Explanatory Vs Response Variable X And Y
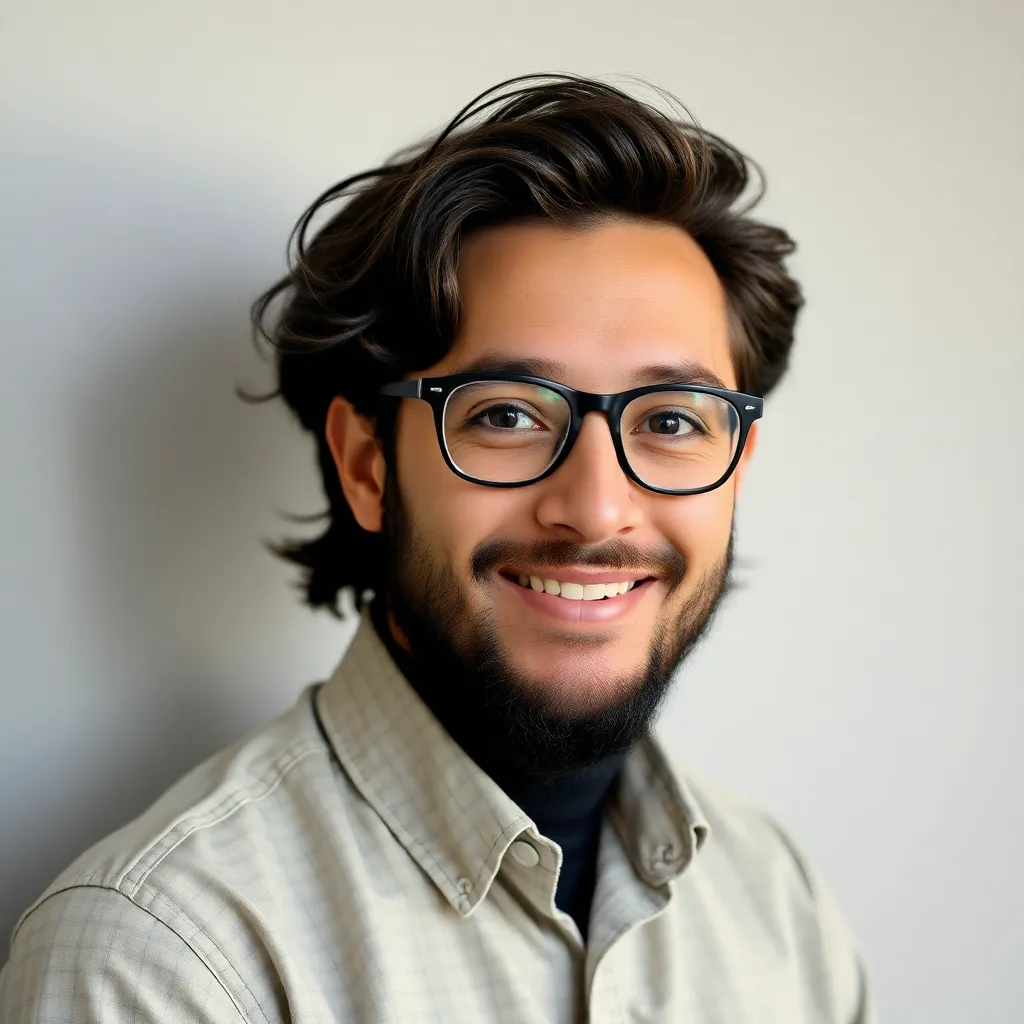
listenit
May 13, 2025 · 5 min read
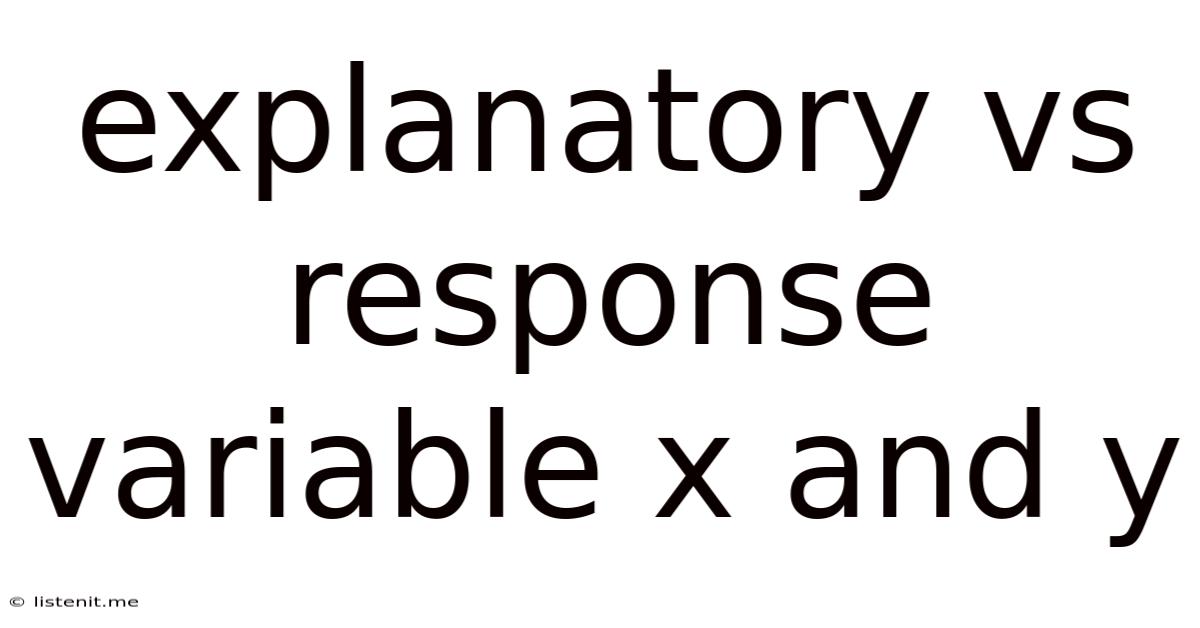
Table of Contents
Explanatory vs. Response Variable: Understanding the X and Y in Your Data
Understanding the relationship between variables is fundamental to statistical analysis and data interpretation. In many statistical analyses, we're interested in exploring how one variable influences another. This often involves identifying an explanatory variable (also called the independent variable or predictor variable) and a response variable (also called the dependent variable or outcome variable). While seemingly simple, the distinction between these two is crucial for correctly designing experiments, interpreting results, and drawing meaningful conclusions. This comprehensive guide will delve deep into the nuances of explanatory and response variables, exploring their roles in various statistical methods and providing real-world examples to solidify your understanding.
Defining Explanatory and Response Variables
Before we delve into the complexities, let's establish clear definitions:
-
Explanatory Variable (X): This variable is believed to influence or explain changes in the response variable. It's the variable that is manipulated or observed in an experiment or study. It's considered the cause (though correlation doesn't equal causation). It's often plotted on the horizontal (x) axis in graphs.
-
Response Variable (Y): This variable is the outcome or effect that is being measured or observed. It's the variable that is believed to be influenced by the explanatory variable. It's considered the effect (though again, correlation doesn't equal causation). It's often plotted on the vertical (y) axis in graphs.
The Crucial Distinction: Causation vs. Correlation
It's imperative to emphasize that identifying an explanatory and response variable doesn't automatically imply a causal relationship. Correlation simply means that two variables tend to change together. A strong correlation doesn't prove that one variable causes the change in the other. There could be other underlying factors (confounding variables) at play.
For example, a strong positive correlation might exist between ice cream sales and drowning incidents. However, this doesn't mean ice cream consumption causes drowning. Both are likely influenced by a third variable: hot weather.
Examples of Explanatory and Response Variables Across Disciplines
To further illuminate the concept, let's examine examples across various fields:
1. Medicine:
-
Study: Investigating the effect of a new drug on blood pressure.
- Explanatory Variable (X): Dosage of the new drug (e.g., low, medium, high).
- Response Variable (Y): Blood pressure measurement.
-
Study: Examining the relationship between smoking and lung cancer.
- Explanatory Variable (X): Number of cigarettes smoked per day.
- Response Variable (Y): Incidence of lung cancer.
2. Education:
-
Study: Assessing the impact of a new teaching method on student test scores.
- Explanatory Variable (X): Teaching method (e.g., traditional vs. new method).
- Response Variable (Y): Student test scores.
-
Study: Exploring the correlation between hours of study and GPA.
- Explanatory Variable (X): Hours of study per week.
- Response Variable (Y): Grade Point Average (GPA).
3. Economics:
-
Study: Analyzing the effect of interest rates on consumer spending.
- Explanatory Variable (X): Interest rates.
- Response Variable (Y): Consumer spending levels.
-
Study: Investigating the relationship between advertising expenditure and sales revenue.
- Explanatory Variable (X): Advertising expenditure.
- Response Variable (Y): Sales revenue.
4. Environmental Science:
-
Study: Examining the impact of pollution levels on fish populations.
- Explanatory Variable (X): Levels of water pollution.
- Response Variable (Y): Size of fish populations.
-
Study: Investigating the relationship between carbon dioxide emissions and global temperature.
- Explanatory Variable (X): Carbon dioxide emissions.
- Response Variable (Y): Global average temperature.
Identifying X and Y in Different Statistical Methods
The identification of explanatory and response variables is crucial for selecting appropriate statistical methods. Different methods are designed for different types of data and relationships between variables.
-
Regression Analysis: This is used to model the relationship between a response variable and one or more explanatory variables. Linear regression is a common type used when the relationship is linear.
-
Correlation Analysis: This measures the strength and direction of the linear relationship between two variables, but doesn't imply causation. It's often a preliminary step before more complex analyses.
-
ANOVA (Analysis of Variance): This is used to compare the means of a response variable across different groups defined by an explanatory variable (often categorical).
-
t-tests: These are used to compare the means of a response variable between two groups.
Challenges and Considerations in Identifying X and Y
While the concept seems straightforward, several complexities can arise:
-
Multiple Explanatory Variables: Many real-world situations involve multiple factors influencing the response variable. Multiple regression analysis is designed to handle these situations.
-
Confounding Variables: These are variables that affect both the explanatory and response variables, potentially leading to spurious correlations. Careful experimental design and statistical control are necessary to mitigate their impact.
-
Non-linear Relationships: The relationship between X and Y may not always be linear. Nonlinear regression techniques are needed in such cases.
-
Causality vs. Association: As repeatedly emphasized, correlation does not equal causation. Careful consideration of potential confounding variables and other factors is crucial to avoid misinterpreting results.
Beyond Simple X and Y: Exploring Complex Relationships
The simple X and Y framework can be expanded to encompass more intricate relationships. For example:
-
Mediation: A mediator variable explains the mechanism through which an explanatory variable influences a response variable.
-
Moderation: A moderator variable alters the strength or direction of the relationship between the explanatory and response variables.
Conclusion: Mastering the Explanatory and Response Variable Distinction
Understanding the difference between explanatory and response variables is paramount for conducting rigorous and meaningful statistical analyses. By correctly identifying these variables and considering potential confounding factors and complex relationships, researchers can draw accurate conclusions and contribute valuable insights to their respective fields. The examples provided in this guide highlight the versatility of these concepts across a multitude of disciplines, emphasizing their importance in various statistical methods. Remember that while identifying X and Y is crucial, it's equally crucial to acknowledge that correlation doesn't necessarily translate into direct causation. Careful interpretation and consideration of potential confounding factors are essential for accurate conclusions. By mastering this distinction, you'll significantly enhance your ability to interpret data and understand the world around you.
Latest Posts
Latest Posts
-
Why Do Electric Field Lines Never Cross
May 13, 2025
-
1 10 As A Percent And Decimal
May 13, 2025
-
Can All Minerals Be A Gemstone
May 13, 2025
-
Multicellular Heterotrophs Without A Cell Wall
May 13, 2025
-
What Are The Gcf Of 48
May 13, 2025
Related Post
Thank you for visiting our website which covers about Explanatory Vs Response Variable X And Y . We hope the information provided has been useful to you. Feel free to contact us if you have any questions or need further assistance. See you next time and don't miss to bookmark.