Chi Square Test Of Homogeneity Vs Independence
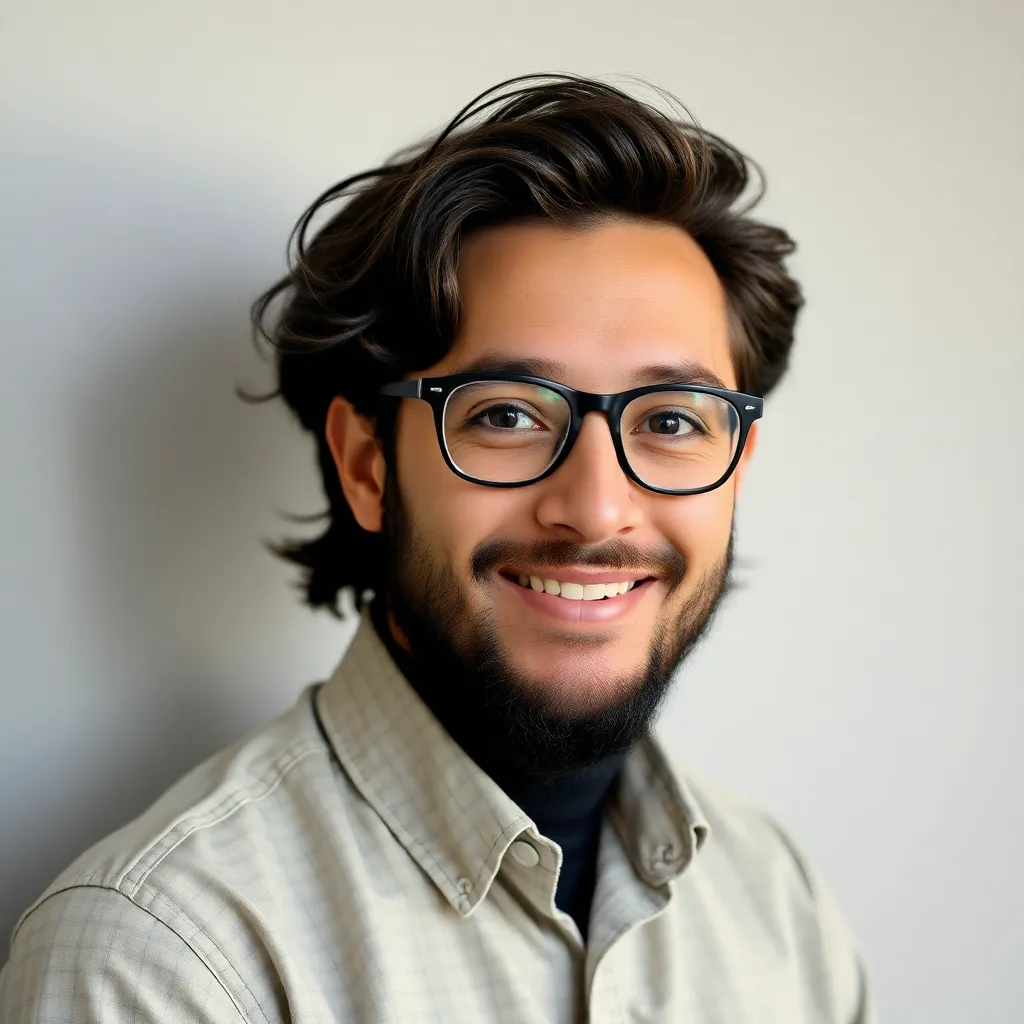
listenit
Apr 27, 2025 · 6 min read

Table of Contents
Chi-Square Test: Homogeneity vs. Independence – A Deep Dive
The chi-square test is a powerful statistical tool used to analyze categorical data. It helps us determine if there's a significant association between two categorical variables. However, there are two distinct types of chi-square tests often confused: the test of independence and the test of homogeneity. While both use the same core calculation, they address different research questions and have subtle yet important distinctions. This comprehensive guide will clarify these differences, delve into their applications, and equip you with the knowledge to choose the right test for your data analysis.
Understanding Categorical Data and the Chi-Square Test
Before diving into the nuances of independence and homogeneity, let's establish a foundation. Categorical data represents qualities or characteristics, not numerical values. Think of eye color (blue, brown, green), gender (male, female), or political affiliation (Democrat, Republican, Independent). The chi-square test assesses whether the observed frequencies in different categories differ significantly from what we'd expect under a specific hypothesis. This "expectation" is crucial and is what distinguishes the two tests.
The Chi-Square Test of Independence
The chi-square test of independence examines whether two categorical variables are independent of each other within a single population. In simpler terms, it asks: Is there a relationship between these two variables? Does knowing the value of one variable give us any information about the likely value of the other?
Example: Let's say we want to investigate if there's a relationship between smoking status (smoker, non-smoker) and the development of lung cancer (yes, no). We collect data from a single population and create a contingency table:
Lung Cancer (Yes) | Lung Cancer (No) | Total | |
---|---|---|---|
Smoker | 100 | 200 | 300 |
Non-Smoker | 20 | 780 | 800 |
Total | 120 | 980 | 1100 |
The chi-square test helps us determine if the observed frequencies in this table differ significantly from what we'd expect if smoking and lung cancer were truly independent. If there's a significant association, it suggests a relationship between smoking and lung cancer risk.
Steps Involved in a Chi-Square Test of Independence:
-
State the hypotheses: The null hypothesis (H₀) is that the two variables are independent. The alternative hypothesis (H₁) is that they are dependent.
-
Set the significance level (alpha): This is typically set at 0.05, meaning we're willing to accept a 5% chance of rejecting the null hypothesis when it's actually true (Type I error).
-
Calculate the expected frequencies: For each cell in the contingency table, the expected frequency is calculated based on the assumption of independence: (Row Total * Column Total) / Grand Total.
-
Calculate the chi-square statistic: This involves summing the squared differences between observed and expected frequencies, divided by the expected frequencies for each cell.
-
Determine the degrees of freedom: This is calculated as (number of rows - 1) * (number of columns - 1).
-
Find the p-value: Using the chi-square statistic and degrees of freedom, we look up the p-value in a chi-square distribution table or use statistical software.
-
Make a decision: If the p-value is less than the significance level (alpha), we reject the null hypothesis and conclude there's a significant association between the variables. Otherwise, we fail to reject the null hypothesis.
The Chi-Square Test of Homogeneity
The chi-square test of homogeneity compares the distribution of a single categorical variable across different populations. It asks: Are the distributions of this variable similar across these populations? Are the proportions of different categories the same in each group?
Example: Let's say we want to compare the distribution of political affiliation (Democrat, Republican, Independent) across three different age groups (18-30, 31-50, 51+). We collect data from each age group separately and create a contingency table:
18-30 | 31-50 | 51+ | |
---|---|---|---|
Democrat | 150 | 200 | 100 |
Republican | 100 | 150 | 200 |
Independent | 50 | 50 | 100 |
The chi-square test of homogeneity helps determine if the distribution of political affiliation is the same across these three age groups. If the distributions are significantly different, it suggests that political affiliation varies across age groups.
Steps Involved in a Chi-Square Test of Homogeneity:
The steps are very similar to the test of independence:
-
State the hypotheses: The null hypothesis (H₀) is that the distribution of the categorical variable is the same across all populations. The alternative hypothesis (H₁) is that the distributions are different.
-
Set the significance level (alpha): Typically 0.05.
-
Calculate the expected frequencies: Here, the expected frequency for each cell is calculated based on the overall proportion of each category across all populations. For example, the expected number of Democrats in the 18-30 group would be calculated using the overall proportion of Democrats across all age groups and the total number of individuals in the 18-30 group.
-
Calculate the chi-square statistic: This is done using the same formula as in the test of independence.
-
Determine the degrees of freedom: (number of rows - 1) * (number of columns - 1).
-
Find the p-value: Using the chi-square statistic and degrees of freedom.
-
Make a decision: If the p-value is less than alpha, reject the null hypothesis and conclude the distributions are different.
Key Differences Summarized:
Feature | Test of Independence | Test of Homogeneity |
---|---|---|
Research Question | Is there an association between two variables within one population? | Are the distributions of one variable the same across multiple populations? |
Number of Variables | Two categorical variables | One categorical variable, multiple populations |
Sampling | Single sample from one population | Multiple samples from different populations |
Expected Frequencies Calculation | Based on marginal totals (row and column totals) | Based on overall proportions across all populations |
Choosing the Right Test:
The key to choosing the correct test lies in understanding your research question and data collection method.
-
Use the test of independence when: You have one sample and want to examine the relationship between two categorical variables within that single population.
-
Use the test of homogeneity when: You have multiple samples from different populations and want to compare the distribution of a single categorical variable across those populations.
Beyond the Basics: Assumptions and Limitations
While powerful, the chi-square test has assumptions and limitations:
-
Expected frequencies: Cells should have expected frequencies of at least 5. If not, consider combining categories or using Fisher's exact test (especially for 2x2 tables).
-
Independence of observations: Observations should be independent of each other.
-
Categorical data: The test is designed for categorical data; it's inappropriate for continuous data.
-
Large sample sizes: While the test can work with smaller samples, larger sample sizes generally lead to more reliable results.
Conclusion:
The chi-square test of independence and homogeneity are valuable tools for analyzing categorical data. Understanding their subtle yet crucial differences is essential for accurate data interpretation and drawing meaningful conclusions. By carefully considering your research question, data collection method, and the assumptions of the test, you can apply the appropriate chi-square test effectively and contribute to robust and reliable data-driven insights. Remember to always pair your statistical analysis with careful consideration of the context and potential limitations of your study design.
Latest Posts
Latest Posts
-
Why Is Energy Required For Boiling Process
Apr 27, 2025
-
How Long Is 4 Light Years In Earth Years
Apr 27, 2025
-
Salt Bridge Function In Galvanic Cell
Apr 27, 2025
-
What Is The Primary Difference Between Fermentation And Anaerobic Respiration
Apr 27, 2025
-
A 15 Foot Ladder Is Leaning Against A Wall
Apr 27, 2025
Related Post
Thank you for visiting our website which covers about Chi Square Test Of Homogeneity Vs Independence . We hope the information provided has been useful to you. Feel free to contact us if you have any questions or need further assistance. See you next time and don't miss to bookmark.