Chi Squared Test For Homogeneity Vs Independence
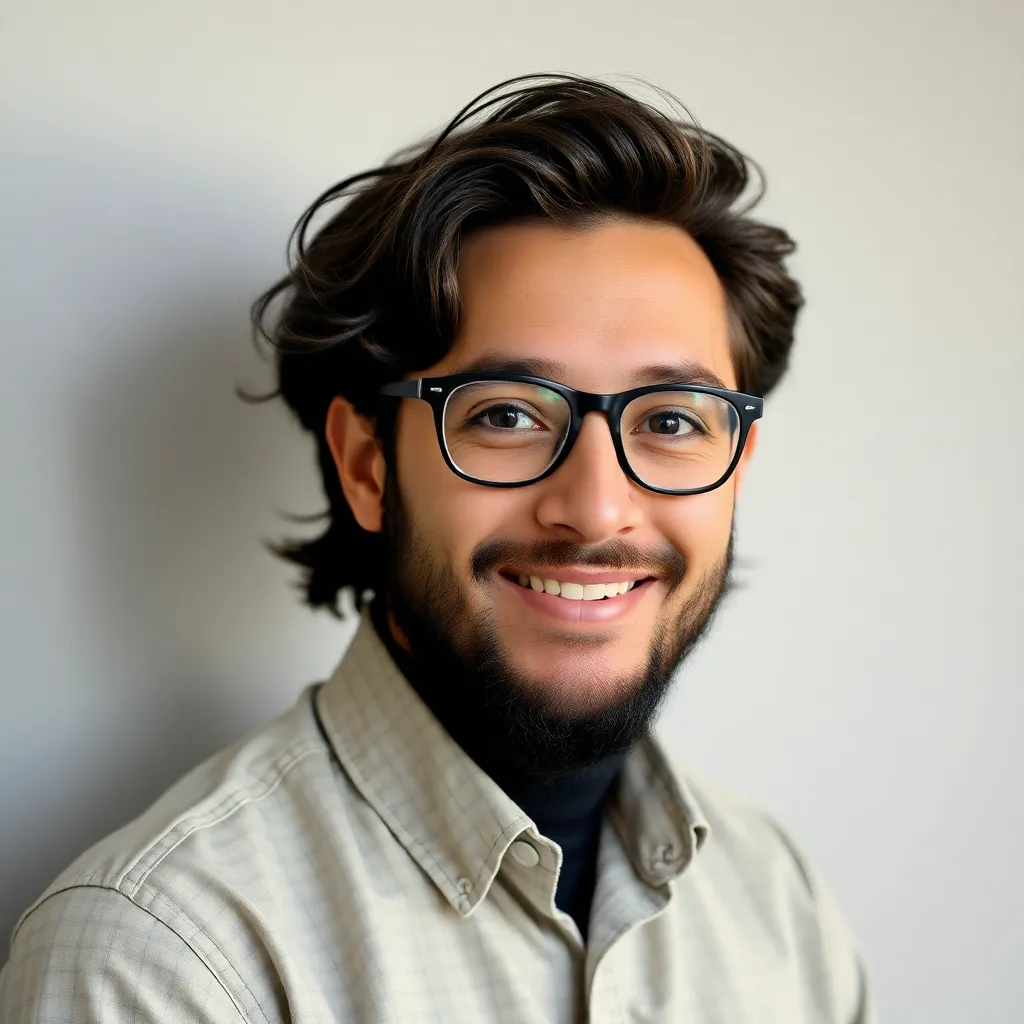
listenit
May 10, 2025 · 7 min read
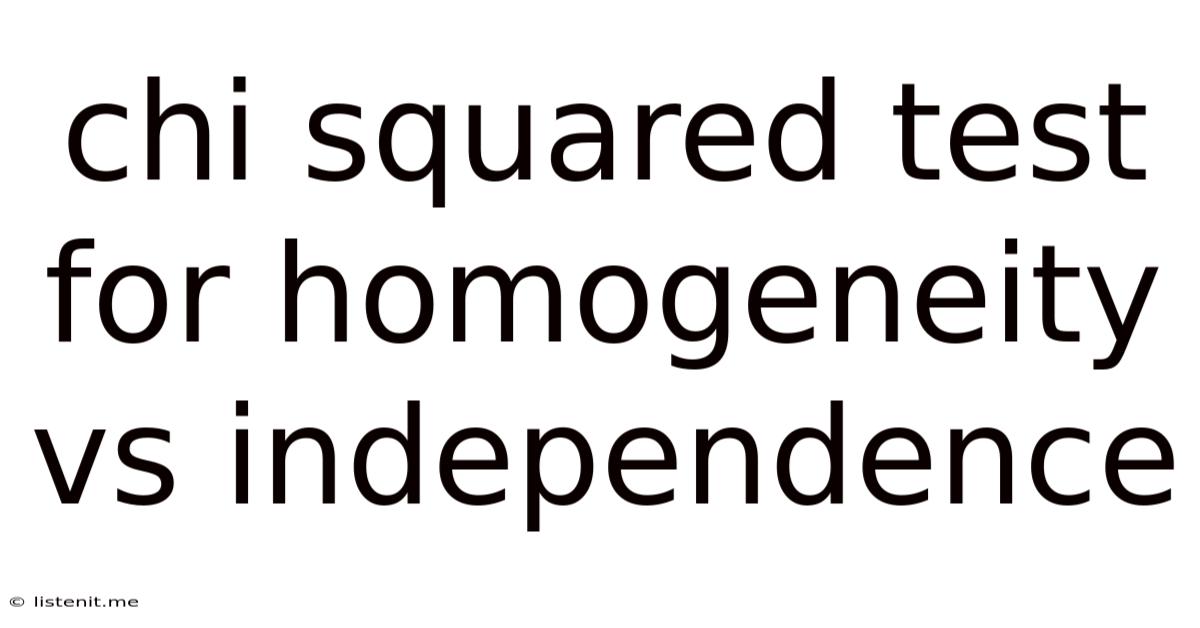
Table of Contents
Chi-Squared Test: Homogeneity vs. Independence – A Deep Dive
The chi-squared test is a powerful statistical tool used to analyze categorical data. It helps determine if there's a significant association between different categories or if the distribution of a categorical variable differs significantly across different groups. However, two distinct types of chi-squared tests often cause confusion: the test of independence and the test of homogeneity. While both use the same fundamental chi-squared statistic, they address different research questions and have subtle differences in their interpretation. This article will clarify the distinctions between these two tests, providing a comprehensive understanding of their applications and interpretations.
Understanding the Chi-Squared Test Fundamentals
Before delving into the differences, let's establish a common foundation. Both the chi-squared test of independence and the chi-squared test of homogeneity analyze categorical data presented in a contingency table (also known as a cross-tabulation). A contingency table displays the frequency counts of observations across different categories of two or more variables. The core idea is to compare the observed frequencies in the contingency table to the frequencies we would expect if there were no association between the variables (or no difference in distributions).
The chi-squared statistic (χ²) measures the discrepancy between the observed and expected frequencies. A larger χ² value suggests a greater difference between observed and expected frequencies, indicating a stronger association or a more significant difference in distributions. This value is then compared to a critical value from the chi-squared distribution, determined by the degrees of freedom and the chosen significance level (usually 0.05). If the calculated χ² exceeds the critical value, we reject the null hypothesis.
Chi-Squared Test of Independence
The chi-squared test of independence investigates whether two categorical variables are independent. The null hypothesis (H₀) states that there is no association between the two variables; they are independent. The alternative hypothesis (H₁) states that there is an association between the variables; they are not independent.
Example: Let's say we're investigating the relationship between smoking status (smoker/non-smoker) and lung cancer diagnosis (yes/no). We collect data and create a contingency table:
Lung Cancer (Yes) | Lung Cancer (No) | Total | |
---|---|---|---|
Smoker | 150 | 50 | 200 |
Non-Smoker | 50 | 150 | 200 |
Total | 200 | 200 | 400 |
A chi-squared test of independence would determine if smoking status and lung cancer diagnosis are independent. If the test yields a significant result (p-value < 0.05), we reject the null hypothesis and conclude there is a statistically significant association between smoking and lung cancer.
Key Features of the Test of Independence:
- One sample: The data comes from a single sample. We're analyzing the relationship between two variables within that sample.
- Sampling method: The sampling method used doesn't directly affect the test's interpretation, as long as it's appropriate for the data.
- Research question: Does an association exist between two categorical variables?
Chi-Squared Test of Homogeneity
The chi-squared test of homogeneity examines whether the distribution of a single categorical variable is the same across different populations or groups. The null hypothesis (H₀) states that the distribution of the categorical variable is the same across all groups. The alternative hypothesis (H₁) states that the distribution is not the same across all groups.
Example: Let's consider the same lung cancer example, but now we have data from three different cities: City A, City B, and City C. We want to determine if the proportion of smokers is the same across these three cities. Our contingency table would look like this:
City A | City B | City C | Total | |
---|---|---|---|---|
Smoker | 60 | 70 | 70 | 200 |
Non-Smoker | 140 | 130 | 130 | 400 |
Total | 200 | 200 | 200 | 600 |
A chi-squared test of homogeneity would assess whether the distribution of smoking status (smoker/non-smoker) is consistent across the three cities. A significant result (p-value < 0.05) would indicate that the proportion of smokers differs significantly across at least two of the cities.
Key Features of the Test of Homogeneity:
- Multiple samples: The data comes from multiple independent samples (e.g., different cities, age groups, etc.).
- Sampling method: The sampling method is crucial; samples should be representative of their respective populations.
- Research question: Is the distribution of a categorical variable consistent across different groups?
Key Differences Summarized
Feature | Test of Independence | Test of Homogeneity |
---|---|---|
Objective | Determine association between two variables | Compare distributions across multiple groups |
Number of Samples | One | Multiple |
Null Hypothesis | No association between variables | Same distribution across groups |
Data Structure | Two categorical variables | One categorical variable, multiple groups |
Example | Relationship between smoking and cancer | Smoking prevalence across different cities |
Assumptions and Limitations
Both tests rely on certain assumptions:
- Independence of observations: Observations within each group must be independent.
- Expected frequencies: Expected cell frequencies should be sufficiently large (generally, at least 5 in each cell). This ensures the chi-squared approximation is valid. If expected frequencies are too low, Fisher's exact test might be a more appropriate alternative.
- Data type: The data must be categorical.
- Random sampling: The data should be collected through random sampling, especially for the test of homogeneity.
Ignoring these assumptions can lead to inaccurate or misleading results.
Interpreting the Results
The p-value from the chi-squared test is crucial for interpretation. If the p-value is less than the significance level (typically 0.05), we reject the null hypothesis. For the test of independence, this implies a statistically significant association between the variables. For the test of homogeneity, it suggests a statistically significant difference in the distribution of the categorical variable across the groups. It's important to remember that statistical significance doesn't necessarily imply practical significance. The magnitude of the association or difference should also be considered.
Effect Size Measures
While the p-value indicates statistical significance, it doesn't quantify the strength of the association or difference. Several effect size measures can provide this information:
- Cramer's V: For the test of independence, Cramer's V measures the strength of association between two categorical variables. It ranges from 0 (no association) to 1 (perfect association).
- Phi coefficient: A specific case of Cramer's V used when both variables have only two categories.
- Contingency coefficient: Another measure of association, though it's not bounded by 1.
These measures provide a more comprehensive interpretation of the results, complementing the p-value.
Choosing the Right Test
The choice between the chi-squared test of independence and the test of homogeneity depends on the research question and the structure of the data. If you're investigating the relationship between two categorical variables within a single sample, use the test of independence. If you're comparing the distribution of a single categorical variable across multiple groups, use the test of homogeneity.
Conclusion
The chi-squared test is a fundamental tool for analyzing categorical data. Understanding the distinction between the test of independence and the test of homogeneity is crucial for appropriate application and accurate interpretation. By carefully considering the research question, data structure, and assumptions, researchers can effectively use the chi-squared test to draw meaningful conclusions from categorical data and contribute to robust scientific findings. Remember to always consider effect size measures alongside p-values for a more complete understanding of your results. Proper application and interpretation of these tests are essential for valid statistical inference.
Latest Posts
Latest Posts
-
Does Index Of Refraction Have Units
May 10, 2025
-
Bond Order Of No In No3
May 10, 2025
-
How To Find Range Of Square Root Function
May 10, 2025
-
How To Find The Equation Of The Perpendicular Bisector
May 10, 2025
-
Do Bacteria Contain Dna Or Rna
May 10, 2025
Related Post
Thank you for visiting our website which covers about Chi Squared Test For Homogeneity Vs Independence . We hope the information provided has been useful to you. Feel free to contact us if you have any questions or need further assistance. See you next time and don't miss to bookmark.