Influencing Factors Of Degradation Correlation Plot
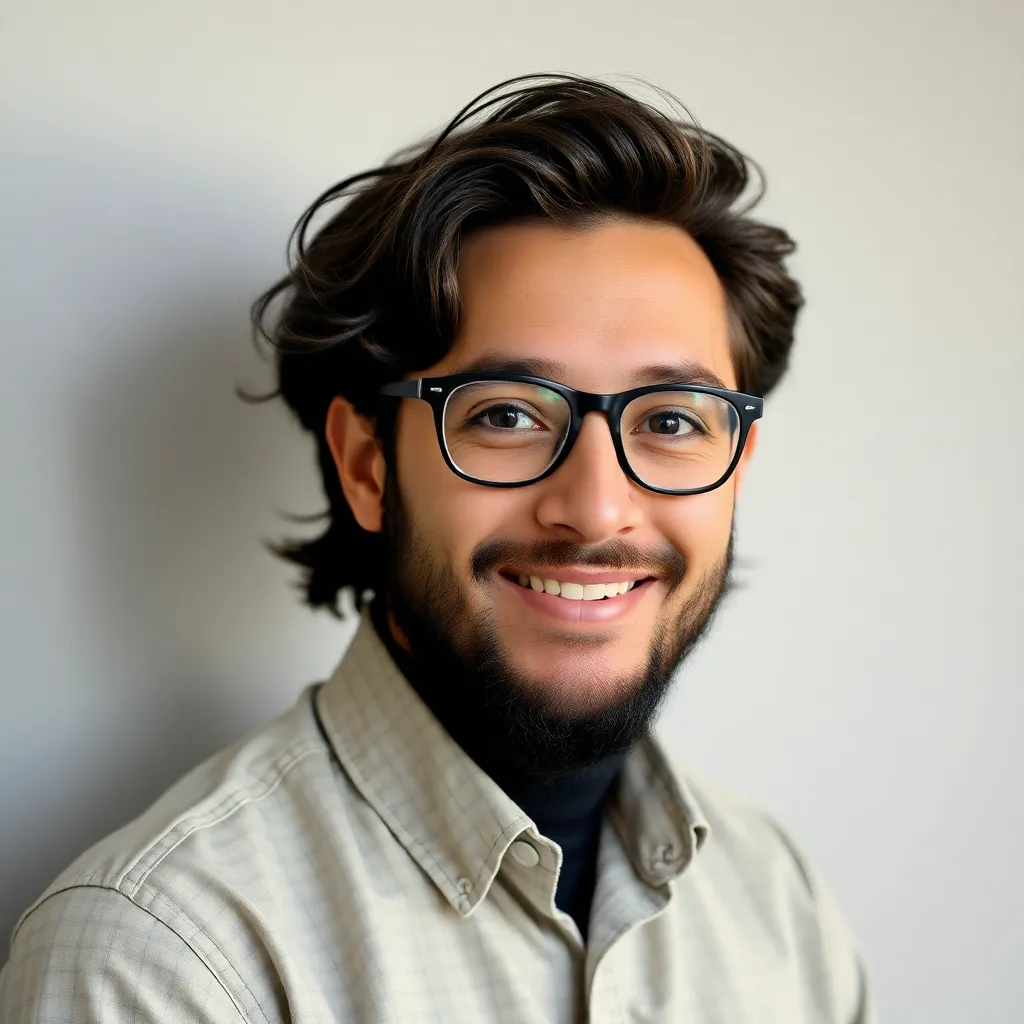
listenit
May 27, 2025 · 7 min read
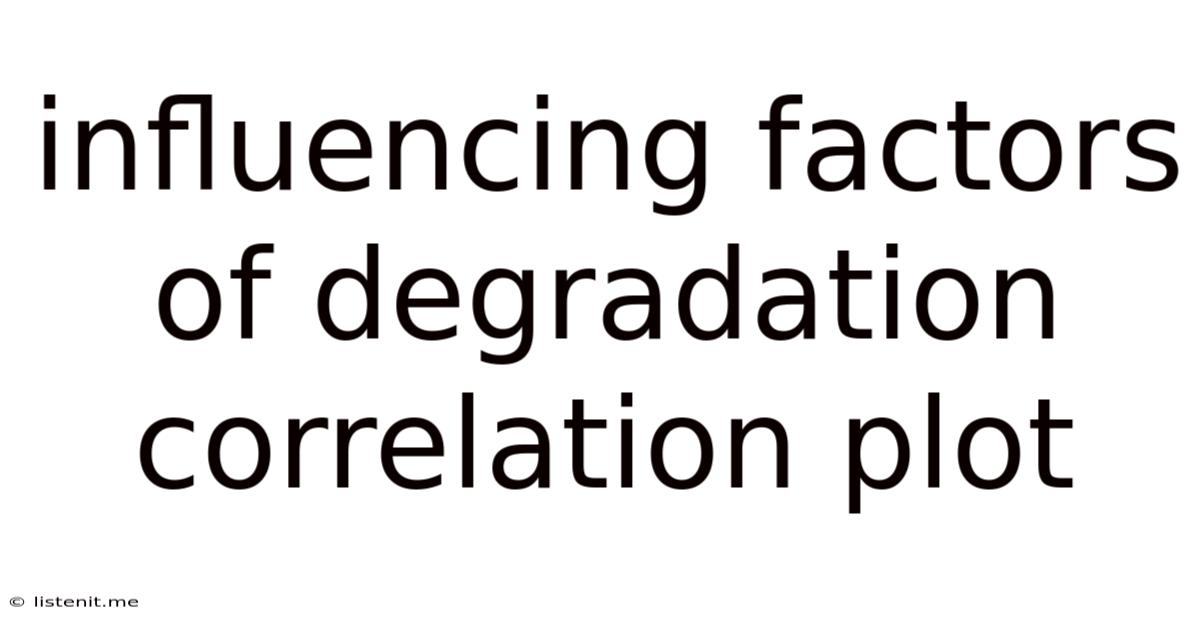
Table of Contents
Influencing Factors of Degradation Correlation Plot: A Comprehensive Guide
Degradation correlation plots are powerful visual tools used to understand the relationships between different degradation mechanisms in complex systems. These plots help identify which factors contribute most significantly to overall degradation and how these factors interact. However, the effectiveness of a degradation correlation plot hinges on several influencing factors, ranging from data quality to the choice of analysis methods. Understanding these factors is crucial for generating meaningful and accurate insights. This comprehensive guide explores the key influences on degradation correlation plots, providing a detailed understanding for researchers and engineers alike.
I. Data Quality: The Foundation of Accurate Plots
The quality of the input data is arguably the most significant influencing factor. Garbage in, garbage out – this adage applies perfectly to degradation correlation plots. Several aspects of data quality directly impact the reliability and interpretability of the plot:
A. Data Completeness: Missing Data and its Implications
Incomplete datasets are a common challenge. Missing data points can introduce bias and lead to inaccurate estimations of correlations. Strategies for handling missing data include imputation techniques (e.g., mean imputation, k-nearest neighbors) or using specialized statistical methods designed for incomplete datasets. However, it's crucial to acknowledge the limitations of any imputation method and its potential impact on the results. Transparency about data handling is essential for responsible interpretation.
B. Data Accuracy and Precision: Errors and Noise
Measurement errors and noise in the data can significantly distort the correlation patterns observed in the plot. Accurate and precise measurements are crucial. This involves careful calibration of instruments, well-defined measurement protocols, and potentially the use of multiple measurement techniques to improve data validation. Analyzing the sources of error and quantifying their uncertainty can help assess the robustness of the correlations identified.
C. Data Representation: Choosing the Right Variables
The choice of degradation variables is critical. The selected variables should directly reflect the degradation mechanisms of interest. Using inappropriate variables can mask true correlations or produce spurious ones. For instance, if analyzing the degradation of a material, selecting relevant properties like hardness, tensile strength, or surface roughness, instead of less directly related properties, is crucial for obtaining meaningful results. Careful consideration of the system and the degradation processes is essential.
D. Data Transformation: Enhancing Correlation Visibility
Raw data may not always reveal clear correlation patterns. Transforming the data, such as applying logarithmic or square root transformations, can sometimes improve the linearity of relationships and make correlations more apparent. The choice of transformation should be guided by the data distribution and the nature of the degradation mechanisms. For example, if the data exhibits a skewed distribution, a logarithmic transformation might help normalize it.
II. Statistical Methods: Selecting Appropriate Approaches
The choice of statistical method used to generate the correlation plot significantly influences the results. Several methods exist, each with its own strengths and weaknesses:
A. Pearson Correlation Coefficient: Linear Relationships
The Pearson correlation coefficient is commonly used to quantify the linear association between two variables. It ranges from -1 (perfect negative correlation) to +1 (perfect positive correlation), with 0 indicating no linear correlation. However, it's crucial to remember that a Pearson correlation of 0 does not imply the absence of any relationship – it only indicates the absence of a linear relationship. Non-linear relationships may exist, even with a Pearson correlation close to zero.
B. Spearman Rank Correlation Coefficient: Non-linear Relationships
The Spearman rank correlation coefficient measures the monotonic relationship between two variables. This means it can detect non-linear relationships as long as the relationship is consistently increasing or decreasing. It's less sensitive to outliers than the Pearson correlation coefficient. The choice between Pearson and Spearman depends on the nature of the expected relationship between the degradation variables.
C. Other Correlation Measures: Addressing Specific Needs
Other correlation measures, such as Kendall's tau or distance correlation, may be more appropriate in specific situations. Kendall's tau is another rank-based correlation coefficient, often preferred when dealing with ordinal data or when the data contains many ties. Distance correlation is a more general measure that can detect both linear and non-linear associations, even in high-dimensional data. Selecting the most appropriate method requires understanding the limitations and assumptions of each technique.
III. Visual Representation: Enhancing Interpretability
The way the correlation plot is visually represented greatly affects how easily the results can be understood and interpreted.
A. Scatter Plots: Visualizing Bivariate Relationships
Scatter plots are the most common way to visualize the correlation between two variables. They provide a clear visual representation of the relationship's strength and direction. The use of color-coding or different symbols can further enhance the plot's interpretability by highlighting additional factors or grouping data points.
B. Heatmaps: Representing Multiple Correlations Simultaneously
Heatmaps are useful for visualizing correlations among multiple variables simultaneously. They display a matrix of correlation coefficients, with colors representing the strength and direction of the correlation. Heatmaps are particularly useful when dealing with a large number of variables, allowing for a quick overview of complex relationships.
C. Network Graphs: Visualizing Complex Interactions
Network graphs provide a visual representation of the relationships between multiple variables as a network, with nodes representing variables and edges representing correlations. The thickness or color of the edges can represent the strength of the correlation. Network graphs are especially useful for revealing intricate interactions among several degradation mechanisms.
IV. External Factors: Contextual Influences
Several external factors can significantly influence the interpretation of a degradation correlation plot:
A. Environmental Conditions: External Impacts
Environmental conditions, such as temperature, humidity, or exposure to chemicals, can influence the degradation process and thus affect the observed correlations. Understanding and controlling for these environmental factors are crucial for obtaining reliable results. Data should ideally be collected under controlled conditions or appropriately adjusted for environmental variations.
B. Operational Conditions: Usage Impacts
Operational conditions, such as load, stress, or frequency of use, also influence degradation. These factors must be accounted for in the analysis. The correlation patterns observed might be significantly different depending on the operating conditions. Therefore, stratifying the data based on operational conditions can reveal more nuanced insights.
C. Material Properties and Manufacturing Processes: Intrinsic Influences
The inherent properties of the material and the manufacturing processes used can influence the degradation behavior. Differences in material composition, microstructure, or surface finish can lead to varied degradation rates and different correlation patterns. Considering these inherent material properties is critical for a complete understanding.
V. Advanced Techniques: Enhanced Insights
Several advanced techniques can enhance the insights gained from degradation correlation plots:
A. Regression Analysis: Quantifying Relationships
Regression analysis can quantify the relationships between degradation variables and predict future degradation based on the observed correlations. Different regression models (linear, non-linear, etc.) can be used depending on the nature of the relationships. Regression models provide a more quantitative understanding of the interplay between the different factors influencing degradation.
B. Principal Component Analysis (PCA): Dimensionality Reduction
PCA is a useful dimensionality reduction technique for handling data with a large number of variables. It transforms the original variables into a smaller set of uncorrelated principal components, which capture the major sources of variation in the data. This simplifies the analysis and visualization of correlations while still retaining most of the relevant information.
C. Machine Learning: Advanced Predictive Modeling
Machine learning techniques, such as neural networks or support vector machines, can be used for advanced predictive modeling of degradation based on the observed correlations. These techniques are particularly useful when dealing with complex, non-linear relationships that are difficult to model using traditional statistical methods.
VI. Conclusion: A Holistic Approach to Degradation Correlation Plots
Generating meaningful degradation correlation plots requires a holistic approach that considers various influencing factors, from data quality to advanced statistical techniques. Careful attention to data collection, appropriate statistical methods, effective visualization, and the inclusion of contextual information are critical for obtaining accurate and insightful results. By understanding and addressing these factors, researchers and engineers can effectively utilize degradation correlation plots to gain a deeper understanding of complex degradation mechanisms and ultimately improve the reliability and lifespan of various systems. Furthermore, incorporating advanced analytical techniques can provide even more sophisticated insights into the intricate relationships underlying degradation processes, ultimately leading to more efficient preventative maintenance strategies and enhanced product design. Remember that transparency in methodology and data handling is crucial for the reliable interpretation of the results, enabling informed decisions and fostering trust in the findings.
Latest Posts
Latest Posts
-
Receptors That Bind Norepinephrine And Epinephrine Are Known As
May 29, 2025
-
Why Not To Give Albuterol To Chf
May 29, 2025
-
How Do Cultural Services Help Humans
May 29, 2025
-
Nutrition Is The Science That Investigates
May 29, 2025
-
Accumulation Of Pollutants At Successive Levels Of The Food Chain
May 29, 2025
Related Post
Thank you for visiting our website which covers about Influencing Factors Of Degradation Correlation Plot . We hope the information provided has been useful to you. Feel free to contact us if you have any questions or need further assistance. See you next time and don't miss to bookmark.