Chi Square Test Of Independence Vs Homogeneity
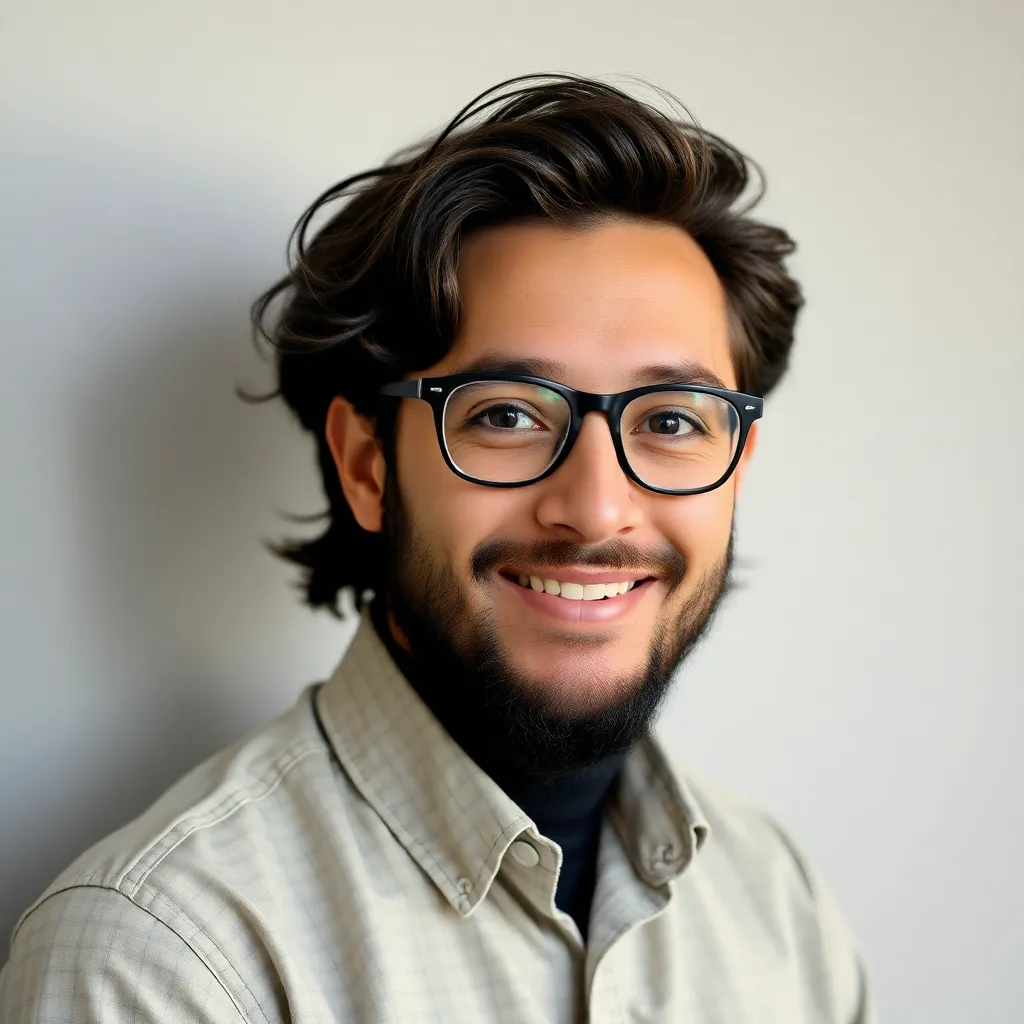
listenit
May 11, 2025 · 7 min read
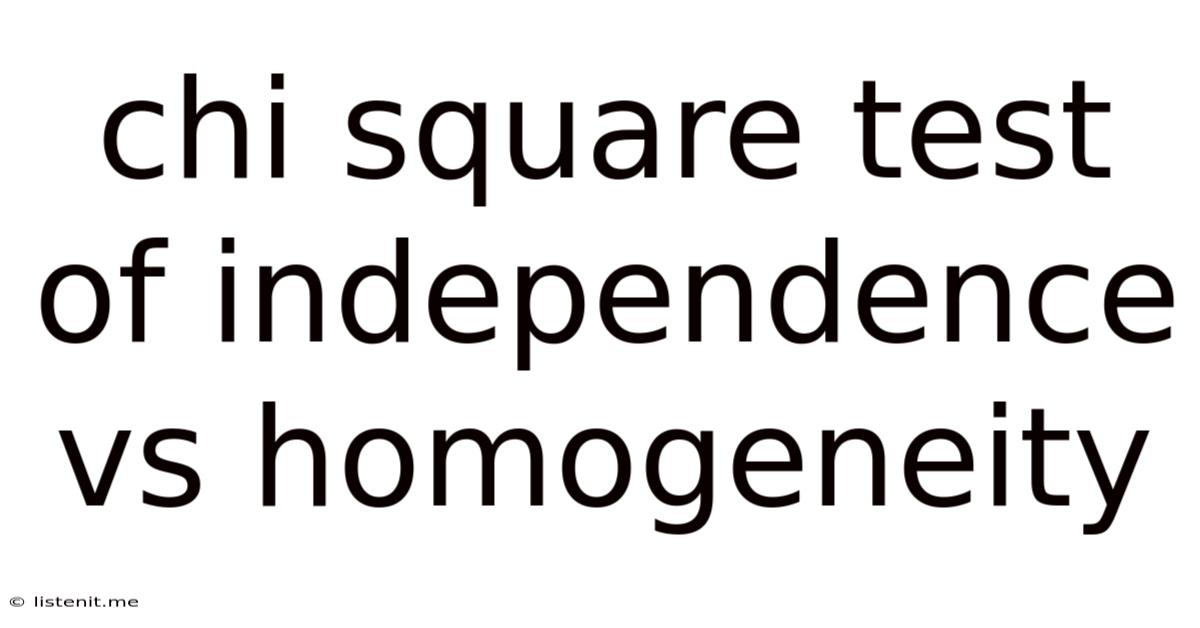
Table of Contents
Chi-Square Test of Independence vs. Homogeneity: A Comprehensive Guide
The chi-square test is a powerful statistical tool used to analyze categorical data. It allows us to determine if there's a significant association between two categorical variables. However, there are two distinct types of chi-square tests: the test of independence and the test of homogeneity. While both use the same chi-square statistic, they differ subtly in their hypotheses and applications. Understanding these differences is crucial for correctly interpreting results and drawing accurate conclusions. This comprehensive guide will delve into the nuances of each test, highlighting their similarities, differences, and practical applications.
Understanding Categorical Data and the Chi-Square Test
Before diving into the specifics of independence and homogeneity tests, let's establish a foundational understanding of categorical data and the chi-square test itself. Categorical data represents characteristics or qualities, rather than numerical quantities. Examples include gender (male/female), eye color (brown, blue, green), or political affiliation (Democrat, Republican, Independent).
The chi-square test assesses whether the observed frequencies of categorical data differ significantly from the expected frequencies. This comparison helps determine if there's a relationship between the variables under consideration. The test statistic, denoted as χ², follows a chi-square distribution, whose shape depends on the degrees of freedom. A higher χ² value indicates a greater discrepancy between observed and expected frequencies, suggesting a stronger relationship. The p-value associated with the χ² value determines the statistical significance of the results. A low p-value (typically below 0.05) indicates that the observed differences are unlikely due to chance alone, leading to the rejection of the null hypothesis.
The Chi-Square Test of Independence
The chi-square test of independence investigates whether two categorical variables are independent of each other within a single population. The null hypothesis (H₀) states that there is no association between the two variables; they are independent. The alternative hypothesis (H₁) states that there is an association between the variables; they are not independent.
Hypotheses:
- H₀ (Null Hypothesis): The two categorical variables are independent.
- H₁ (Alternative Hypothesis): The two categorical variables are not independent.
Example:
Let's say we want to investigate whether there's a relationship between smoking status (smoker/non-smoker) and lung cancer (yes/no). We collect data from a sample of individuals and create a contingency table showing the frequencies of each combination of smoking status and lung cancer diagnosis. The chi-square test of independence helps determine if smoking status and lung cancer are independent or if there's a statistically significant association between them.
Conducting the Chi-Square Test of Independence
- State the Hypotheses: Clearly define the null and alternative hypotheses.
- Set the Significance Level (α): This is typically set at 0.05.
- Create a Contingency Table: Organize the observed frequencies into a contingency table.
- Calculate Expected Frequencies: For each cell in the contingency table, calculate the expected frequency using the formula:
(row total * column total) / grand total
. - Calculate the Chi-Square Statistic (χ²): Use the formula: χ² = Σ [(Observed frequency - Expected frequency)² / Expected frequency].
- Determine the Degrees of Freedom: df = (number of rows - 1) * (number of columns - 1).
- Find the p-value: Using the χ² value and degrees of freedom, find the p-value from a chi-square distribution table or statistical software.
- Make a Decision: If the p-value is less than the significance level (α), reject the null hypothesis. If the p-value is greater than α, fail to reject the null hypothesis.
The Chi-Square Test of Homogeneity
The chi-square test of homogeneity examines whether the distribution of a single categorical variable is the same across different populations or groups. The null hypothesis (H₀) posits that the distribution of the categorical variable is the same across all groups. The alternative hypothesis (H₁) suggests that the distributions are different across at least one pair of groups.
Hypotheses:
- H₀ (Null Hypothesis): The distribution of the categorical variable is the same across all populations.
- H₁ (Alternative Hypothesis): The distribution of the categorical variable is not the same across all populations.
Example:
Suppose we want to compare the proportion of individuals who prefer different brands of coffee (Brand A, Brand B, Brand C) across three different age groups (18-30, 31-50, 51+). The chi-square test of homogeneity would determine if the distribution of coffee brand preferences is consistent across these age groups.
Conducting the Chi-Square Test of Homogeneity
The procedure for conducting the test of homogeneity is very similar to the test of independence:
- State the Hypotheses: Clearly define the null and alternative hypotheses.
- Set the Significance Level (α): Typically set at 0.05.
- Create a Contingency Table: Organize the observed frequencies into a contingency table.
- Calculate Expected Frequencies: The calculation of expected frequencies is identical to the test of independence:
(row total * column total) / grand total
. - Calculate the Chi-Square Statistic (χ²): The formula remains the same: χ² = Σ [(Observed frequency - Expected frequency)² / Expected frequency].
- Determine the Degrees of Freedom: df = (number of rows - 1) * (number of columns - 1).
- Find the p-value: Use the χ² value and degrees of freedom to find the p-value.
- Make a Decision: If the p-value is less than α, reject the null hypothesis. If the p-value is greater than α, fail to reject the null hypothesis.
Key Differences Between Tests of Independence and Homogeneity
While both tests utilize the same chi-square statistic and calculation procedures, a crucial distinction lies in how the data is sampled and the nature of the hypotheses being tested:
-
Sampling: The test of independence analyzes data from a single sample where observations are classified according to two categorical variables. The test of homogeneity analyzes data from multiple independent samples, each representing a different population, where observations are classified according to a single categorical variable.
-
Hypotheses: The test of independence investigates the association between two variables within a single population. The test of homogeneity examines the equality of distributions of a single variable across different populations.
-
Focus: The test of independence focuses on the relationship between two variables. The test of homogeneity focuses on comparing distributions across groups.
Assumptions of the Chi-Square Test
Both tests of independence and homogeneity rely on several assumptions for valid results:
- Random Sampling: The data should be obtained through a random sampling process.
- Independence of Observations: Observations within each sample should be independent of each other.
- Expected Frequencies: Expected frequencies in each cell of the contingency table should be sufficiently large (generally, at least 5). If this assumption is violated, alternative methods like Fisher's exact test might be more appropriate.
- Categorical Data: The data should be categorical.
Choosing Between Tests of Independence and Homogeneity
The choice between a test of independence and a test of homogeneity depends on the research question and how the data was collected. If you are investigating the relationship between two categorical variables within a single sample, use the test of independence. If you are comparing the distributions of a single categorical variable across multiple independent samples, use the test of homogeneity.
Practical Applications and Interpretations
Chi-square tests find widespread applications in various fields, including:
- Medical Research: Investigating the association between risk factors (e.g., smoking, diet) and disease outcomes.
- Market Research: Analyzing consumer preferences and brand loyalty across demographic groups.
- Social Sciences: Studying the relationship between social factors (e.g., education, income) and attitudes or behaviors.
- Environmental Science: Examining the distribution of species across different habitats.
The interpretation of the results involves considering the p-value and the effect size. A statistically significant result (p-value < α) suggests evidence against the null hypothesis, indicating a relationship (independence test) or difference in distributions (homogeneity test). However, a statistically significant result doesn't necessarily imply a strong effect. Examining measures of effect size, such as Cramer's V or Phi coefficient, can provide further insights into the strength of the association or difference.
Conclusion
The chi-square test, encompassing both tests of independence and homogeneity, is a fundamental tool for analyzing categorical data. Understanding the subtle yet crucial differences between these two tests is vital for proper application and accurate interpretation of results. By carefully considering the research question, sampling method, and assumptions, researchers can effectively utilize the chi-square test to draw meaningful conclusions from categorical data and gain valuable insights across various domains. Remember to always consider the context of your data and the practical implications of your findings when interpreting the results of a chi-square test. The p-value should be interpreted in conjunction with effect size and subject matter expertise to provide a complete and nuanced understanding of your findings.
Latest Posts
Latest Posts
-
Why Do Electric Field Lines Never Cross
May 13, 2025
-
1 10 As A Percent And Decimal
May 13, 2025
-
Can All Minerals Be A Gemstone
May 13, 2025
-
Multicellular Heterotrophs Without A Cell Wall
May 13, 2025
-
What Are The Gcf Of 48
May 13, 2025
Related Post
Thank you for visiting our website which covers about Chi Square Test Of Independence Vs Homogeneity . We hope the information provided has been useful to you. Feel free to contact us if you have any questions or need further assistance. See you next time and don't miss to bookmark.