General Formula To Describe The Variation
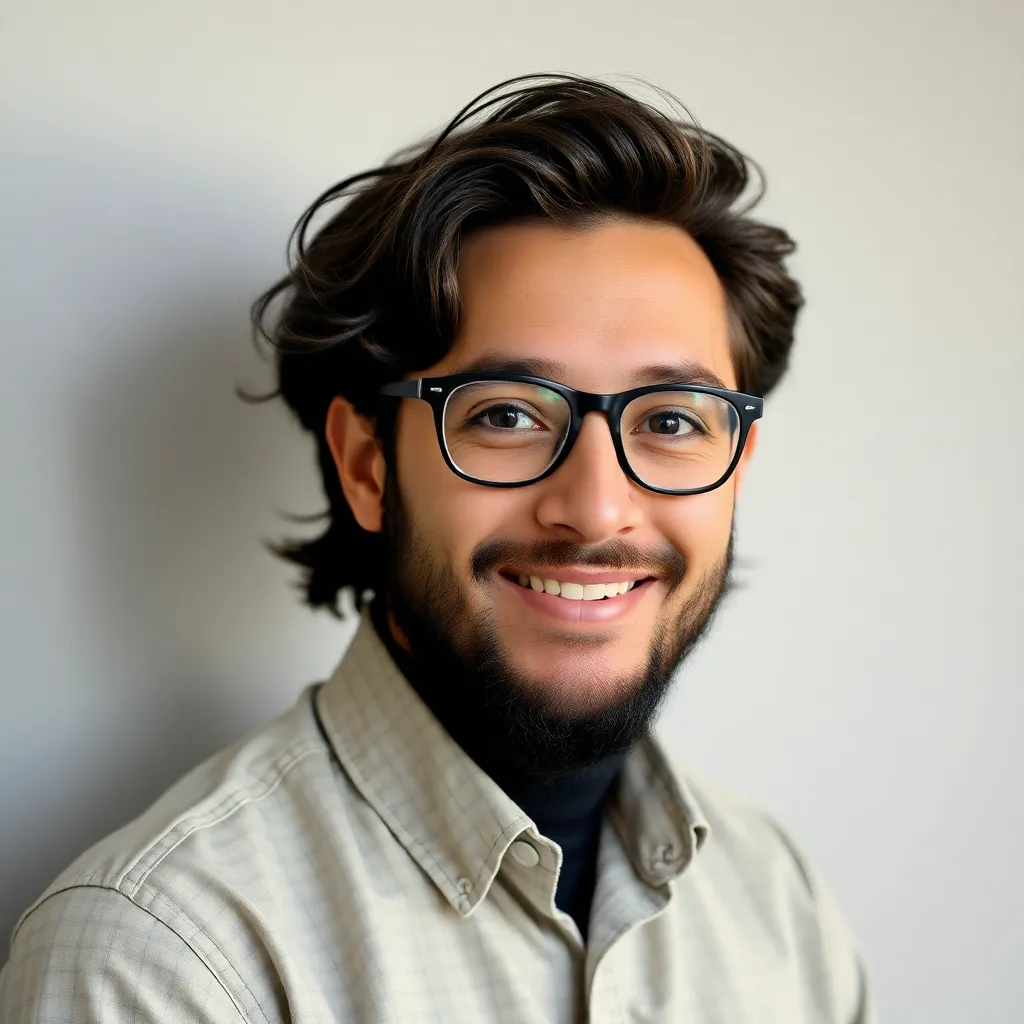
listenit
May 11, 2025 · 5 min read

Table of Contents
The General Formula to Describe Variation: Unveiling the Power of Mathematical Models
Understanding variation is crucial across numerous scientific disciplines and real-world applications. Whether analyzing the spread of a disease, predicting stock market fluctuations, or optimizing manufacturing processes, the ability to describe and model variation is paramount. This article delves into the general formulas and concepts used to represent variation, focusing on its different types and the mathematical tools employed to understand and predict it.
Types of Variation
Before diving into the formulas, it's essential to understand the different types of variation we encounter. These broadly fall into categories based on the relationship between variables:
1. Direct Variation
Direct variation describes a relationship where two variables increase or decrease proportionally. As one variable increases, the other increases at the same rate, and vice-versa. The general formula for direct variation is:
y = kx
Where:
- y and x are the two variables.
- k is the constant of proportionality. This constant represents the rate of change between the variables.
Example: The distance traveled (y) is directly proportional to the speed (x) if the time is constant. A faster speed leads to a greater distance covered.
2. Inverse Variation
Inverse variation depicts a relationship where an increase in one variable leads to a decrease in the other, and vice-versa. The product of the variables remains constant. The general formula for inverse variation is:
y = k/x
Where:
- y and x are the two variables.
- k is the constant of variation.
Example: The time (y) it takes to complete a task is inversely proportional to the number of workers (x). More workers result in less time needed.
3. Joint Variation
Joint variation involves three or more variables where one variable is directly proportional to the product of two or more other variables. The general formula is:
z = kxy
Where:
- z is the dependent variable.
- x and y are the independent variables.
- k is the constant of variation.
Example: The volume (z) of a cylinder is jointly proportional to its height (x) and the square of its radius (y).
4. Combined Variation
Combined variation is a more complex type incorporating both direct and inverse variations. One variable may be directly proportional to some variables and inversely proportional to others. The general formula is a combination of direct and inverse variation formulas. For instance:
z = kx/y
Where:
- z is the dependent variable.
- x and y are the independent variables.
- k is the constant of variation.
Example: The force of gravity (z) is directly proportional to the product of the masses (x) and inversely proportional to the square of the distance (y) between them (Newton's Law of Universal Gravitation).
Mathematical Tools for Describing Variation
Several mathematical tools aid in describing and analyzing variation, including:
1. Statistical Measures
- Mean (Average): Provides a central tendency measure, indicating the typical value.
- Median: Represents the middle value when data is ordered.
- Mode: Shows the most frequent value.
- Range: Indicates the spread of data by calculating the difference between the highest and lowest values.
- Variance and Standard Deviation: Quantify the dispersion or spread of data around the mean. A higher standard deviation signifies greater variability.
2. Regression Analysis
Regression analysis, especially linear regression, is crucial for modeling relationships between variables. It allows us to find the best-fitting line (or curve) that describes the relationship, enabling prediction and understanding of how changes in one variable affect another. The equation for a simple linear regression is:
y = mx + c
Where:
- y is the dependent variable.
- x is the independent variable.
- m is the slope (representing the rate of change).
- c is the y-intercept (the value of y when x is 0).
3. Correlation Analysis
Correlation analysis measures the strength and direction of the linear relationship between two variables. The correlation coefficient (often denoted as 'r') ranges from -1 to +1:
- +1: Perfect positive correlation (variables increase together).
- 0: No linear correlation.
- -1: Perfect negative correlation (variables move in opposite directions).
4. Probability Distributions
Probability distributions describe the likelihood of different outcomes for a variable. Different distributions, such as the normal distribution, binomial distribution, or Poisson distribution, model variations in various contexts. These distributions help predict the probability of certain events occurring within a specific range.
Applications of Variation Models
The ability to describe and model variation is essential in countless fields:
1. Finance
- Stock market prediction: Models analyze historical stock prices to predict future movements, accounting for inherent volatility.
- Risk assessment: Variation in investment returns is quantified to assess risk and inform portfolio diversification strategies.
2. Healthcare
- Disease spread modeling: Epidemiological models track disease transmission, factoring in variations in infection rates and population susceptibility.
- Clinical trials: Statistical analysis of variation in treatment outcomes helps determine the effectiveness of new drugs or therapies.
3. Manufacturing
- Quality control: Variation in product dimensions or performance is monitored to maintain quality standards.
- Process optimization: Models identify sources of variation in manufacturing processes to improve efficiency and reduce waste.
4. Environmental Science
- Climate change modeling: Variations in temperature, precipitation, and sea levels are analyzed to predict the impacts of climate change.
- Pollution monitoring: Variations in pollutant concentrations are tracked to assess environmental health and inform pollution control policies.
Advanced Concepts and Considerations
While the formulas presented above provide a foundational understanding, describing variation often requires more advanced techniques:
- Time series analysis: Deals with data collected over time, considering trends, seasonality, and randomness.
- Non-linear models: Used when the relationship between variables is not linear. Examples include polynomial regression and other non-linear regression techniques.
- Multivariate analysis: Analyzes the relationships between multiple variables simultaneously, providing a more comprehensive understanding of complex systems.
- Bayesian methods: Incorporate prior knowledge and uncertainty into models, leading to more robust estimations of variation.
Conclusion
Describing variation accurately is critical for understanding and predicting phenomena across various disciplines. The fundamental formulas for direct, inverse, joint, and combined variation provide a starting point. However, statistical measures, regression analysis, correlation analysis, and probability distributions are invaluable tools for a more profound understanding. Furthermore, advanced techniques like time series analysis and multivariate analysis are necessary for dealing with the complexities often found in real-world datasets. Mastering these tools empowers us to make data-driven decisions and navigate the inherent variability present in our world. Continuous learning and refinement of these modeling techniques are vital for staying ahead in a world that constantly generates new data and presents novel challenges.
Latest Posts
Latest Posts
-
Why Do Electric Field Lines Never Cross
May 13, 2025
-
1 10 As A Percent And Decimal
May 13, 2025
-
Can All Minerals Be A Gemstone
May 13, 2025
-
Multicellular Heterotrophs Without A Cell Wall
May 13, 2025
-
What Are The Gcf Of 48
May 13, 2025
Related Post
Thank you for visiting our website which covers about General Formula To Describe The Variation . We hope the information provided has been useful to you. Feel free to contact us if you have any questions or need further assistance. See you next time and don't miss to bookmark.