Find The Mean Of Probability Distribution
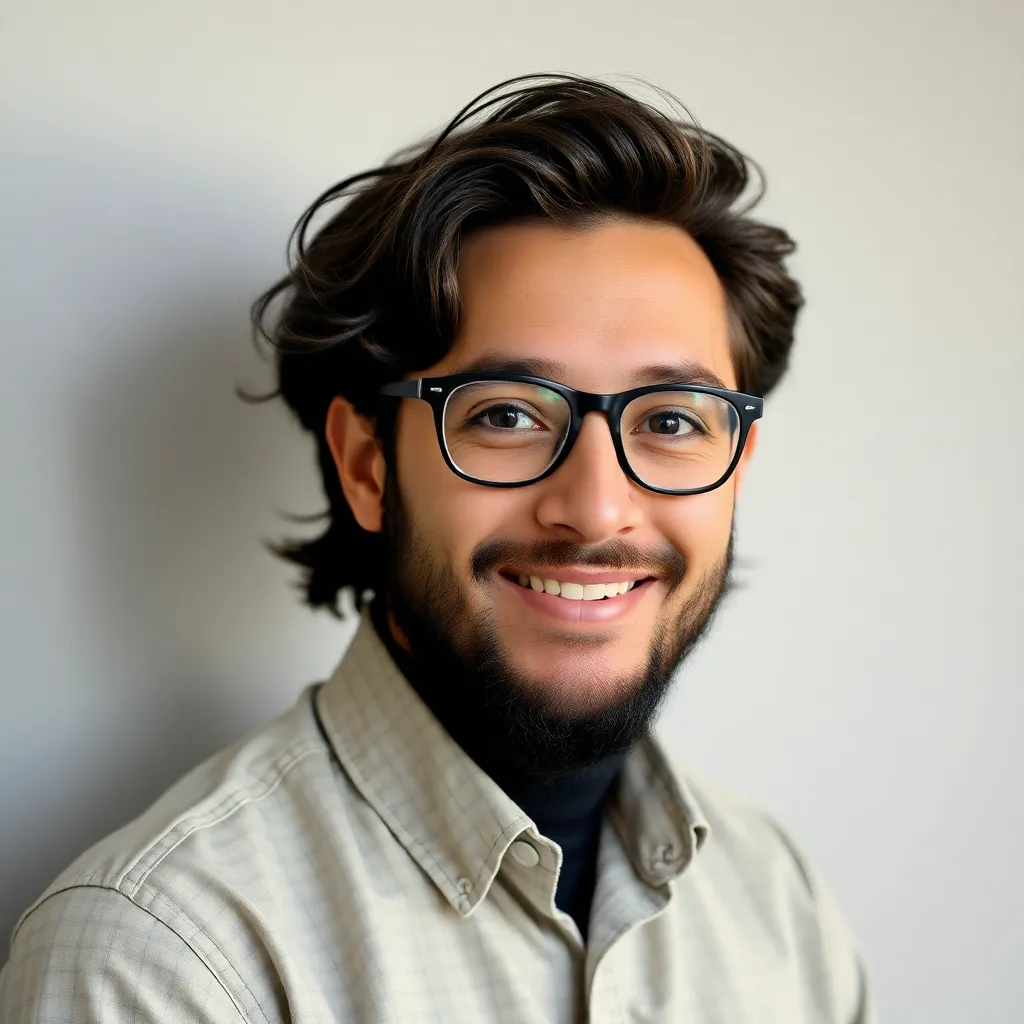
listenit
May 12, 2025 · 5 min read
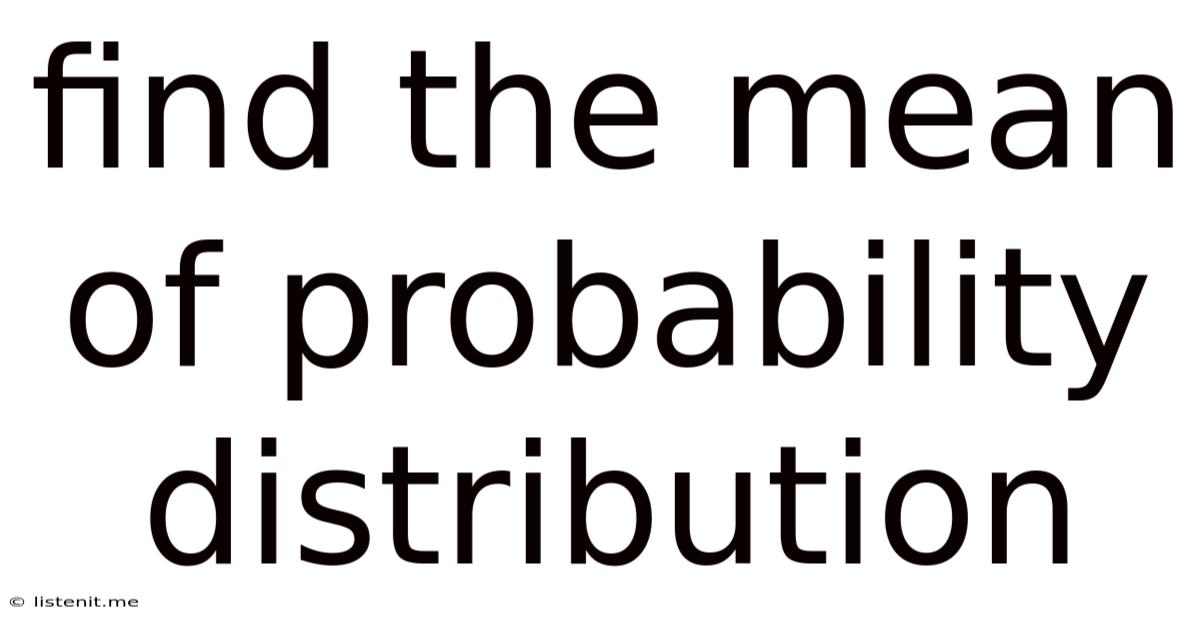
Table of Contents
Finding the Mean of a Probability Distribution: A Comprehensive Guide
Understanding the mean of a probability distribution is crucial in statistics and numerous applications across various fields. The mean, also known as the expected value, provides a measure of the central tendency of a random variable. This comprehensive guide will delve into the intricacies of calculating the mean for different types of probability distributions, offering clear explanations and practical examples. We'll explore both discrete and continuous distributions, providing you with the tools to confidently tackle this essential statistical concept.
What is the Mean of a Probability Distribution?
The mean of a probability distribution represents the average value of a random variable, weighted by its probability. It's a crucial summary statistic that provides insights into the distribution's center. Intuitively, it tells you what the "typical" or "expected" value of the random variable would be if you were to conduct many repetitions of the random experiment.
For a discrete random variable, the mean is calculated as the sum of each possible value multiplied by its probability. For a continuous random variable, the mean is calculated using integration. Understanding the type of distribution is the first critical step in determining the correct method.
Calculating the Mean for Discrete Probability Distributions
For a discrete probability distribution, the mean (often denoted as μ or E(X)) is calculated using the following formula:
μ = Σ [x * P(X = x)]
Where:
- x represents each possible value of the discrete random variable X.
- P(X = x) represents the probability of the random variable X taking on the value x.
- Σ denotes the summation over all possible values of x.
Example: The Mean of a Simple Discrete Distribution
Let's consider a simple example: A fair six-sided die is rolled. The random variable X represents the outcome of the roll. The probability distribution is:
- P(X = 1) = 1/6
- P(X = 2) = 1/6
- P(X = 3) = 1/6
- P(X = 4) = 1/6
- P(X = 5) = 1/6
- P(X = 6) = 1/6
To find the mean:
μ = (1 * 1/6) + (2 * 1/6) + (3 * 1/6) + (4 * 1/6) + (5 * 1/6) + (6 * 1/6) = 3.5
Therefore, the mean of this distribution is 3.5. This makes intuitive sense; the average outcome of rolling a fair six-sided die is 3.5.
Example: A More Complex Discrete Distribution – Binomial Distribution
The binomial distribution models the probability of getting a certain number of successes in a fixed number of independent Bernoulli trials. The mean of a binomial distribution is particularly straightforward:
μ = n * p
Where:
- n is the number of trials.
- p is the probability of success in a single trial.
For example, if you flip a coin 10 times (n = 10) and the probability of heads (success) is 0.5 (p = 0.5), the mean number of heads is:
μ = 10 * 0.5 = 5
This intuitively means you'd expect to get 5 heads on average if you repeated this experiment many times.
Example: Poisson Distribution
The Poisson distribution models the probability of a given number of events occurring in a fixed interval of time or space if these events occur with a known average rate and independently of the time since the last event. The mean of a Poisson distribution is simply its parameter, λ (lambda):
μ = λ
Calculating the Mean for Continuous Probability Distributions
For continuous probability distributions, the mean is calculated using integration. The formula is:
μ = ∫ x * f(x) dx
Where:
- x represents the value of the continuous random variable.
- f(x) is the probability density function (PDF) of the random variable.
- ∫ denotes integration over the entire range of x.
Example: The Mean of an Exponential Distribution
The exponential distribution is often used to model the time until an event occurs in a Poisson process. Its probability density function is:
f(x) = λe^(-λx) for x ≥ 0, where λ > 0.
To find the mean:
μ = ∫₀^∞ x * λe^(-λx) dx = 1/λ
Therefore, the mean of an exponential distribution is the reciprocal of its rate parameter, λ.
Example: The Mean of a Normal Distribution
The normal distribution, arguably the most important distribution in statistics, is characterized by its mean (μ) and standard deviation (σ). Conveniently, the mean of a normal distribution is simply its parameter μ.
Example: The Mean of a Uniform Distribution
A continuous uniform distribution has a constant probability density function over a specified interval [a, b]. The mean is simply the midpoint of the interval:
μ = (a + b) / 2
Applications of Finding the Mean of a Probability Distribution
Calculating the mean of a probability distribution has numerous applications across various fields:
- Finance: Calculating expected returns on investments, assessing portfolio risk.
- Insurance: Determining expected claim payouts, setting premiums.
- Engineering: Modeling system reliability, predicting failure rates.
- Healthcare: Analyzing disease prevalence, predicting patient outcomes.
- Quality Control: Monitoring manufacturing processes, assessing product defects.
- Queueing Theory: Modeling waiting times in systems, optimizing service capacity.
- Actuarial Science: Assessing risk and setting insurance premiums.
- Data Science: Understanding data distributions, creating predictive models.
Beyond the Mean: Other Measures of Central Tendency
While the mean is a valuable measure of central tendency, it's important to note that it's not always the best choice. Outliers can significantly influence the mean, making it less representative of the data's central tendency. In such cases, other measures like the median (the middle value when data is sorted) or the mode (the most frequent value) might be more appropriate.
Conclusion
Understanding how to find the mean of a probability distribution is a fundamental skill in statistics and data analysis. This guide has provided a comprehensive overview of the methods for calculating the mean for both discrete and continuous distributions, along with practical examples to illustrate the concepts. By mastering this skill, you'll gain a powerful tool for interpreting data, making predictions, and solving problems across a wide range of fields. Remember to always consider the context of your data and choose the most appropriate measure of central tendency for your specific needs. Understanding the limitations of the mean and exploring alternative measures ensures a more robust and accurate analysis.
Latest Posts
Latest Posts
-
675 As A Fraction In Simplest Form
May 12, 2025
-
What Is The Whole Number For 1 3
May 12, 2025
-
What Is 20 Of 60 Dollars
May 12, 2025
-
Baking Cookies Physical Or Chemical Change
May 12, 2025
-
What Is The Simplest Form Of 5 10
May 12, 2025
Related Post
Thank you for visiting our website which covers about Find The Mean Of Probability Distribution . We hope the information provided has been useful to you. Feel free to contact us if you have any questions or need further assistance. See you next time and don't miss to bookmark.