Conditions For One Sample T Test
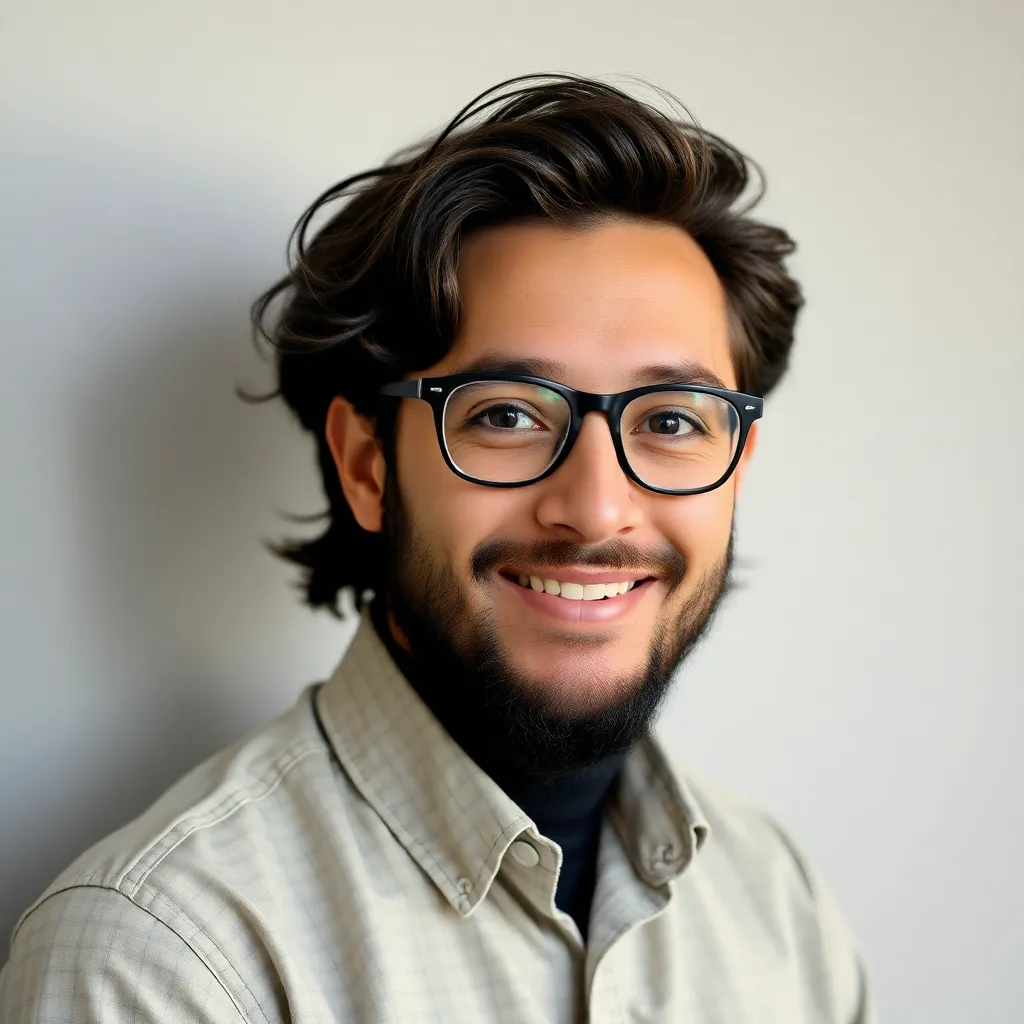
listenit
May 12, 2025 · 6 min read
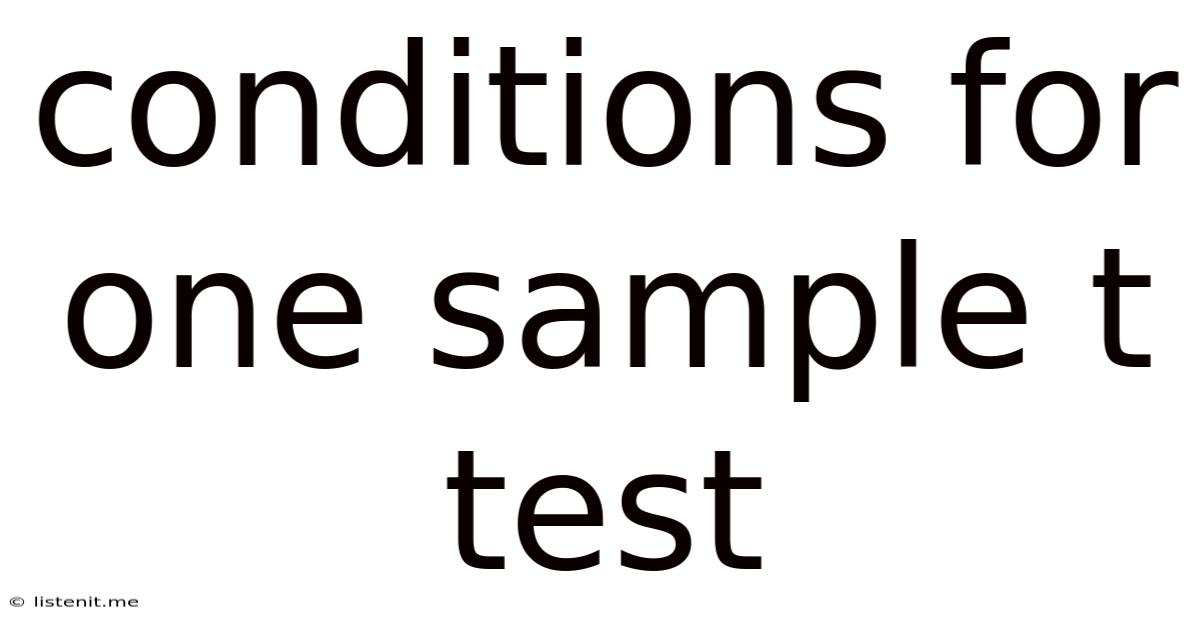
Table of Contents
Conditions for a One-Sample t-Test: A Comprehensive Guide
The one-sample t-test is a fundamental statistical procedure used to determine whether a sample mean significantly differs from a known or hypothesized population mean. While seemingly straightforward, the accuracy and validity of the results hinge critically on fulfilling several key assumptions. Failing to meet these conditions can lead to inaccurate conclusions and unreliable inferences. This article provides a comprehensive overview of these conditions, explaining their importance and offering strategies for addressing potential violations.
Understanding the One-Sample t-Test
Before delving into the assumptions, let's briefly recap the purpose of the one-sample t-test. This test is employed when you have a single sample of data and want to compare its mean to a pre-specified value (often a population mean from prior research or a theoretical value). The t-test assesses whether the observed difference between the sample mean and the hypothesized population mean is statistically significant, meaning it's unlikely to have occurred by random chance alone.
The test generates a t-statistic, which is then compared to a critical value from the t-distribution. This comparison determines whether to reject the null hypothesis (that there is no significant difference between the sample mean and the hypothesized population mean) or fail to reject it.
Crucial Assumptions of the One-Sample t-Test
The reliability and validity of the one-sample t-test depend heavily on the satisfaction of several key assumptions. These assumptions relate to the nature of the data and the sampling process. Let's explore each assumption in detail:
1. Data Follows a Normal Distribution (or Approximate Normality)
This is arguably the most crucial assumption. The one-sample t-test relies on the sampling distribution of the mean being approximately normally distributed. While the Central Limit Theorem suggests that even with non-normal populations, the sampling distribution of the mean tends towards normality as the sample size increases (generally, n ≥ 30 is considered sufficient), it's best practice to ensure the data itself is reasonably close to a normal distribution, particularly with smaller sample sizes.
How to Assess Normality:
- Visual Inspection: Histograms, Q-Q plots (quantile-quantile plots), and box plots are valuable visual tools. A histogram should resemble a bell-curve. In a Q-Q plot, points should fall approximately along a straight diagonal line.
- Statistical Tests: Formal tests like the Shapiro-Wilk test or the Kolmogorov-Smirnov test can assess normality statistically. However, these tests can be sensitive to sample size, and a large sample might show a statistically significant deviation from normality even if the deviation is practically insignificant.
What to do if Normality is Violated:
- Increase Sample Size: A larger sample size often mitigates the impact of non-normality.
- Transform the Data: Applying transformations like logarithmic, square root, or inverse transformations can sometimes normalize skewed data.
- Use a Non-parametric Test: If normality cannot be achieved, consider using a non-parametric alternative like the Wilcoxon signed-rank test, which doesn't assume normality. This test compares the medians instead of the means.
2. Random Sampling
The sample data must be obtained through a random sampling process. This ensures that the sample is representative of the population and avoids bias. Systematic biases in sampling can invalidate the results, leading to inaccurate conclusions.
Ensuring Random Sampling:
- Proper Sampling Methods: Employ appropriate random sampling techniques like simple random sampling, stratified random sampling, or cluster sampling, depending on the study design.
- Avoid Selection Bias: Carefully consider potential sources of selection bias and take steps to minimize them.
3. Independence of Observations
The observations within the sample must be independent of each other. This means that the value of one observation does not influence the value of another. Violation of independence often occurs in time-series data or clustered data where observations are related.
Addressing Independence Issues:
- Careful Study Design: Design the study to ensure independence between observations.
- Appropriate Statistical Models: If independence is violated, more complex statistical models, such as those accounting for autocorrelation in time-series data, might be necessary.
4. Data Represents a Continuous or Ordinal Variable
The one-sample t-test is primarily designed for continuous data, where the variable can take on any value within a range. It can also be applied to ordinal data (data with a rank order), provided that the data approximates a normal distribution. It is not suitable for nominal data (categorical data with no inherent order).
5. Homogeneity of Variance (for some variations of the test)
While the standard one-sample t-test doesn't explicitly require homogeneity of variance (as it's comparing a sample to a single known population mean), some variations or extensions might have this requirement. If you are using a modified version of the t-test that incorporates a separate estimate of population variance or confidence intervals, then homogeneity of variance might be assumed.
Consequences of Violating Assumptions
Ignoring the assumptions of the one-sample t-test can have significant repercussions:
- Type I Error: Violating assumptions, particularly the normality assumption, can increase the probability of a Type I error – incorrectly rejecting the null hypothesis when it is actually true. This leads to false positive conclusions.
- Inflated Type II Error: Violations might also lead to an increased probability of a Type II error – failing to reject the null hypothesis when it is false. This results in missing a true effect.
- Invalid p-values: Incorrect application of the t-test due to assumption violations will produce unreliable p-values, making it difficult to interpret statistical significance.
- Unreliable Confidence Intervals: The confidence intervals calculated from the t-test will not accurately reflect the true population mean if the assumptions are violated.
Robustness of the One-Sample t-Test
It is important to note that the one-sample t-test is relatively robust to minor violations of the normality assumption, especially when the sample size is large (n ≥ 30). The Central Limit Theorem helps ensure that the sampling distribution of the mean remains approximately normal even if the underlying data is slightly non-normal. However, for smaller samples or substantial deviations from normality, the robustness diminishes, and the consequences mentioned above become more likely.
Conclusion
The one-sample t-test is a powerful tool for comparing a sample mean to a known population mean. However, its accurate application depends on fulfilling specific assumptions. Careful attention to these conditions – normality, random sampling, independence, data type, and potentially homogeneity of variance – is crucial for ensuring reliable and valid results. When assumptions are violated, appropriate remedial measures, such as data transformations or employing non-parametric alternatives, should be considered to maintain the integrity of the statistical analysis. Always carefully assess the data and consider the potential consequences of violating the assumptions before interpreting the results of a one-sample t-test. By understanding these conditions and applying them correctly, researchers can draw accurate and meaningful inferences from their data.
Latest Posts
Latest Posts
-
How Did The Wib Help America
May 13, 2025
-
What Are The Equivalent Fractions For 2 5
May 13, 2025
-
Can The Remainder Be Greater Than The Divisor
May 13, 2025
-
The Focal Length Of A Converging Lens Is
May 13, 2025
-
Is Zinc Carbonate Soluble In Water
May 13, 2025
Related Post
Thank you for visiting our website which covers about Conditions For One Sample T Test . We hope the information provided has been useful to you. Feel free to contact us if you have any questions or need further assistance. See you next time and don't miss to bookmark.