How Many Independent Variables Should There Be In An Experiment
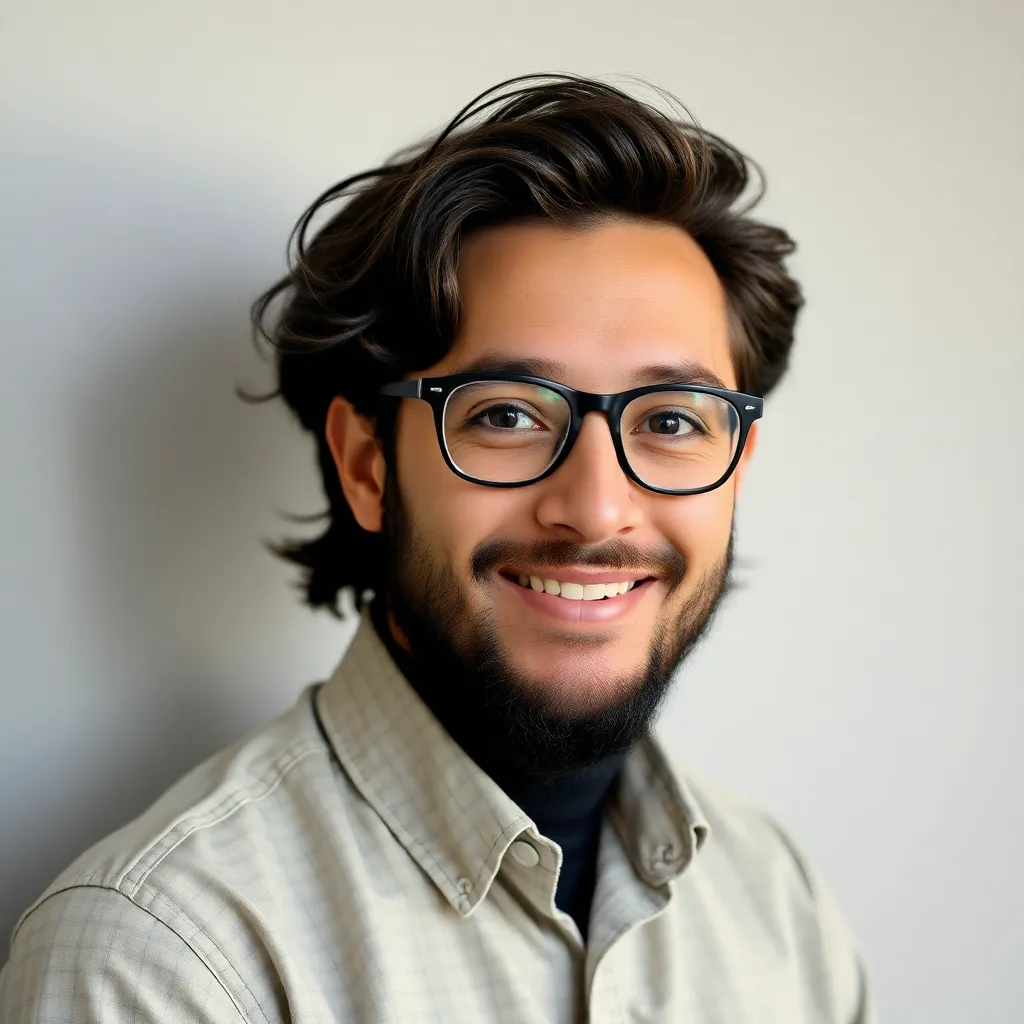
listenit
Apr 04, 2025 · 6 min read
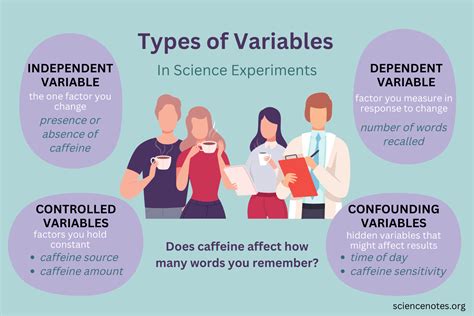
Table of Contents
How Many Independent Variables Should There Be in an Experiment? A Guide to Optimal Experimental Design
Determining the optimal number of independent variables in your experiment is crucial for achieving meaningful results and avoiding the pitfalls of overly complex or underpowered studies. There's no magic number; the ideal count depends on several interconnected factors, including your research question, resources, and the complexity of the phenomenon you're investigating. This comprehensive guide will delve into the considerations involved in deciding how many independent variables to include in your experimental design.
Understanding Independent Variables
Before diving into the optimal number, let's clarify what independent variables (IVs) are. In an experiment, independent variables are the factors that are manipulated or changed by the researcher to observe their effect on the dependent variable (DV). The DV is the outcome or response being measured. For instance, in a study examining the effect of fertilizer type and watering frequency on plant growth, the fertilizer type and watering frequency are the IVs, and plant growth is the DV.
Factors Influencing the Number of Independent Variables
The decision of how many IVs to include is a delicate balance. Too few might not capture the nuances of the phenomenon, while too many can lead to:
- Increased Complexity: Managing multiple IVs significantly increases the complexity of the experiment, making data analysis more challenging and potentially increasing the risk of errors.
- Resource Constraints: More IVs often necessitate more participants, more materials, and more time, leading to increased costs and logistical hurdles.
- Diminishing Returns: Beyond a certain point, adding more IVs might not yield proportionally significant increases in knowledge or understanding. The marginal gain in information might not justify the extra effort and expense.
- Type I and Type II Errors: An overabundance of IVs can inflate the probability of Type I errors (false positives) due to multiple comparisons. Conversely, underpowered studies with too few participants can increase the risk of Type II errors (false negatives).
The Case for Fewer Independent Variables: Simplicity and Power
Starting with fewer IVs (often just one or two) offers several significant advantages:
- Clearer Interpretation: Simpler experiments allow for easier interpretation of results. The impact of each IV on the DV is more readily apparent without the confounding effects of multiple interacting variables.
- Higher Statistical Power: Focusing on a smaller number of IVs allows for a more concentrated allocation of resources, leading to higher statistical power. This enhances the likelihood of detecting true effects if they exist.
- Reduced Complexity: Data analysis becomes significantly less complex, making it easier to identify significant findings and draw robust conclusions.
- Greater Control: With fewer IVs, it's easier to tightly control extraneous variables that might confound the results. This enhances the internal validity of the study.
Example: Focusing on a Single IV
Imagine a study examining the effect of different teaching methods on student performance. Instead of including numerous teaching methods, starting with a comparison between two distinct approaches (e.g., traditional lecture vs. active learning) can provide a clearer and more powerful initial understanding of the effect. Subsequent studies can then build upon these findings by introducing additional IVs.
The Case for More Independent Variables: Exploring Interactions and Complexity
While simplicity has its advantages, ignoring potentially crucial interactions between variables can lead to an incomplete understanding of the phenomenon. Including more IVs can be justifiable when:
- Investigating Interactions: When there's a theoretical reason to suspect interactions between variables, including multiple IVs is essential to uncover these relationships. Understanding how IVs interact can lead to more nuanced and comprehensive insights.
- Exploring a Complex Phenomenon: Some phenomena are inherently complex and require the inclusion of multiple IVs to capture their multifaceted nature. For instance, studying the effectiveness of a new drug might necessitate considering factors like dosage, age, and gender.
- Building on Previous Research: If previous research has identified important factors, incorporating these into a new study can help refine understanding and address limitations of past work.
Example: Exploring Interactions between IVs
In our teaching methods example, adding another IV such as student learning style (visual, auditory, kinesthetic) would allow us to investigate whether the effectiveness of different teaching methods varies depending on the learning style of the students. This interaction effect could significantly enrich the findings.
Finding the Balance: A Practical Approach
The optimal number of independent variables isn't a fixed number; it's a decision guided by careful consideration of several factors:
-
Research Question: The complexity of your research question directly influences the number of IVs needed. A focused question might only require one or two IVs, whereas a broader investigation may necessitate more.
-
Theoretical Framework: A well-developed theoretical framework can guide the selection of IVs and suggest potentially important interactions to investigate.
-
Resources: Your available resources (time, budget, participants) will naturally constrain the number of IVs you can practically include.
-
Statistical Power Analysis: Conducting a power analysis before the study is crucial. This analysis helps determine the minimum sample size needed to detect statistically significant effects with a desired level of power, considering the number of IVs included.
-
Factorial Design: Consider using factorial designs, which allow you to efficiently examine the effects of multiple IVs and their interactions. However, factorial designs become increasingly complex and resource-intensive as the number of IVs increases.
-
Hierarchical Approach: A hierarchical approach can be beneficial, starting with a simpler experiment focusing on a few key IVs and then progressively adding more IVs in subsequent studies. This incremental approach allows for a more manageable and iterative research process.
Practical Guidelines and Recommendations
-
Start Simple: Begin with the fewest number of IVs necessary to address your research question. Adding more IVs later is always possible.
-
Prioritize Key Variables: Focus on the most critical and theoretically relevant variables first.
-
Consider Interactions: If you have reason to suspect interactions between variables, include them in your design.
-
Conduct a Power Analysis: This is essential to ensure that your study has sufficient statistical power to detect meaningful effects given the number of IVs.
-
Manage Complexity: Use appropriate statistical techniques (e.g., ANOVA, multiple regression) to analyze data with multiple IVs.
-
Interpret Results Cautiously: With more IVs, the risk of spurious correlations increases. Carefully interpret the results and avoid drawing unwarranted conclusions.
Conclusion
The number of independent variables in an experiment is a critical decision that profoundly affects the study's feasibility, interpretability, and power. There is no universally "correct" number; the optimal count is determined by a nuanced consideration of the research question, theoretical framework, available resources, and statistical considerations. By thoughtfully balancing simplicity with the need for comprehensive understanding, researchers can design experiments that yield valuable and meaningful insights. Prioritizing clarity, utilizing appropriate statistical techniques, and carefully interpreting the findings are paramount to deriving meaningful conclusions from studies involving multiple independent variables. Remember, a well-designed experiment with fewer, carefully chosen IVs is often more powerful and insightful than a complex experiment overwhelmed by an excessive number of variables.
Latest Posts
Latest Posts
-
Why Fossils Found In Sedimentary Rocks
Apr 04, 2025
-
Simplify The Square Root Of 27
Apr 04, 2025
-
22 Is What Percent Of 55
Apr 04, 2025
-
1 Degree Celsius Equals How Many Fahrenheit
Apr 04, 2025
-
How Many Combinatos Of 5 Letters
Apr 04, 2025
Related Post
Thank you for visiting our website which covers about How Many Independent Variables Should There Be In An Experiment . We hope the information provided has been useful to you. Feel free to contact us if you have any questions or need further assistance. See you next time and don't miss to bookmark.