How To Find A Point Estimate
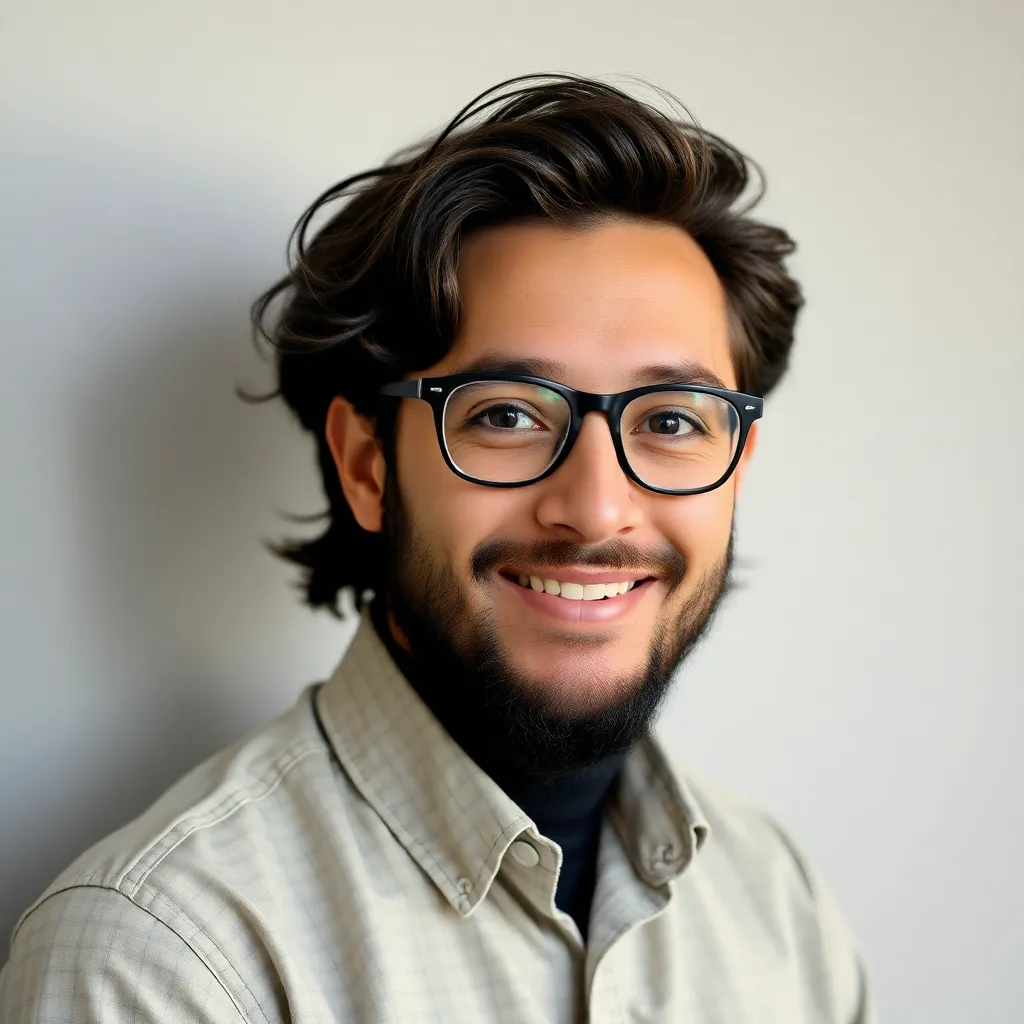
listenit
May 09, 2025 · 6 min read

Table of Contents
How to Find a Point Estimate: A Comprehensive Guide
Finding a point estimate is a crucial step in statistical inference. It allows us to summarize a large dataset with a single value, providing a concise representation of a population parameter. This guide will comprehensively explore the various methods and considerations involved in finding accurate and reliable point estimates. We will cover different types of estimates, their underlying assumptions, and practical applications.
Understanding Point Estimates
A point estimate is a single value used to approximate a population parameter. This parameter could be the population mean (μ), population standard deviation (σ), population proportion (p), or any other characteristic of the population we are interested in. Instead of analyzing the entire population (which is often impractical or impossible), we draw a sample and use the sample data to calculate the point estimate.
For example, if we want to know the average height of all women in a country, we wouldn't measure every woman. Instead, we would take a sample of women, measure their heights, and calculate the average height of the sample. This average height would be our point estimate for the population mean height.
The key difference between a point estimate and an interval estimate (like a confidence interval) is that a point estimate is a single value, while an interval estimate provides a range of plausible values.
Methods for Finding Point Estimates
The method for finding a point estimate depends on the population parameter you're trying to estimate and the type of data you have. Here are some common methods:
1. Estimating the Population Mean (μ)
The most common way to estimate the population mean is by using the sample mean (x̄). The sample mean is calculated by summing all the values in the sample and dividing by the number of observations (n).
Formula: x̄ = Σxᵢ / n
where:
- x̄ is the sample mean
- Σxᵢ is the sum of all values in the sample
- n is the sample size
Example: Suppose we have a sample of five students' test scores: 85, 92, 78, 88, and 95. The sample mean would be (85 + 92 + 78 + 88 + 95) / 5 = 87.6. Therefore, our point estimate for the population mean test score is 87.6.
2. Estimating the Population Proportion (p)
The population proportion represents the proportion of individuals in a population possessing a specific characteristic. The point estimate for the population proportion is the sample proportion (p̂).
Formula: p̂ = x / n
where:
- p̂ is the sample proportion
- x is the number of individuals in the sample with the specific characteristic
- n is the sample size
Example: Suppose we survey 100 people and find that 30 of them own a pet. The sample proportion is 30/100 = 0.3. Our point estimate for the population proportion of pet owners is 0.3 or 30%.
3. Estimating the Population Variance (σ²) and Standard Deviation (σ)
Estimating the population variance and standard deviation involves using the sample variance (s²) and sample standard deviation (s).
Formula for Sample Variance: s² = Σ(xᵢ - x̄)² / (n - 1)
Formula for Sample Standard Deviation: s = √s²
where:
- s² is the sample variance
- s is the sample standard deviation
- xᵢ is each individual value in the sample
- x̄ is the sample mean
- n is the sample size
The use of (n-1) in the denominator of the sample variance is called Bessel's correction. It provides an unbiased estimate of the population variance.
4. Estimating the Population Median
The population median is the middle value when the data is ordered. The point estimate for the population median is the sample median. To find the sample median, arrange the data in ascending order. If the sample size is odd, the median is the middle value. If the sample size is even, the median is the average of the two middle values.
5. Estimating Other Parameters
Depending on the specific population parameter of interest, other methods might be needed. For example, estimating parameters for specific probability distributions (like the Poisson distribution or exponential distribution) often requires specialized techniques like maximum likelihood estimation (MLE).
Considerations when Finding Point Estimates
Several factors influence the accuracy and reliability of point estimates:
-
Sample Size: Larger sample sizes generally lead to more accurate point estimates because they reduce sampling error. Sampling error refers to the difference between the sample statistic and the true population parameter.
-
Sampling Method: The method used to select the sample significantly impacts the reliability of the estimate. Random sampling is crucial for ensuring that the sample is representative of the population and minimizes bias.
-
Data Quality: The accuracy of the point estimate depends on the accuracy and reliability of the data collected. Outliers or errors in the data can substantially distort the estimate.
-
Bias: Bias in the sampling method or data collection can lead to point estimates that systematically overestimate or underestimate the true population parameter.
-
Estimator Properties: Different estimators possess different properties. An ideal estimator should be unbiased (its expected value equals the population parameter), consistent (converges to the true parameter as the sample size increases), and efficient (has the smallest variance among all unbiased estimators).
Interpreting Point Estimates
Point estimates are valuable summaries of data, but it's critical to remember that they are only estimates. They are not the exact values of the population parameters. The true population parameter is likely to differ from the point estimate due to sampling variability.
Therefore, it's essential to consider the confidence interval associated with the point estimate, as it provides a range of plausible values for the parameter. The point estimate is the center of this interval. While a point estimate gives a single best guess for the parameter, it is the confidence interval that reveals the uncertainty associated with this guess.
Advanced Techniques for Point Estimation
While the methods described above are commonly used, more advanced techniques exist for point estimation, particularly in complex situations:
-
Maximum Likelihood Estimation (MLE): This method finds the parameter values that maximize the likelihood function, which represents the probability of observing the sample data given the parameters. MLE is widely used in various statistical models.
-
Method of Moments: This method equates sample moments (like the sample mean and variance) to the corresponding population moments to estimate the parameters.
-
Bayesian Estimation: This approach incorporates prior knowledge about the parameters into the estimation process, combining prior beliefs with the observed data to obtain a posterior estimate.
Conclusion
Finding a point estimate is a fundamental aspect of statistical analysis. By understanding the different methods and considerations involved, researchers and analysts can make informed decisions based on data. Remember that point estimates provide valuable summaries but should always be interpreted within the context of their uncertainty, ideally accompanied by measures of variability like confidence intervals. The choice of method depends heavily on the context of the problem, the type of data, and the goals of the analysis. Always strive for robust sampling methods and thorough data quality checks to ensure the reliability of your point estimates.
Latest Posts
Latest Posts
-
Which Of The Following Em Waves Has The Highest Frequency
May 09, 2025
-
A Square That Is Not A Parallelogram
May 09, 2025
-
How Do You Write 25 As A Decimal
May 09, 2025
-
How To Find The Value Of X In Geometry
May 09, 2025
-
What Is The Bond Angle Of H2o
May 09, 2025
Related Post
Thank you for visiting our website which covers about How To Find A Point Estimate . We hope the information provided has been useful to you. Feel free to contact us if you have any questions or need further assistance. See you next time and don't miss to bookmark.