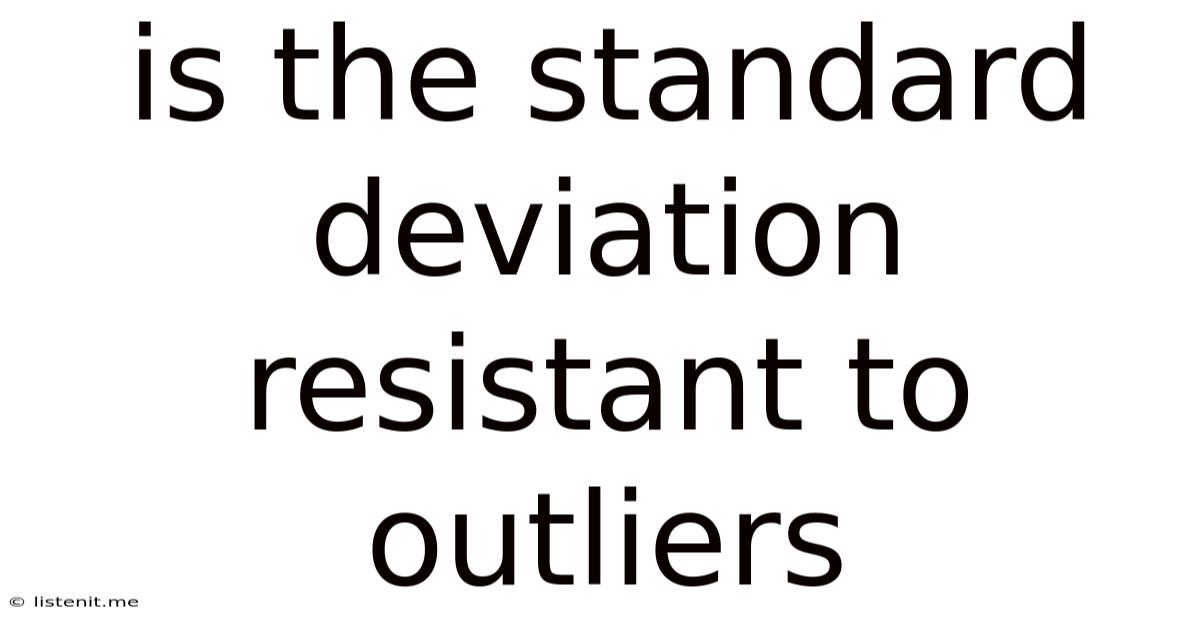
Table of Contents
Is the Standard Deviation Resistant to Outliers? A Deep Dive
The standard deviation, a cornerstone of descriptive statistics, measures the dispersion or spread of a dataset around its mean. It quantifies how much individual data points deviate from the average. But a critical question arises: how robust is the standard deviation to outliers? The short answer is: no, the standard deviation is not resistant to outliers. Outliers, those extreme values significantly different from the rest of the data, exert a disproportionate influence on the standard deviation, inflating its value and potentially misrepresenting the true variability of the dataset. This article will delve into the reasons why, exploring the mathematical underpinnings and providing practical examples to illustrate the impact of outliers. We'll also discuss alternative measures of dispersion that are more robust to extreme values.
Understanding the Standard Deviation
Before exploring the impact of outliers, let's revisit the formula for calculating the standard deviation (σ):
σ = √[ Σ(xi - μ)² / N ]
Where:
- xi: Represents each individual data point.
- μ: Represents the mean (average) of the dataset.
- N: Represents the total number of data points.
- Σ: Represents the summation of all values.
The formula essentially calculates the average of the squared differences between each data point and the mean. Squaring these differences ensures that both positive and negative deviations contribute equally to the overall dispersion. The square root is then taken to return the result to the original units of measurement.
The Impact of Outliers on the Standard Deviation
The crucial aspect of the standard deviation formula that makes it susceptible to outliers is the squaring of the deviations. Outliers, by definition, have large deviations from the mean. When squared, these large deviations become even larger, dramatically increasing the sum of squared deviations (Σ(xi - μ)²). This inflated sum directly impacts the final standard deviation, resulting in an overestimation of the true data variability.
Consider a simple example:
Dataset A: 10, 12, 13, 14, 15
Dataset B: 10, 12, 13, 14, 100
Dataset A has a mean of 12.8 and a standard deviation of approximately 1.9. Dataset B, with only one outlier (100), has a mean of 27.8 and a significantly larger standard deviation of approximately 36. The single outlier drastically inflates the standard deviation, giving a misleading impression of the data's spread. The majority of the data points in Dataset B are clustered around 10-14, yet the standard deviation suggests a much wider spread.
Why Squaring Amplifies Outlier Influence
The squaring operation acts as a magnifying glass for outliers. Small deviations are squared and contribute relatively little to the overall sum. However, large deviations (those associated with outliers) are amplified exponentially. This leads to a situation where a single outlier can dominate the calculation of the standard deviation, outweighing the influence of numerous data points clustered closely together. The effect is disproportionate and leads to a biased measure of dispersion.
Visualizing the Impact
Imagine plotting the datasets on a graph. Dataset A would show a tight cluster of points. Dataset B, however, would show a much wider spread due to the outlier, even though most of the data points remain relatively close together. The standard deviation, in the case of Dataset B, reflects this exaggerated spread caused by the outlier, while visually inspecting the graph reveals a different story.
Alternative Measures of Dispersion: Robust Alternatives to Standard Deviation
Because of the sensitivity of the standard deviation to outliers, statisticians often employ more robust measures of dispersion, particularly when dealing with datasets that may contain outliers:
1. Interquartile Range (IQR): The IQR is the difference between the third quartile (Q3) and the first quartile (Q1) of a dataset. It represents the spread of the middle 50% of the data and is completely unaffected by outliers lying outside this range. This makes it significantly more robust than the standard deviation.
2. Median Absolute Deviation (MAD): The MAD measures the average absolute deviation from the median, not the mean. Because it uses the median (which is less sensitive to outliers than the mean), and uses absolute deviations (which avoids the squaring effect that amplifies outliers), the MAD is a robust measure of dispersion.
3. Trimmed Standard Deviation: This method involves removing a certain percentage of the highest and lowest values before calculating the standard deviation. This reduces the influence of outliers but still uses information from the majority of the data points.
Choosing the Right Measure of Dispersion
The choice of the appropriate measure of dispersion hinges on the nature of the dataset and the specific research question. If the dataset is known to be free of outliers, or if the focus is on the overall variability including extreme values, the standard deviation may be an appropriate choice. However, if the dataset may contain outliers, and the goal is to describe the typical spread excluding extreme values, then more robust measures like the IQR or MAD are preferred.
Dealing with Outliers: Identification and Treatment
Before opting for a robust measure of dispersion, it is crucial to critically examine the presence and potential causes of outliers. Outliers may arise from genuine natural variation or measurement errors.
Identifying Outliers: Several techniques can be used to identify outliers. Box plots offer a visual representation of the data distribution, readily highlighting potential outliers. Statistical methods such as the Z-score (measuring how many standard deviations a data point is from the mean) can also be used to flag potential outliers.
Treating Outliers: Depending on the source of the outlier, different approaches can be considered. If an outlier is due to a measurement error, correcting the error (if possible) or removing the data point may be appropriate. However, removing outliers without proper justification can bias the results. In many cases, it's best to keep the outliers but use a robust measure of dispersion that is less affected by them.
Conclusion: The Importance of Context
The standard deviation is a valuable tool for understanding data variability, but its sensitivity to outliers is a crucial limitation. While it provides a clear and readily interpretable measure of dispersion, this measure should be carefully considered in the presence of outliers. Using robust alternatives such as the IQR or MAD, understanding the underlying causes of outliers, and employing appropriate visualization techniques are all essential steps to accurately characterize and interpret the spread of data, ensuring a reliable and robust statistical analysis. The decision of whether to use the standard deviation or a more robust measure ultimately depends on the specific context of the data analysis and the desired outcome. Always prioritize a critical assessment of the data and the appropriate statistical methods to ensure the accuracy and reliability of the conclusions drawn. Understanding the nuances of data dispersion and choosing the right statistical tools is key to conducting a successful data analysis.
Latest Posts
Latest Posts
-
How To Calculate Heat Of Dissolution
May 10, 2025
-
A Person With A Damaged Visual Association Area May Be
May 10, 2025
-
Complete The Ka1 Expression For H2co3 In An Aqueous Solution
May 10, 2025
-
How Many Chambers In A Frog Heart
May 10, 2025
-
Sodium Carbonate And Lead Ii Nitrate
May 10, 2025
Related Post
Thank you for visiting our website which covers about Is The Standard Deviation Resistant To Outliers . We hope the information provided has been useful to you. Feel free to contact us if you have any questions or need further assistance. See you next time and don't miss to bookmark.