Is Age A Discrete Or Continuous Variable
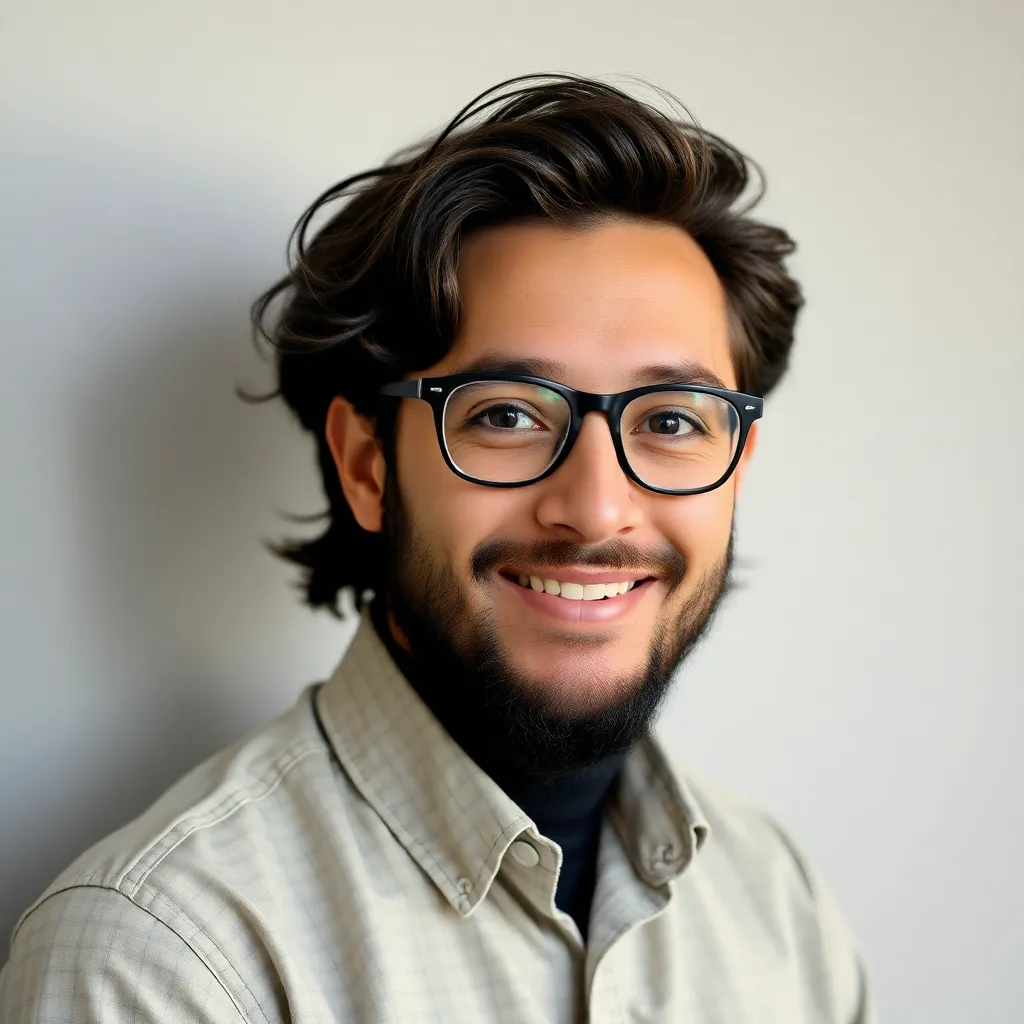
listenit
Apr 23, 2025 · 7 min read

Table of Contents
Is Age a Discrete or Continuous Variable? A Deep Dive into Measurement and Statistical Analysis
The question of whether age is a discrete or continuous variable is a surprisingly complex one, sparking debate among statisticians and researchers alike. While seemingly straightforward, the answer hinges on the level of precision and the context in which age is measured and analyzed. This article delves into the nuances of this debate, exploring the arguments for both sides and examining the implications for data analysis.
Understanding Discrete and Continuous Variables
Before tackling the age debate, let's clarify the definitions:
-
Discrete Variables: These variables can only take on a finite number of values or specific, separate values. They are usually whole numbers and often represent counts or categories. Examples include the number of cars in a parking lot, the number of students in a class, or the number of siblings a person has. You cannot have 2.5 cars or 1.7 siblings.
-
Continuous Variables: These variables can take on any value within a given range. They are often measured using scales and can have decimal places. Examples include height, weight, temperature, and time. A person's height could be 5.8 feet, 5.82 feet, or even 5.823 feet, depending on the precision of the measuring instrument.
The Case for Age as a Continuous Variable
The most common perspective treats age as a continuous variable. This stems from the understanding that age is constantly changing. Every second, minute, hour, and day, we are incrementally older. Theoretically, age could be measured with extreme precision, down to fractions of a second. This characteristic aligns with the definition of a continuous variable, where values can fall anywhere within a range. For instance, a person could be 25.37 years old, accounting for months and days.
This perspective is particularly relevant in:
-
Longitudinal Studies: Tracking changes over time requires the precision offered by considering age as continuous. Analyzing growth curves, for example, relies on the continuous nature of age to capture subtle variations.
-
Survival Analysis: In studies assessing the lifespan of individuals or objects, the exact age at a specific event (e.g., death, equipment failure) is crucial. Treating age as continuous offers greater accuracy.
-
Growth Modeling: Modeling biological or economic growth often uses age as a predictor. The continuous nature of age allows for more accurate predictions of growth trajectories.
-
Medical Research: Precise age is often a critical factor in medical studies, where even slight variations in age can impact responses to treatments or the likelihood of developing certain diseases. Consider the difference in responses between 25-year-olds and 25.5-year-olds in a drug trial. While small, it could potentially influence research results.
Challenges to the Continuous Perspective
While compelling, the continuous view of age presents some challenges. The precision required to treat age as truly continuous is often impractical. We typically record age in years, sometimes including months and days, but rarely delve into hours, minutes, and seconds. The level of detail may be unnecessary for most applications and introduces considerable measurement error.
The practical limitations of measurement mean that even if we consider age inherently continuous, we frequently analyze it as a discretized version in real-world applications.
The Case for Age as a Discrete Variable
The argument for considering age as a discrete variable arises primarily from the way we typically measure and categorize it. We usually record age in whole numbers (years), sometimes adding months or even days. This implies a level of grouping and discretization.
-
Age as a Categorical Variable: Age is often treated as a categorical variable for analysis, grouped into age brackets (e.g., 0-18, 19-35, 36-50, etc.). These broad categories inherently treat age as discrete.
-
Developmental Stages: In fields like psychology and education, age is used to define developmental stages (e.g., infancy, childhood, adolescence, adulthood). These stages represent distinct qualitative changes, reinforcing the discrete nature of age in these contexts.
-
Administrative Data: In administrative data such as census data, age is typically recorded in whole years or specific age groups. This directly reflects a discretization of the underlying continuous process of aging.
Limitations of the Discrete Perspective
Treating age strictly as a discrete variable ignores the continuous progression of aging between whole-number years. This can lead to a loss of information and potentially inaccurate analyses, especially when dealing with:
-
Detailed Longitudinal Data: When tracking changes across very short time periods, the discrete nature of age groups can obscure important variations.
-
Event History Analysis: When the exact age at an event is crucial, simply grouping individuals into age categories can lead to substantial bias.
-
Growth and Development: Considering age as strictly discrete ignores the continuous process of growth and development, and this could misrepresent dynamic processes.
Reconciling the Discrepancy: The Role of Measurement and Context
The seemingly contradictory views of age as discrete or continuous stem from the difference between the underlying process of aging and how we practically measure and analyze it. Aging itself is a continuous process, but the way we record and categorize age often results in discrete representations.
The most appropriate approach depends heavily on the context and the goals of the analysis:
-
High Precision is Needed: When high precision is needed, and the data allows for it, treat age as continuous. Use the most granular measurement available and apply appropriate statistical techniques.
-
Categorical Analysis is Sufficient: When examining broader trends or comparisons across age groups, treating age as a categorical or discrete variable is often acceptable. In this situation, careful consideration of age group definitions is important.
-
Mixed Methods Approach: A combination of continuous and discrete representations might be necessary. For instance, you might analyze a continuous age variable to model trends and then group individuals into categories for reporting or comparison.
Implications for Statistical Analysis
The choice between treating age as discrete or continuous has significant consequences for the statistical methods used:
-
Continuous Age: Suitable methods include regression analysis (linear, polynomial), survival analysis (Kaplan-Meier curves, Cox proportional hazards models), and growth curve modeling. These techniques leverage the full range of age values.
-
Discrete Age: Appropriate techniques might involve ANOVA, chi-square tests, or logistic regression, depending on the nature of the dependent variable and the research question. These methods compare groups defined by discrete age categories.
Choosing the Right Approach: A Practical Guide
Here's a step-by-step guide to help determine the appropriate treatment of age in your analysis:
-
Define your research question: What are you trying to understand or predict? The research question will guide your choice of statistical method and thus, how you handle age.
-
Assess your data: What is the level of precision in your age measurements? Do you have age in years, years and months, or even more detailed measurements?
-
Consider the nature of your variables: Are you comparing groups based on age? Or are you examining relationships between age and other continuous variables?
-
Select appropriate statistical methods: Based on the above considerations, choose statistical methods suitable for your chosen treatment of age (continuous or discrete).
-
Interpret your results carefully: Remember that treating age as discrete can lead to some loss of information, which needs to be considered when interpreting your findings.
Conclusion
The question of whether age is discrete or continuous highlights the interplay between theoretical concepts and practical limitations in data analysis. While aging is fundamentally a continuous process, we often encounter age data in a discretized form. Understanding this duality and carefully selecting the appropriate statistical methods is crucial for sound research and accurate interpretation of results. The best approach hinges on the specific research question, the level of detail in the data, and the requirements of the chosen statistical methods. By carefully considering these factors, researchers can ensure that age is treated appropriately in their analyses, leading to more accurate and insightful results.
Latest Posts
Latest Posts
-
Lipids Cannot Be Considered Polymers Because
Apr 23, 2025
-
What Stage Do Cells Spend Most Of Their Lives In
Apr 23, 2025
-
How To Calculate Density Of Cube
Apr 23, 2025
-
What Is The Square Root Of 1024
Apr 23, 2025
-
32 To The Power Of 2
Apr 23, 2025
Related Post
Thank you for visiting our website which covers about Is Age A Discrete Or Continuous Variable . We hope the information provided has been useful to you. Feel free to contact us if you have any questions or need further assistance. See you next time and don't miss to bookmark.