Difference Between Chi Square Homogeneity And Independence
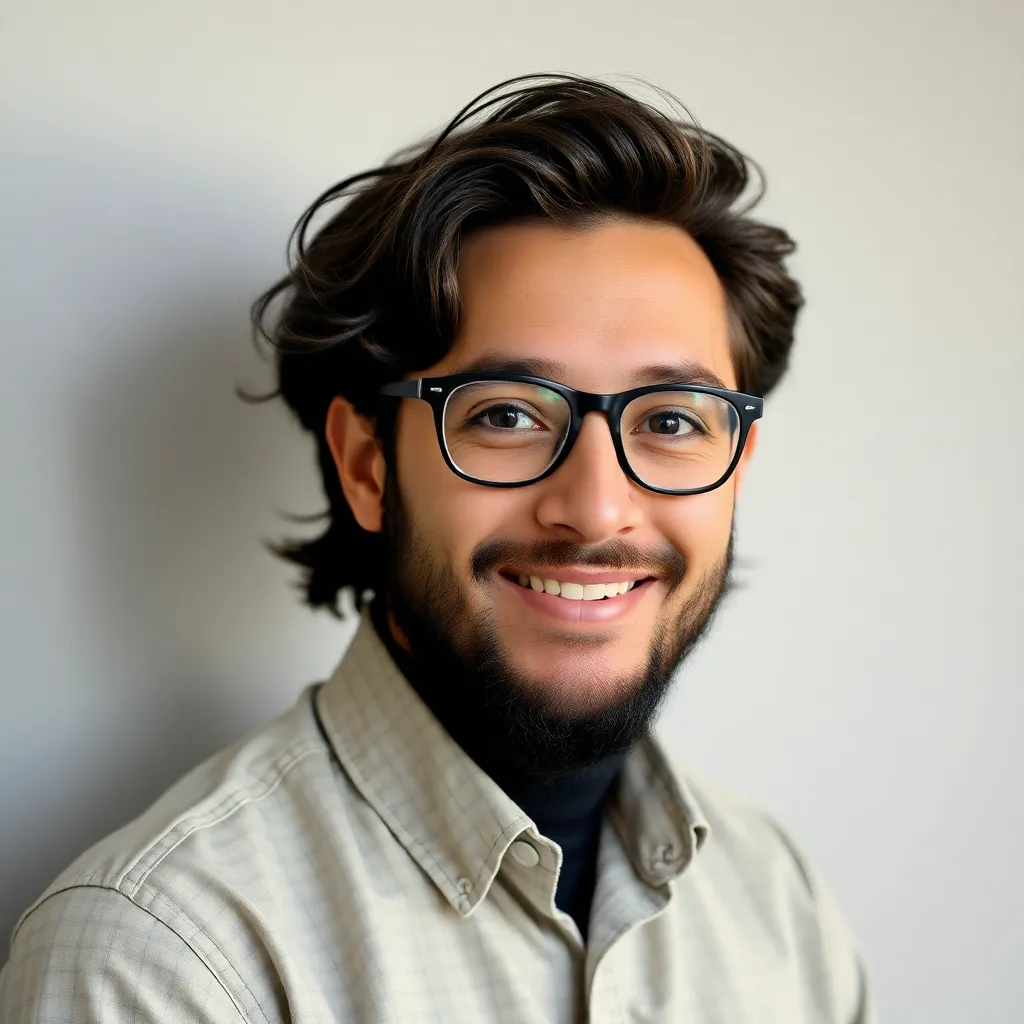
listenit
Apr 26, 2025 · 6 min read

Table of Contents
Chi-Square Test of Homogeneity vs. Independence: A Comprehensive Guide
The chi-square test is a powerful statistical tool used to analyze categorical data. However, two distinct applications of the chi-square test often cause confusion: the test of homogeneity and the test of independence. While both use the same underlying chi-square distribution and similar calculations, they address different research questions and involve different data collection strategies. This comprehensive guide will dissect the key differences between these two tests, ensuring you can confidently choose and interpret the correct one for your analysis.
Understanding the Chi-Square Test
Before delving into the nuances of homogeneity and independence, let's establish a foundation in the chi-square test itself. This statistical test assesses the discrepancy between observed frequencies and expected frequencies in a contingency table. A contingency table, also known as a cross-tabulation, displays the frequencies of observations across two or more categorical variables.
The core idea is to determine if the observed differences between the frequencies are statistically significant or simply due to random chance. A significant chi-square statistic indicates that the observed frequencies deviate substantially from what would be expected under the null hypothesis. The null hypothesis varies depending on whether you are conducting a test of homogeneity or independence.
Chi-Square Test of Independence
The chi-square test of independence examines the relationship between two categorical variables within a single population. The primary question it addresses is: Are the two variables independent of each other, or is there an association between them?
Example: Imagine you want to investigate whether there's a relationship between gender (male/female) and preference for a particular type of coffee (e.g., latte, cappuccino, espresso). You collect data from a single sample of coffee drinkers and categorize them based on both gender and coffee preference. You then use a chi-square test of independence to determine if gender influences coffee preference.
Null Hypothesis: The null hypothesis (H0) for a chi-square test of independence states that there is no association between the two categorical variables. In other words, the variables are independent.
Alternative Hypothesis: The alternative hypothesis (H1) states that there is an association between the two variables.
Data Collection: Data is collected from a single sample representing the population of interest. Each individual in the sample is classified according to both variables.
Chi-Square Test of Homogeneity
The chi-square test of homogeneity, on the other hand, compares the distribution of a single categorical variable across multiple populations. The central question here is: Are the distributions of the categorical variable similar across these populations?
Example: Let's say you want to compare the distribution of political affiliations (e.g., Democrat, Republican, Independent) among three different age groups (18-30, 31-50, 51-70). You collect independent samples from each age group and analyze the distribution of political affiliations within each sample. You would then use a chi-square test of homogeneity to see if the distribution of political affiliations is consistent across the three age groups.
Null Hypothesis: The null hypothesis (H0) for a chi-square test of homogeneity posits that the distributions of the categorical variable are the same across all populations.
Alternative Hypothesis: The alternative hypothesis (H1) states that the distributions of the categorical variable are not the same across all populations. At least one population differs significantly from the others.
Data Collection: Data is collected from multiple independent samples, each representing a different population. The same categorical variable is measured in each sample.
Key Differences Summarized
Feature | Chi-Square Test of Independence | Chi-Square Test of Homogeneity |
---|---|---|
Research Question | Is there an association between two variables in a single population? | Are the distributions of a single variable similar across multiple populations? |
Number of Samples | One sample | Multiple independent samples |
Null Hypothesis | No association between the two variables | Distributions are the same across all populations |
Alternative Hypothesis | Association exists between the two variables | Distributions are different across at least one population |
Data Structure | Single contingency table with two categorical variables | Multiple contingency tables (or a single table with multiple groups) with one categorical variable |
Choosing the Right Test
The choice between the chi-square test of independence and homogeneity depends entirely on your research question and the nature of your data. If you're investigating a potential relationship between two variables within a single sample, use the test of independence. If you are comparing the distribution of a single variable across multiple groups, use the test of homogeneity.
It's crucial to correctly identify which test is appropriate for your research, as misinterpreting the results can lead to inaccurate conclusions.
Interpreting the Results
Regardless of whether you're using a test of independence or homogeneity, the interpretation of the chi-square statistic follows the same general principles:
-
p-value: The p-value represents the probability of observing the data (or more extreme data) if the null hypothesis is true. A small p-value (typically less than 0.05) indicates strong evidence against the null hypothesis, leading to rejection of the null hypothesis. In the context of independence, a small p-value suggests a significant association between the variables. In the context of homogeneity, a small p-value suggests significant differences in the distribution across the populations.
-
Effect Size: While the p-value tells you if the difference is statistically significant, it doesn't indicate the magnitude of the difference. Effect size measures quantify the strength of the association or the size of the differences between groups. Common effect size measures for chi-square tests include Cramer's V and phi coefficient. A larger effect size implies a stronger association or more substantial differences between groups.
-
Contingency Table: A well-presented contingency table showing the observed and expected frequencies provides valuable insights into the nature and magnitude of the associations or differences discovered. This allows for a more nuanced understanding beyond the p-value alone.
Practical Considerations and Common Mistakes
-
Expected Frequencies: The chi-square test assumes that the expected frequencies in each cell of the contingency table are sufficiently large (generally at least 5). If this assumption is violated, alternative methods like Fisher's exact test might be more appropriate.
-
Sample Size: A larger sample size generally increases the power of the chi-square test, making it more likely to detect a true effect. However, a very large sample size can lead to statistically significant results even if the effect size is practically negligible.
-
Multiple Comparisons: When testing for homogeneity across many groups, performing multiple comparisons can inflate the type I error rate (the probability of incorrectly rejecting the null hypothesis). Adjustments like the Bonferroni correction may be necessary.
-
Causation vs. Correlation: A significant chi-square result only indicates an association between variables (or differences in distributions), not causation. Correlation doesn't imply causation. Further investigation may be needed to determine if one variable causes changes in the other.
Conclusion
The chi-square test of independence and homogeneity are valuable tools for analyzing categorical data, but their application depends heavily on the research question and study design. Understanding the fundamental differences between these tests—particularly the distinction between a single sample versus multiple independent samples—is crucial for correctly applying the appropriate test and interpreting the results accurately. Remember always to consider the p-value, effect size, and the details within the contingency table for a complete interpretation. By carefully selecting the appropriate test and interpreting the results with caution, researchers can draw meaningful and reliable conclusions from their categorical data.
Latest Posts
Latest Posts
-
What Is 44 Percent Of 50
Apr 27, 2025
-
Does Nacl Have A High Melting Point
Apr 27, 2025
-
3 And 2 5 As An Improper Fraction
Apr 27, 2025
-
Can Mitochondria Be Seen With A Light Microscope
Apr 27, 2025
-
What Is 8 Divided By 64
Apr 27, 2025
Related Post
Thank you for visiting our website which covers about Difference Between Chi Square Homogeneity And Independence . We hope the information provided has been useful to you. Feel free to contact us if you have any questions or need further assistance. See you next time and don't miss to bookmark.