Conceptual Adaptation Using Knowledge Graph And Semantics Ai
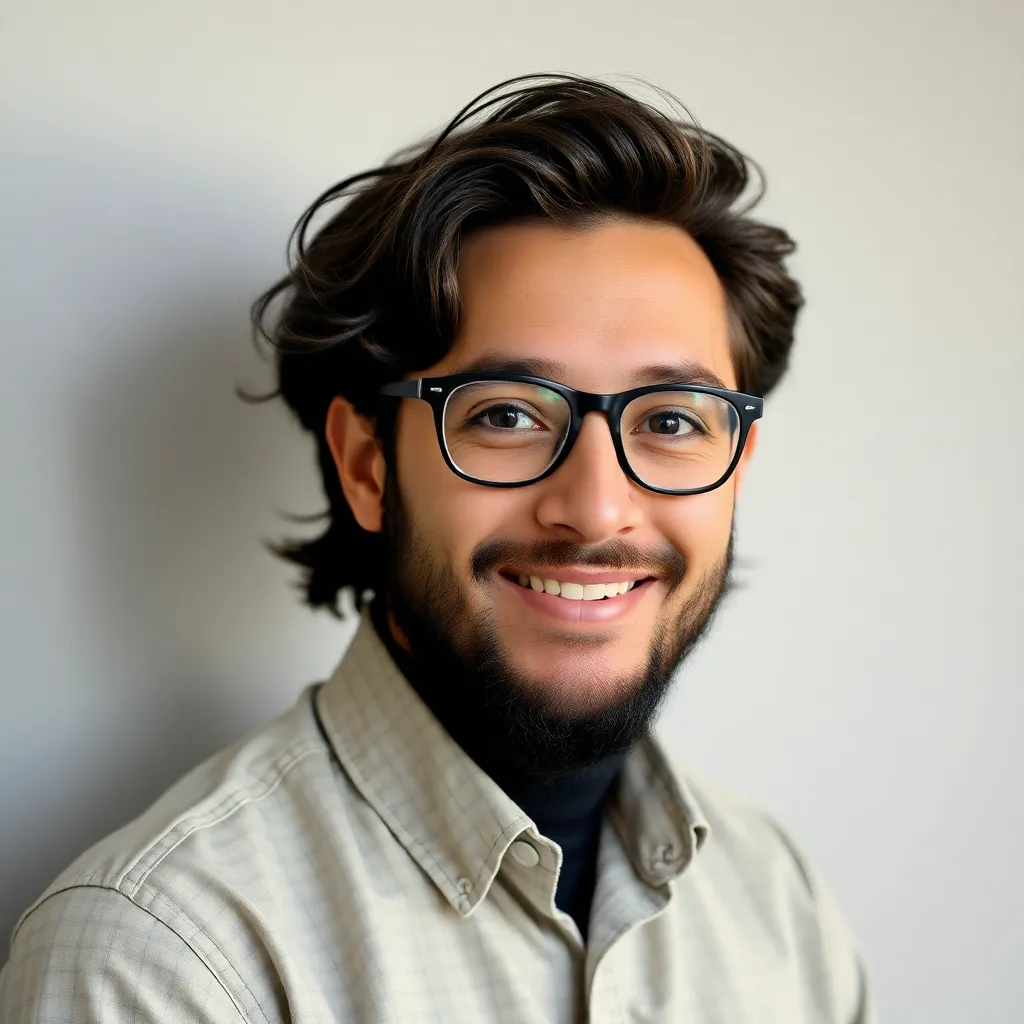
listenit
May 27, 2025 · 6 min read
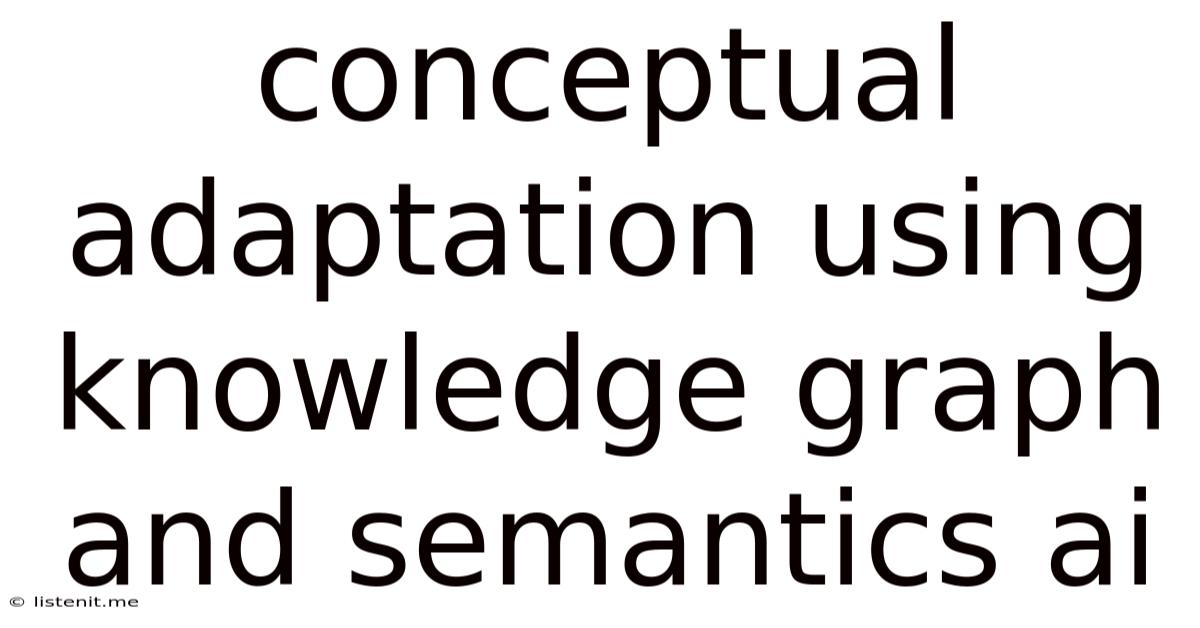
Table of Contents
Conceptual Adaptation Using Knowledge Graphs and Semantic AI
The digital age has ushered in an era of unprecedented data growth. This explosion of information presents both incredible opportunities and significant challenges. Harnessing this data effectively requires sophisticated techniques capable of understanding not just the raw facts, but also the underlying concepts and relationships between them. This is where conceptual adaptation, powered by knowledge graphs and semantic AI, comes into play. This article delves deep into this rapidly evolving field, exploring its core principles, practical applications, and future prospects.
Understanding Conceptual Adaptation
Conceptual adaptation refers to the ability of a system to dynamically adjust its understanding and behavior based on new or evolving conceptual information. Unlike traditional systems that operate on rigid, pre-defined rules, conceptually adaptive systems exhibit flexibility and resilience in the face of changing contexts. This adaptability is crucial in numerous domains where knowledge is constantly evolving, ambiguous, or incomplete.
Imagine a chatbot designed to answer customer queries about a company's products. A traditional chatbot might rely on a predefined set of rules and keywords. However, a conceptually adaptive chatbot, leveraging knowledge graphs and semantic AI, can dynamically understand the user's intent even if the phrasing is unconventional or contains nuanced language. It can leverage contextual information to provide more accurate and relevant responses.
The Role of Knowledge Graphs
Knowledge graphs are structured representations of information, organized as a network of interconnected nodes and edges. Nodes represent entities (e.g., people, places, things, concepts), while edges represent relationships between these entities. This structured approach enables machines to understand the meaning and context of information much more effectively than unstructured text or databases.
Key benefits of using knowledge graphs in conceptual adaptation include:
- Enhanced Contextual Understanding: Knowledge graphs provide a rich context for interpreting information, enabling systems to disambiguate meaning and resolve ambiguities.
- Improved Reasoning and Inference: By analyzing the relationships between entities, systems can perform logical reasoning and infer new knowledge.
- Facilitated Knowledge Discovery: Knowledge graphs allow for efficient searching and retrieval of information, enabling users to easily uncover relevant knowledge.
- Scalability and Maintainability: The structured nature of knowledge graphs makes them highly scalable and maintainable, facilitating the integration of new information.
The Power of Semantic AI
Semantic AI focuses on enabling computers to understand and process information in a way that mirrors human understanding. This involves not only processing the literal meaning of words but also grasping their underlying concepts, relationships, and intentions. Semantic technologies, such as Natural Language Processing (NLP) and ontology engineering, are fundamental to building conceptually adaptive systems.
Key semantic AI techniques used in conceptual adaptation include:
- Natural Language Understanding (NLU): NLU allows systems to understand the meaning and intent of human language, even in the presence of ambiguity or incomplete information.
- Ontology Engineering: Ontology engineering involves creating formal representations of knowledge, defining concepts, and specifying relationships between them. These ontologies provide the backbone for knowledge graphs.
- Reasoning and Inference: Semantic AI techniques enable systems to perform logical reasoning and infer new knowledge based on existing information within the knowledge graph.
- Machine Learning (ML): ML algorithms can be trained on knowledge graphs to improve the accuracy and efficiency of conceptual adaptation. For instance, ML can help refine the system's understanding of relationships between entities or predict the user's intent.
Applications of Conceptual Adaptation
Conceptual adaptation, powered by knowledge graphs and semantic AI, is finding applications across a wide range of domains, including:
1. Customer Service and Support
As mentioned earlier, conceptually adaptive chatbots can deliver significantly improved customer service. By understanding the context of a customer's query, they can provide more accurate and personalized responses, even if the query is phrased informally or contains errors.
2. Personalized Recommendations
E-commerce platforms can utilize knowledge graphs to understand customer preferences and behaviors, leading to more relevant product recommendations. By connecting products with their features, user reviews, and other contextual data, the system can adapt to individual customer needs.
3. Healthcare
In healthcare, conceptual adaptation can assist in diagnosis and treatment planning. Knowledge graphs can integrate patient data, medical literature, and clinical guidelines to provide clinicians with more comprehensive insights. This can lead to more accurate diagnoses and more effective treatment strategies.
4. Finance
Financial institutions use conceptual adaptation for fraud detection and risk management. Knowledge graphs can link various data points, such as transaction history, customer profiles, and market data, to identify suspicious patterns and mitigate risks.
5. Education
Adaptive learning platforms leverage conceptual adaptation to personalize the learning experience. By analyzing a student's performance and understanding their learning style, the system can adapt the curriculum and learning materials to meet their individual needs.
Challenges and Future Directions
While the potential of conceptual adaptation is immense, several challenges remain:
1. Data Sparsity and Incompleteness
Knowledge graphs often suffer from data sparsity and incompleteness, particularly in specialized domains. Addressing this requires efficient methods for knowledge acquisition and integration.
2. Knowledge Representation and Reasoning
Developing effective knowledge representation schemes and reasoning techniques is crucial for accurate conceptual adaptation. This requires ongoing research in ontology engineering and semantic reasoning.
3. Scalability and Performance
Building scalable and high-performing conceptually adaptive systems is a significant challenge, requiring optimization techniques for knowledge graph management and semantic reasoning.
4. Explainability and Trust
Understanding how conceptually adaptive systems arrive at their conclusions is crucial for building trust. Developing methods for explaining the system's reasoning process is essential for wider adoption.
Future directions for research and development include:
- Development of more robust and scalable knowledge graph technologies. This includes efficient methods for knowledge acquisition, integration, and management.
- Advancements in semantic reasoning techniques. This involves developing more sophisticated methods for performing logical reasoning and inference on knowledge graphs.
- Integration of machine learning with knowledge graphs. This allows for the development of more accurate and efficient conceptual adaptation systems.
- Development of explainable AI (XAI) techniques for conceptual adaptation. This is crucial for building trust and transparency in these systems.
- Exploration of new applications for conceptual adaptation. The potential applications of this technology are vast, and ongoing research will continue to uncover new possibilities.
Conclusion
Conceptual adaptation, powered by the synergy between knowledge graphs and semantic AI, represents a significant advancement in artificial intelligence. By enabling systems to dynamically adapt their understanding and behavior in response to new information, it opens up a world of possibilities across various domains. While challenges remain, ongoing research and development are paving the way for more robust, scalable, and explainable conceptually adaptive systems. The future of AI lies in its ability to not only process information but also understand and reason with it, and conceptual adaptation is a key step in this direction. As we continue to generate and collect more data, the importance of conceptual adaptation will only continue to grow, unlocking further innovation and empowering us to make sense of the ever-increasing complexity of the world around us.
Latest Posts
Latest Posts
-
Secretion Of Potassium Into The Urine Is
May 28, 2025
-
Where Did The Boer Goat Originate
May 28, 2025
-
Half Lives To Achieve Steady State
May 28, 2025
-
How To Calculate Annual Loss Expectancy
May 28, 2025
-
Transient Neurologic Deficit After A Seizure
May 28, 2025
Related Post
Thank you for visiting our website which covers about Conceptual Adaptation Using Knowledge Graph And Semantics Ai . We hope the information provided has been useful to you. Feel free to contact us if you have any questions or need further assistance. See you next time and don't miss to bookmark.