Finding Critical Points Of Multivariable Functions
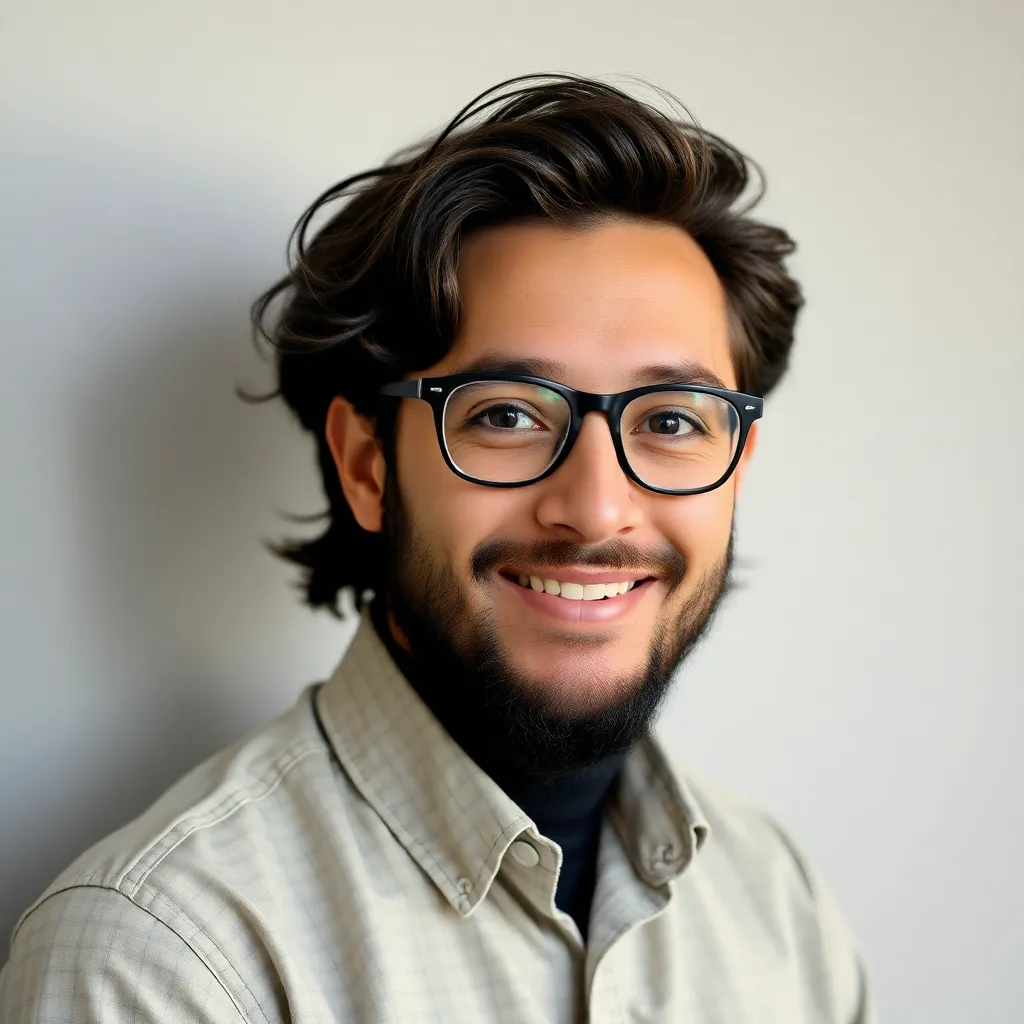
listenit
Apr 16, 2025 · 6 min read

Table of Contents
Finding Critical Points of Multivariable Functions: A Comprehensive Guide
Finding critical points is a fundamental concept in multivariable calculus, crucial for understanding the behavior of functions of several variables. These points represent potential locations of local maxima, minima, or saddle points – key features that shape the function's landscape. This comprehensive guide will delve into the methods and techniques used to identify critical points, providing a clear and detailed understanding for both students and professionals.
Understanding Critical Points
Before diving into the methods, let's establish a firm grasp on what constitutes a critical point. For a function of a single variable, f(x), a critical point occurs where the derivative, f'(x), is zero or undefined. This signifies a potential change in the function's behavior – a shift from increasing to decreasing, or vice versa.
In the realm of multivariable functions, the concept extends naturally. Consider a function of two variables, f(x, y). A critical point is a point (x₀, y₀) in the domain of f(x, y) where both partial derivatives are zero, or at least one partial derivative is undefined. Formally:
- ∇f(x₀, y₀) = 0 where ∇f represents the gradient vector: ∇f = (∂f/∂x, ∂f/∂y)
This means:
- ∂f/∂x (x₀, y₀) = 0
- ∂f/∂y (x₀, y₀) = 0
The same principle extends to functions with more than two variables. For a function f(x₁, x₂, ..., xₙ), a critical point occurs when all partial derivatives are zero:
- ∂f/∂x₁ = 0
- ∂f/∂x₂ = 0
- ...
- ∂f/∂xₙ = 0
Locating Critical Points: A Step-by-Step Process
The process of finding critical points involves a systematic approach:
-
Calculate Partial Derivatives: Begin by computing the partial derivatives of the function with respect to each variable. This step requires a thorough understanding of differentiation techniques for multivariable functions.
-
Set Partial Derivatives to Zero: Set each partial derivative equal to zero. This creates a system of equations.
-
Solve the System of Equations: Solving this system of equations is often the most challenging step. The complexity depends heavily on the function's nature. You might encounter linear equations, non-linear equations, or even systems requiring advanced techniques like numerical methods.
-
Identify Critical Points: The solutions to the system of equations represent the coordinates (x₀, y₀, ...) of the critical points.
Classifying Critical Points: The Second Partial Derivative Test
Once critical points are identified, the next crucial step is classifying them. This determines whether each critical point corresponds to a local maximum, a local minimum, or a saddle point. For functions of two variables, the second partial derivative test is the standard approach. This test utilizes the Hessian matrix:
Hessian Matrix (H):
H = | ∂²f/∂x² ∂²f/∂x∂y |
| ∂²f/∂y∂x ∂²f/∂y² |
The test proceeds as follows:
-
Compute Second Partial Derivatives: Calculate all second-order partial derivatives: ∂²f/∂x², ∂²f/∂y², ∂²f/∂x∂y, and ∂²f/∂y∂x. Note that if the function is sufficiently smooth (i.e., has continuous second partial derivatives), then ∂²f/∂x∂y = ∂²f/∂y∂x (Clairaut's Theorem).
-
Evaluate the Hessian at Each Critical Point: Substitute the coordinates of each critical point into the Hessian matrix to obtain a specific Hessian matrix for each point.
-
Calculate the Determinant (D) and the Second Partial Derivative with respect to x (∂²f/∂x²):
- D = det(H) = (∂²f/∂x²)(∂²f/∂y²) - (∂²f/∂x∂y)²
-
Classify Based on D and ∂²f/∂x²:
- D > 0 and ∂²f/∂x² > 0: Local minimum
- D > 0 and ∂²f/∂x² < 0: Local maximum
- D < 0: Saddle point
- D = 0: The test is inconclusive. Further investigation is needed, potentially using higher-order derivatives or graphical analysis.
Extending to Functions of More Than Two Variables
For functions of three or more variables, the second partial derivative test generalizes, but the classification becomes more complex. The Hessian becomes a larger matrix, and the determinant is used similarly to determine concavity. However, the interpretation of the results is less intuitive. In these higher-dimensional cases, numerical methods and specialized software often become essential for both locating and classifying critical points.
Example: Finding and Classifying Critical Points
Let's consider the function: f(x, y) = x³ - 3x + y²
-
Partial Derivatives:
- ∂f/∂x = 3x² - 3
- ∂f/∂y = 2y
-
Set to Zero:
- 3x² - 3 = 0 => x² = 1 => x = ±1
- 2y = 0 => y = 0
-
Critical Points: The critical points are (1, 0) and (-1, 0).
-
Second Partial Derivatives:
- ∂²f/∂x² = 6x
- ∂²f/∂y² = 2
- ∂²f/∂x∂y = 0
-
Hessian Matrix:
H = | 6x 0 | | 0 2 |
-
Classification:
- (1, 0): D = (6)(2) - 0² = 12 > 0, and ∂²f/∂x² = 6 > 0. Therefore, (1, 0) is a local minimum.
- (-1, 0): D = (-6)(2) - 0² = -12 < 0. Therefore, (-1, 0) is a saddle point.
Handling Constraints: Lagrange Multipliers
Often, we need to find critical points subject to constraints. For instance, we might want to find the maximum or minimum value of a function f(x, y) along a curve defined by g(x, y) = 0. This is where the method of Lagrange multipliers comes into play.
The core idea is to introduce a new variable, λ (the Lagrange multiplier), and form the Lagrangian function:
L(x, y, λ) = f(x, y) - λg(x, y)
Critical points are then found by solving the following system of equations:
- ∇f = λ∇g
- g(x, y) = 0
Advanced Techniques and Considerations
Finding critical points can become significantly more challenging with complex functions or numerous variables. Several advanced techniques can be helpful:
-
Numerical Methods: For functions that are difficult or impossible to solve analytically, numerical methods like Newton-Raphson are indispensable. These iterative methods provide approximate solutions.
-
Software Tools: Mathematical software packages like Mathematica, MATLAB, and others provide powerful tools for symbolic and numerical computation, significantly easing the process.
-
Visualization: Visualizing the function's surface can offer valuable insights and help in identifying potential critical points, particularly when dealing with complex functions.
Conclusion
Finding critical points of multivariable functions is a cornerstone of multivariable calculus with far-reaching applications in optimization, physics, engineering, and various other fields. Understanding the techniques presented here, including the second partial derivative test and Lagrange multipliers, empowers one to analyze and understand the behavior of multivariable functions effectively. Remember that while the fundamental principles remain consistent, the complexity can increase significantly with the number of variables and the nature of the function. Mastering these concepts provides a strong foundation for tackling more advanced problems in calculus and related disciplines.
Latest Posts
Latest Posts
-
Difference Between Oceanic Plates And Continental Plates
Apr 16, 2025
-
What Is 9 Percent Of 72
Apr 16, 2025
-
98 As A Product Of Prime Factors
Apr 16, 2025
-
What Is A Change In Velocity Called
Apr 16, 2025
-
Z Varies Jointly As X And Y
Apr 16, 2025
Related Post
Thank you for visiting our website which covers about Finding Critical Points Of Multivariable Functions . We hope the information provided has been useful to you. Feel free to contact us if you have any questions or need further assistance. See you next time and don't miss to bookmark.