How Many Independent Variables Can You Have In An Experiment
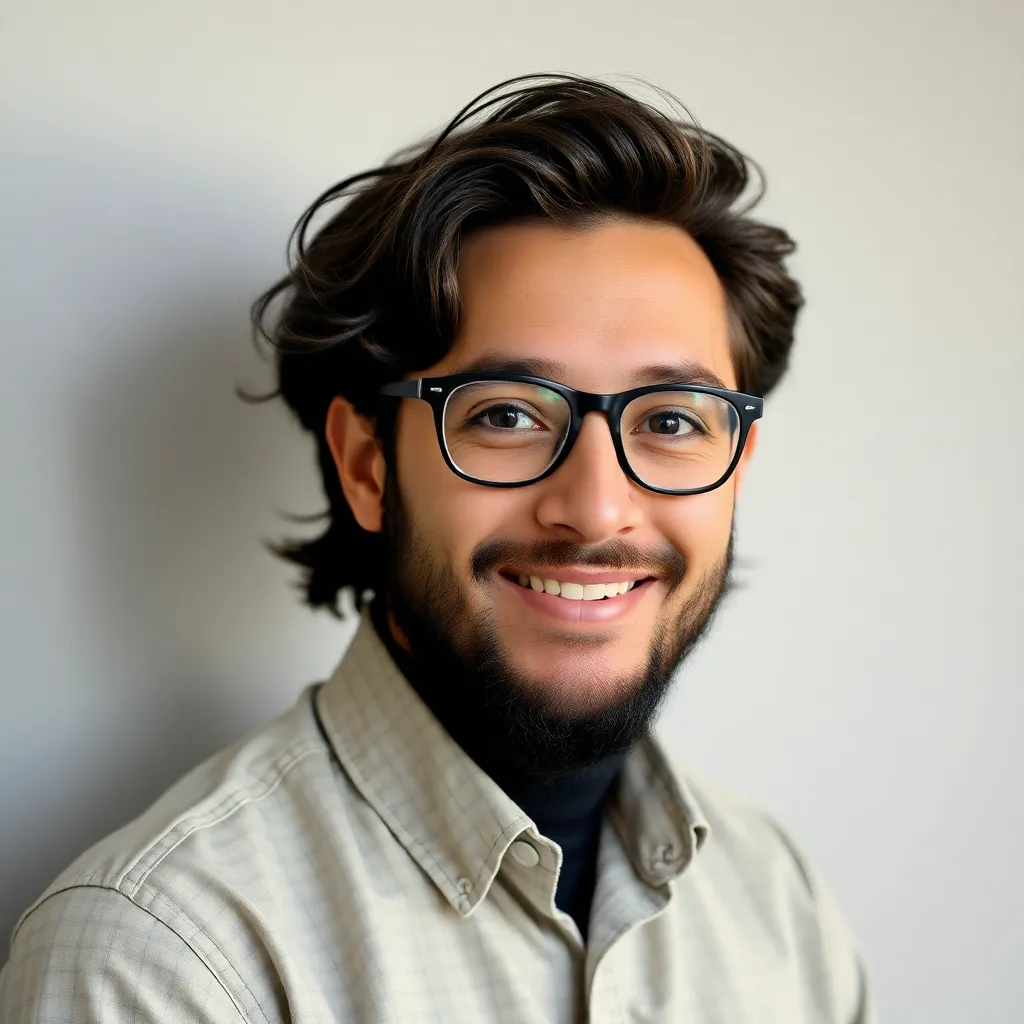
listenit
May 10, 2025 · 6 min read
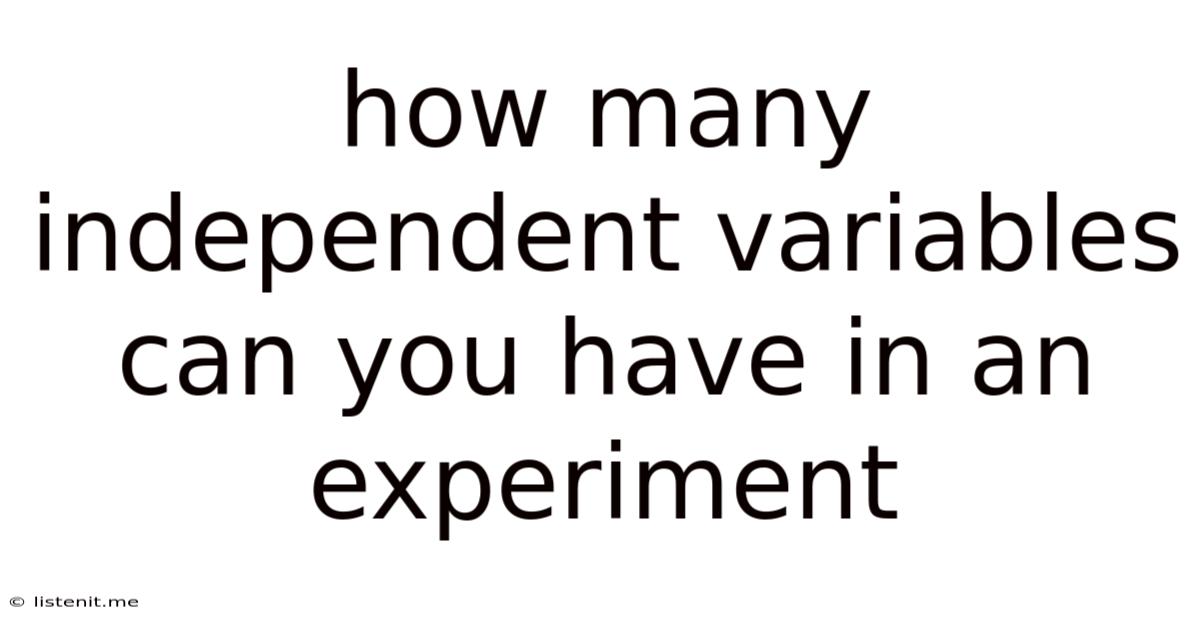
Table of Contents
How Many Independent Variables Can You Have in an Experiment? A Deep Dive into Experimental Design
The question of how many independent variables (IVs) an experiment can accommodate isn't about a hard limit, but rather a careful consideration of feasibility and the complexity of interpretation. While technically you can have many IVs, practically speaking, the number is constrained by several factors. This article will delve into these factors, exploring the advantages and disadvantages of using multiple IVs, and offering guidance on designing robust and interpretable experiments.
Understanding Independent Variables and Experimental Design
Before diving into the number of IVs, let's establish a clear understanding of the concept. An independent variable is the variable that is manipulated or changed by the researcher to observe its effect on the dependent variable (DV). The DV is the variable being measured or observed. A well-designed experiment aims to isolate the effect of the IV on the DV, controlling for extraneous variables that could confound the results.
Simple Experiments: Often, introductory experimental designs focus on a single IV and a single DV. This simplicity allows for a clear demonstration of cause-and-effect relationships. For example, an experiment investigating the effect of fertilizer type (IV) on plant growth (DV) would be a simple, single-IV design.
Factorial Designs: Embracing Multiple IVs
As research questions become more nuanced, the need to explore the interaction effects of multiple IVs arises. This is where factorial designs come into play. A factorial design is an experiment that manipulates two or more IVs simultaneously, allowing researchers to examine not only the main effects of each IV but also their interactions.
For instance, consider an experiment investigating the effects of both fertilizer type (IV1) and watering frequency (IV2) on plant growth (DV). This is a 2 x 2 factorial design (two levels of fertilizer and two levels of watering frequency). The results would reveal:
- Main effects: The individual effect of fertilizer type and watering frequency on plant growth.
- Interaction effects: Whether the effect of fertilizer type depends on the watering frequency, and vice versa. An interaction effect means the combined effect of two IVs is different from the sum of their individual effects.
The Practical Limits: Factors Influencing the Number of IVs
While technically, you could include a large number of IVs in a factorial design, several practical considerations limit this:
1. Participant Numbers and Statistical Power
As you increase the number of IVs and their levels (e.g., a 2 x 2 x 2 design has three IVs, each with two levels), the number of experimental conditions increases exponentially. A 3 x 4 x 2 design, for instance, has 24 different conditions. To achieve sufficient statistical power—the probability of detecting a real effect if one exists—you need a large number of participants, often distributed evenly across all conditions. Recruiting and managing a large participant pool can be time-consuming, costly, and logistically challenging. Insufficient participants can lead to Type II errors (failing to reject a false null hypothesis).
2. Complexity of Interpretation
With more IVs, interpreting the results becomes increasingly complex. Analyzing main effects and interactions between multiple variables can be challenging, particularly when dealing with higher-order interactions (involving three or more IVs). Complex interactions can be difficult to explain theoretically and may require advanced statistical techniques. The risk of overfitting the data – finding patterns that are specific to the sample and don't generalize to the population – increases with a large number of IVs.
3. Experimental Control and Confounding Variables
Maintaining rigorous experimental control becomes more difficult with more IVs. The greater the number of variables being manipulated, the higher the probability of uncontrolled extraneous variables influencing the results. Confounding occurs when the effects of one IV are intertwined with another, making it impossible to isolate the individual effects of each.
4. Resource Constraints
Conducting experiments with many IVs demands significant resources. These resources include:
- Time: More conditions require more time for data collection and analysis.
- Money: Participant compensation, materials, and potentially specialized equipment can become expensive.
- Personnel: Managing a larger and more complex experiment necessitates more researchers or research assistants.
Strategies for Managing Multiple Independent Variables
Instead of trying to cram many IVs into a single experiment, researchers often employ alternative strategies:
1. Prioritize Key Variables: Focus on the Most Important Factors
Carefully consider which IVs are most crucial to your research question. Prioritize those with strong theoretical rationale and potentially significant effects. Start with a smaller, more manageable number of IVs and gradually expand your investigation if needed.
2. Hierarchical Designs: Phased Approach
A hierarchical design involves conducting a series of experiments, each building upon the previous one. Start with a simpler design (e.g., a 2 x 2), analyze the results, and then add additional IVs in subsequent studies based on the findings of the earlier experiments. This approach allows for a more focused and efficient investigation.
3. Mixed-Model Designs: Combining Between- and Within-Subjects Factors
A mixed-model design combines between-subjects factors (different participants assigned to different levels of an IV) and within-subjects factors (the same participants exposed to all levels of an IV). This can sometimes reduce the number of participants needed compared to a purely between-subjects design. However, it also introduces potential issues such as order effects (the order in which conditions are presented influences the results).
4. Advanced Statistical Techniques: Handling Complexity
Advanced statistical methods like ANOVA (Analysis of Variance), regression analysis, and structural equation modeling (SEM) can help analyze data from experiments with multiple IVs. These methods are capable of handling complex interactions and assessing the relative contributions of different IVs to the DV.
Determining the Optimal Number of IVs: A Practical Guide
There isn't a magic number of independent variables suitable for every experiment. The optimal number depends on various factors, including the research question, available resources, and the complexity of the phenomena under investigation.
Start with a strong theoretical framework: A well-defined theoretical foundation will guide your selection of IVs and ensure that your experiment addresses a specific and meaningful research question.
Conduct a thorough literature review: Examine existing research to identify key variables and potential interactions that have been investigated previously. This will help you narrow down the IVs to those that are most relevant and likely to yield valuable insights.
Consider the feasibility of the study: Assess your resources (time, budget, personnel) and determine if you have the capacity to manage a complex experiment with many IVs. A realistic assessment of your resources will help you determine the scope of your investigation.
Pilot study: A pilot study with a smaller number of IVs can help you test your experimental procedures, refine your measures, and assess the feasibility of your design before committing to a larger, more complex study.
Prioritize parsimony: Aim for the simplest design that can adequately address your research question. Avoid adding unnecessary IVs simply to increase the complexity of your study. A simpler design is generally easier to interpret and replicate.
Conclusion: Balancing Ambition with Feasibility
The number of independent variables in an experiment is not arbitrarily limited but rather guided by practical considerations. While including multiple IVs allows for a more comprehensive investigation of complex phenomena, it also introduces complexities in design, data analysis, and interpretation. Researchers must strike a balance between the ambition of exploring multiple variables and the feasibility of conducting a rigorous and interpretable experiment. By carefully considering the factors discussed above and adopting strategic approaches to experimental design, researchers can conduct robust studies with multiple IVs, leading to valuable and reliable scientific conclusions.
Latest Posts
Latest Posts
-
What Is The Product Of 3 And 5
May 11, 2025
-
What Is The Number Under The Element
May 11, 2025
-
What Substance Speeds Up A Chemical Reaction
May 11, 2025
-
How Many Neutrons Does Cr Have
May 11, 2025
-
The Quotient Of A Number And 5
May 11, 2025
Related Post
Thank you for visiting our website which covers about How Many Independent Variables Can You Have In An Experiment . We hope the information provided has been useful to you. Feel free to contact us if you have any questions or need further assistance. See you next time and don't miss to bookmark.