Is The Mean Resistant To Outliers
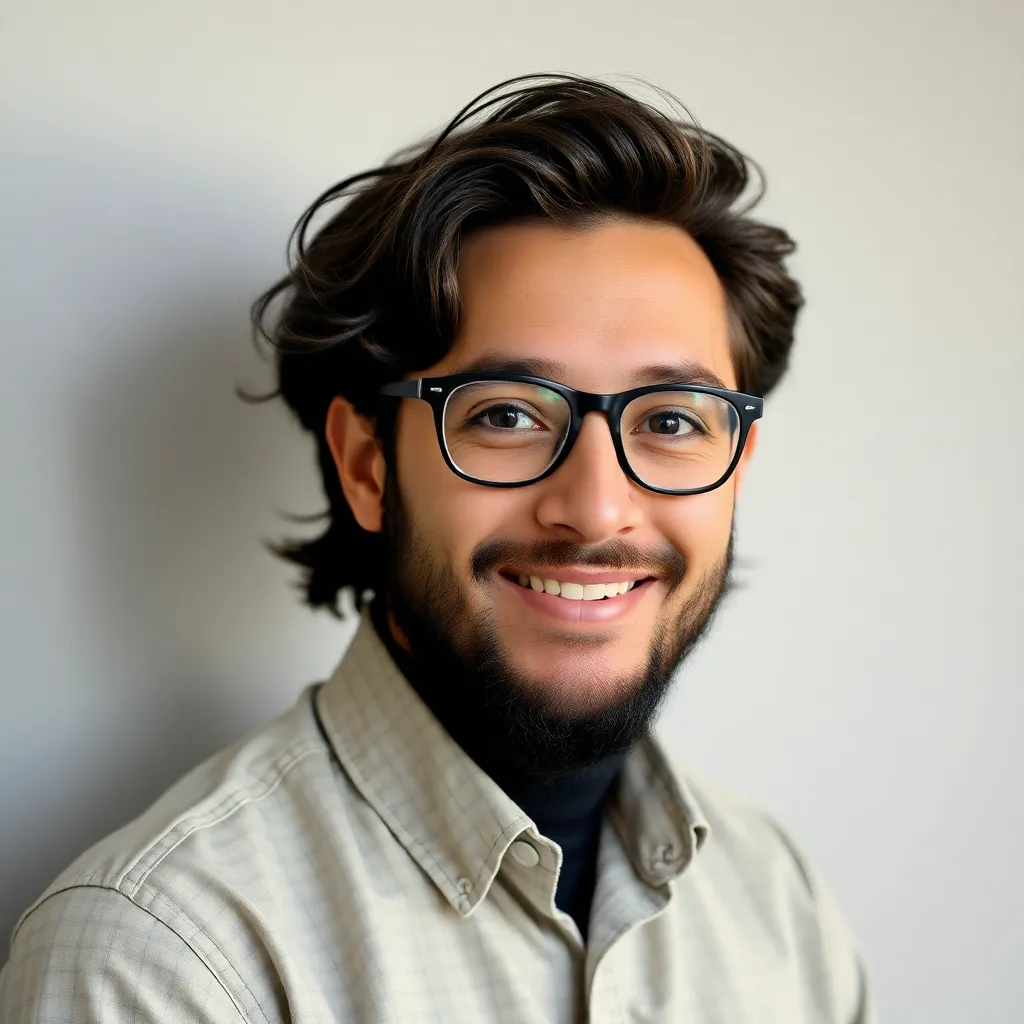
listenit
May 10, 2025 · 7 min read
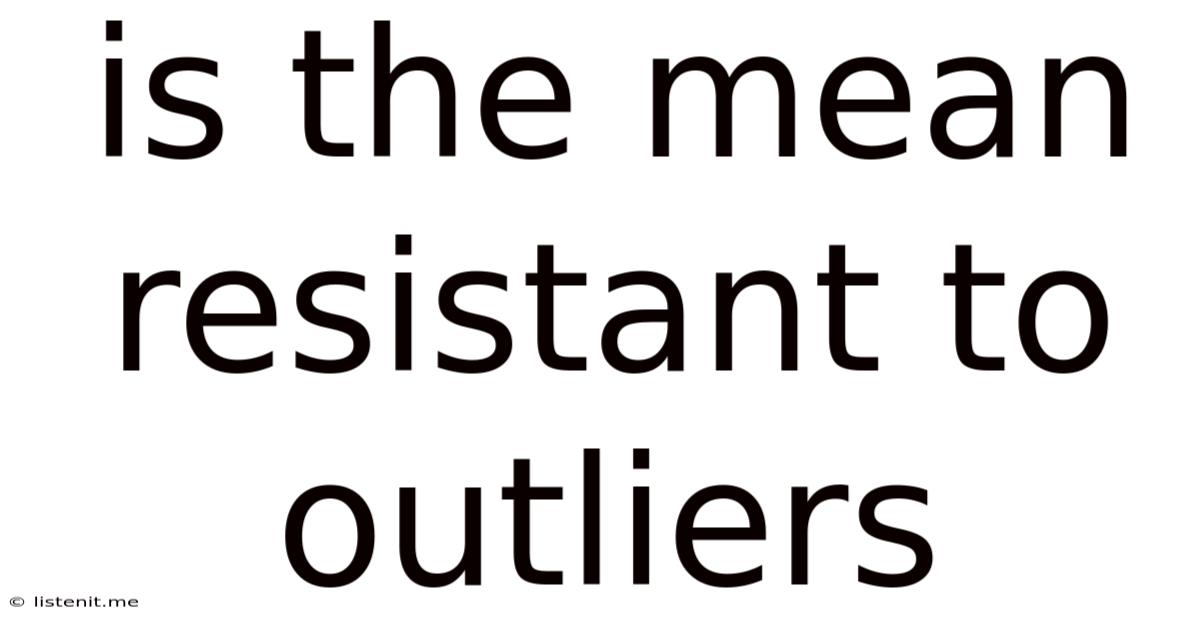
Table of Contents
Is the Mean Resistant to Outliers? A Deep Dive into Statistical Robustness
The mean, or average, is a cornerstone of descriptive statistics. We use it constantly – from calculating average grades to understanding national income distributions. But how robust is this seemingly simple measure? Specifically, is the mean resistant to outliers? The short answer is: no. This article delves into why, exploring the concept of robustness, alternative measures, and the implications for data analysis.
Understanding the Mean and Outliers
Before we tackle the core question, let's define our terms:
-
Mean: The arithmetic mean is calculated by summing all values in a dataset and dividing by the number of values. It represents the central tendency of the data.
-
Outliers: Outliers are data points that significantly deviate from the other observations in a dataset. They can be caused by errors in data collection, genuinely extreme values, or simply represent a different population altogether.
The mean's susceptibility to outliers stems directly from its calculation. Because every data point contributes directly to the sum, a single extreme value can disproportionately influence the result, pulling the mean away from the center of the majority of the data.
Why the Mean is Not Resistant to Outliers
Consider a simple example: a dataset representing the salaries of employees in a small company. Let's say the salaries are: $40,000, $42,000, $45,000, $48,000, and $50,000. The mean is $45,000, representing a reasonable average.
Now, let's introduce an outlier: the CEO's salary of $500,000. The new mean becomes $125,000. The addition of a single data point drastically altered the mean, making it a poor representation of the typical employee salary. This clearly demonstrates the mean's lack of resistance to outliers.
This sensitivity is further amplified in datasets with larger ranges and fewer observations. In such cases, even a moderately extreme value can significantly distort the mean.
Visualizing the Impact of Outliers
Visualizing data is crucial in understanding the influence of outliers. Histograms and box plots are particularly useful.
-
Histograms: A histogram displays the distribution of data. An outlier will appear as a separate bar far from the main cluster of data, clearly demonstrating its impact on the mean.
-
Box Plots: Box plots provide a concise summary of data, including quartiles and outliers. Outliers are typically displayed as individual points beyond the "whiskers," highlighting their separation from the main data distribution.
By visually inspecting the data distribution, we can quickly identify the presence of outliers and anticipate their effect on the mean.
Robust Alternatives to the Mean
Given the mean's vulnerability to outliers, statisticians have developed robust alternatives that are less sensitive to extreme values:
-
Median: The median is the middle value in a sorted dataset. It's far less influenced by outliers because only the relative position of data points matters, not their magnitude. In our salary example, the median remains relatively stable even with the CEO's inclusion, offering a more accurate representation of typical employee salaries.
-
Trimmed Mean: A trimmed mean is calculated by removing a certain percentage of the highest and lowest values from the dataset before calculating the mean. This effectively mitigates the influence of outliers by excluding them from the calculation. The percentage to trim is chosen based on the specific dataset and the level of outlier influence to be removed.
-
Winsorized Mean: Similar to the trimmed mean, the Winsorized mean replaces the extreme values with less extreme values before computing the mean. Instead of removing the extreme values, they are replaced by the highest or lowest value that is not considered an outlier. This preserves more information than trimming.
-
M-estimators: These are a broader class of robust estimators that use iterative methods to minimize a loss function that is less sensitive to outliers than the traditional squared error loss function used in calculating the mean. They provide a more sophisticated approach to handling outliers.
Choosing the Right Measure of Central Tendency
The decision of which measure of central tendency to use depends heavily on the specific data and research question.
-
Use the mean when: the data is normally distributed, or approximately normally distributed, and there are few or no outliers. The mean is useful for parametric statistical tests.
-
Use the median when: the data is skewed, contains outliers, or the distribution is unknown. The median is less sensitive to outliers and is often preferable for non-parametric tests.
-
Use the trimmed or Winsorized mean when: you want to retain some information from the extreme values but mitigate their extreme influence. These are useful when a compromise between the mean and median is desired.
-
Consider M-estimators when: you need a highly robust measure that can handle complex outlier patterns effectively.
Always visually inspect your data using histograms, box plots, or scatter plots to assess the presence and potential impact of outliers before selecting a measure of central tendency.
Implications for Data Analysis
The sensitivity of the mean to outliers has significant implications for various aspects of data analysis:
-
Descriptive Statistics: Reporting only the mean without considering outliers can lead to misleading conclusions about the central tendency of the data. Always report the median or other robust measures along with the mean, particularly when outliers are present.
-
Inferential Statistics: Many statistical tests assume normality and are sensitive to outliers. Outliers can inflate the variance, reducing the power of hypothesis tests and potentially leading to incorrect conclusions. Robust statistical methods, designed to be less sensitive to outliers, should be considered.
-
Data Cleaning and Preprocessing: Identifying and handling outliers is a crucial step in data preprocessing. Depending on the context, outliers might be removed, corrected, or transformed. However, removing data should always be justified, and careful consideration should be given to the potential loss of information.
Beyond the Mean: A Deeper Look at Robust Statistics
The issue of outlier resistance extends beyond the choice of central tendency. Many common statistical methods are susceptible to outliers. Robust statistics is a branch of statistics focused on developing methods that are less sensitive to outliers and deviations from assumptions like normality. These methods are crucial for obtaining reliable and meaningful results, especially when dealing with real-world datasets which are often messy and contain unexpected values.
Robust methods are not just about dealing with single outliers, but also with entire data subsets that differ systematically from the primary data. These might not even be easily identifiable as “outliers” in a classical sense. For example, consider a dataset measuring student performance. A group of students from a particular school consistently scores lower than other students due to differences in resources. These students, as a group, would not be identified as "outliers" by simple outlier detection methods, but they would substantially affect the mean score of all students. Robust methods can handle such systematic effects more effectively.
Understanding the limitations of traditional methods and embracing the power of robust statistics will lead to more reliable and meaningful insights from data analysis.
Conclusion: A Balanced Approach to Outliers
The mean, while a widely used measure, is not resistant to outliers. Its sensitivity can lead to misleading interpretations and inaccurate statistical inferences. Therefore, a balanced approach is necessary:
-
Visualize your data: Always inspect your data visually to identify potential outliers.
-
Consider robust alternatives: Use median, trimmed mean, Winsorized mean, or M-estimators as appropriate, depending on the nature of your data and research questions.
-
Understand the context: Investigate the source of outliers. Are they genuine extreme values, measurement errors, or representative of a different population? This understanding will guide the appropriate handling strategy.
-
Apply robust statistical methods: Utilize robust statistical methods throughout your analysis to mitigate the impact of outliers on your inferences.
By adopting this thoughtful approach, you can ensure that your data analysis is reliable, accurate, and provides meaningful insights, avoiding the pitfalls of outlier sensitivity. Remember, a critical eye and a flexible approach to data analysis are essential for extracting the true story from your data.
Latest Posts
Latest Posts
-
What Does 1 1 2 Cup Mean
May 10, 2025
-
Alignment Of Sun Moon And Earth
May 10, 2025
-
Complex Zeros Of A Polynomial Function
May 10, 2025
-
A B C Solve For C
May 10, 2025
-
Dna The Double Helix Worksheet Answers
May 10, 2025
Related Post
Thank you for visiting our website which covers about Is The Mean Resistant To Outliers . We hope the information provided has been useful to you. Feel free to contact us if you have any questions or need further assistance. See you next time and don't miss to bookmark.