Chi Square Test For Homogeneity Vs Independence
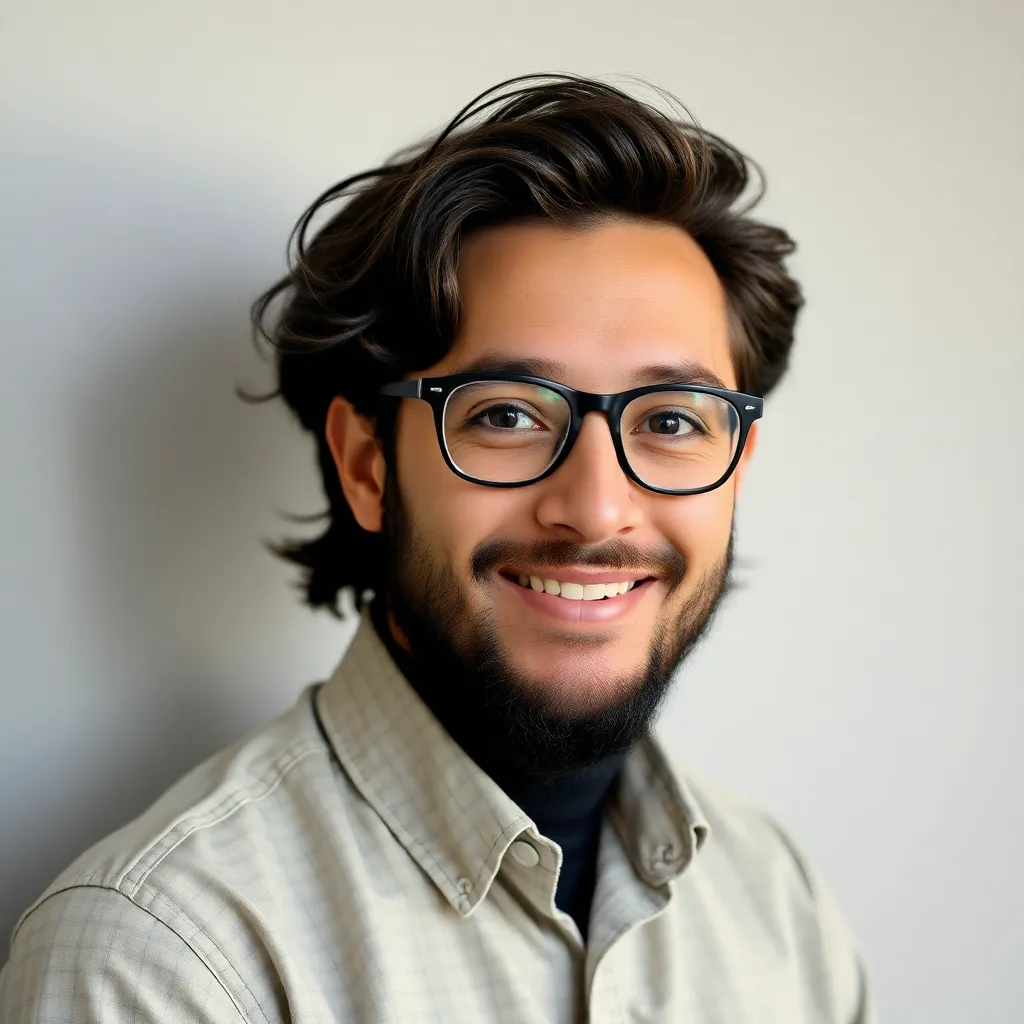
listenit
May 10, 2025 · 7 min read
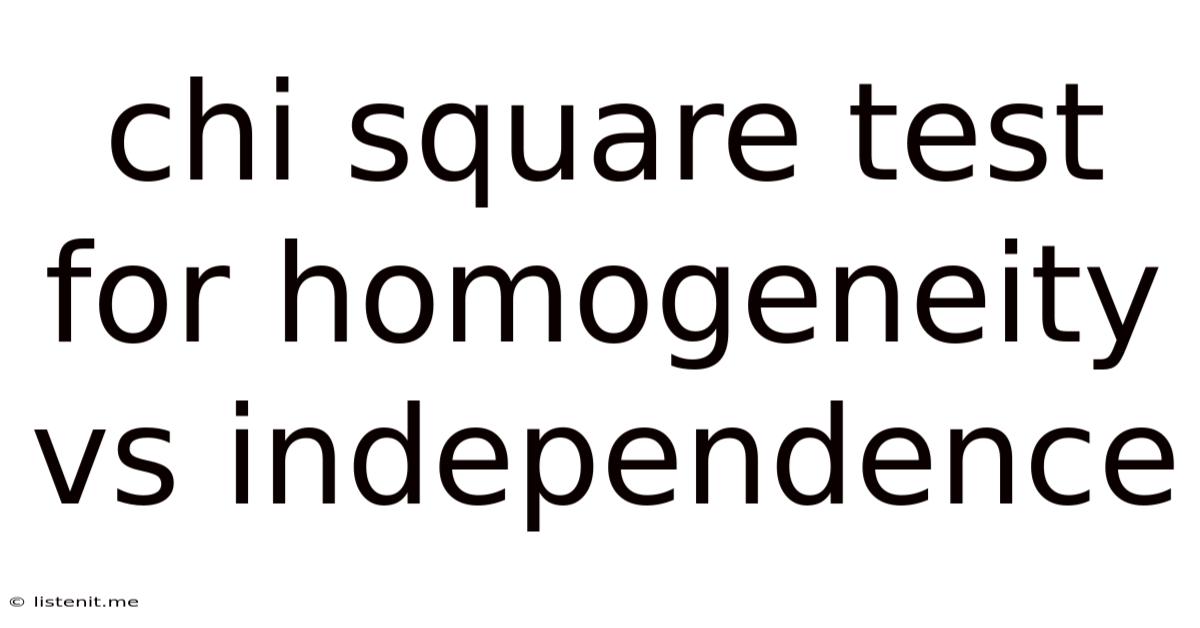
Table of Contents
Chi-Square Test: Homogeneity vs. Independence – Understanding the Differences
The chi-square test is a powerful statistical tool used to analyze categorical data. It determines whether there's a significant association between two categorical variables. However, there are two distinct applications of the chi-square test: the test of independence and the test of homogeneity. While both use the same underlying chi-square distribution, they address different research questions and involve slightly different interpretations. This article will delve into the nuances of each test, highlighting their similarities and crucial differences. We will explore their applications, assumptions, and interpretations to provide a comprehensive understanding of when to use which test.
Understanding Categorical Data and the Chi-Square Test
Before diving into the specifics of homogeneity and independence, it's crucial to grasp the nature of categorical data. Categorical data represents qualities or characteristics, not numerical quantities. Examples include gender (male/female), eye color (blue, brown, green), or educational attainment (high school, bachelor's, master's). The chi-square test examines the observed frequencies within these categories against the expected frequencies if no association existed between the variables.
The core idea behind the chi-square test is comparing observed frequencies with expected frequencies. A large difference between these frequencies suggests a significant association between the variables. This difference is quantified using the chi-square statistic, which follows a chi-square distribution. The p-value derived from this statistic helps determine the statistical significance of the association.
Chi-Square Test of Independence
The chi-square test of independence assesses whether two categorical variables are independent of each other within a single population. In simpler terms, it investigates whether the occurrence of one variable influences the probability of observing the other.
Research Question: Does the relationship between Variable A and Variable B differ significantly from what would be expected if they were unrelated?
Example: A researcher wants to determine if there's an association between smoking habits (smoker/non-smoker) and the development of lung cancer (yes/no). The researcher collects data from a single population sample.
Assumptions:
- Random Sampling: The data should represent a random sample from the population of interest.
- Expected Frequencies: The expected frequency for each cell in the contingency table should be at least 5. This assumption ensures the validity of the chi-square approximation. If this assumption is violated, Fisher's exact test might be a more appropriate alternative.
- Independence of Observations: Each observation should be independent of the others.
Steps to Perform a Chi-Square Test of Independence:
-
Formulate Hypotheses:
- Null Hypothesis (H0): There is no association between the two variables. They are independent.
- Alternative Hypothesis (H1): There is an association between the two variables. They are not independent.
-
Construct a Contingency Table: Organize the observed frequencies into a contingency table, showing the counts for each combination of categories.
-
Calculate Expected Frequencies: For each cell in the contingency table, calculate the expected frequency under the assumption of independence. This is done using the formula: Expected frequency = (Row total * Column total) / Grand total
-
Calculate the Chi-Square Statistic: Compute the chi-square statistic using the formula: χ² = Σ [(Observed frequency - Expected frequency)² / Expected frequency]
-
Determine the Degrees of Freedom: The degrees of freedom (df) are calculated as (number of rows - 1) * (number of columns - 1).
-
Find the p-value: Using the chi-square statistic and degrees of freedom, find the p-value from a chi-square distribution table or statistical software.
-
Interpret the Results: If the p-value is less than the significance level (typically 0.05), reject the null hypothesis. This indicates a statistically significant association between the two variables. If the p-value is greater than the significance level, fail to reject the null hypothesis. There is insufficient evidence to suggest an association.
Chi-Square Test of Homogeneity
The chi-square test of homogeneity determines whether the distribution of a single categorical variable is the same across two or more different populations. It assesses whether the proportions of each category are consistent across these populations.
Research Question: Is the distribution of Variable A the same across Population 1, Population 2, and Population 3?
Example: A researcher wants to determine if the proportion of men and women who prefer coffee over tea is the same in three different age groups (18-30, 31-50, 51+). The researcher collects data from separate samples representing each age group. Note that here, we only have one categorical variable (coffee/tea preference), but we're examining it across multiple populations (age groups).
Assumptions:
- Random Sampling: Data from each population should represent a random sample.
- Expected Frequencies: Expected frequencies for each cell should be at least 5.
- Independence of Observations: Observations within each sample should be independent, but observations across samples can be related.
Steps to Perform a Chi-Square Test of Homogeneity:
The steps are very similar to the independence test:
-
Formulate Hypotheses:
- Null Hypothesis (H0): The distribution of the categorical variable is the same across all populations.
- Alternative Hypothesis (H1): The distribution of the categorical variable is not the same across all populations.
-
Construct a Contingency Table: Organize the observed frequencies into a contingency table.
-
Calculate Expected Frequencies: Calculate the expected frequencies under the assumption of homogeneity. This is done slightly differently than in the independence test. The expected frequency for a cell is calculated as: Expected frequency = (Row total * Column total) / Grand total
-
Calculate the Chi-Square Statistic: Use the same formula as in the independence test.
-
Determine the Degrees of Freedom: The degrees of freedom are calculated as (number of rows - 1) * (number of columns - 1).
-
Find the p-value: Use the chi-square statistic and degrees of freedom to find the p-value.
-
Interpret the Results: Interpret the results as in the independence test. A significant p-value (less than 0.05) suggests that the distribution of the categorical variable differs significantly across the populations.
Key Differences Between Independence and Homogeneity Tests
Feature | Chi-Square Test of Independence | Chi-Square Test of Homogeneity |
---|---|---|
Research Question | Is there an association between two variables in a single population? | Is the distribution of a single variable the same across multiple populations? |
Number of Variables | Two categorical variables | One categorical variable across multiple populations |
Sampling | One sample from a single population | Multiple samples from different populations |
Hypotheses | H0: Variables are independent; H1: Variables are dependent | H0: Distributions are homogeneous; H1: Distributions are not homogeneous |
Expected Frequencies Calculation | (Row total * Column total) / Grand total | (Row total * Column total) / Grand total |
Choosing the Right Test: Independence vs. Homogeneity
The choice between the independence and homogeneity tests depends on the research question and how the data are collected. If you are investigating the relationship between two variables within a single sample, you use the test of independence. If you're comparing the distribution of a single variable across different populations with separate samples for each population, you use the test of homogeneity. While the calculations are very similar, the interpretation of the results differs based on the research context.
Practical Considerations and Limitations
-
Small Expected Frequencies: As mentioned earlier, the chi-square test assumes that expected frequencies are at least 5. If this assumption is violated, consider using Fisher's exact test, which is more accurate for small sample sizes.
-
Effect Size: While the p-value indicates statistical significance, it doesn't indicate the magnitude of the association or difference. Consider calculating effect size measures like Cramer's V or Phi coefficient to quantify the strength of the relationship.
-
Data Assumptions: It's critical to ensure that the assumptions of random sampling and independence of observations are met. Violations of these assumptions can affect the validity of the results.
Conclusion
The chi-square test is a valuable tool for analyzing categorical data. Understanding the distinction between the test of independence and the test of homogeneity is crucial for appropriate application and accurate interpretation. By carefully considering the research question, data collection method, and assumptions, researchers can effectively utilize these tests to draw meaningful conclusions about the relationships between categorical variables and distributions across different populations. Remember to always consider effect size measures to gain a comprehensive understanding of the observed relationship. Utilizing statistical software can significantly ease the computational burden and improve the accuracy of these analyses.
Latest Posts
Latest Posts
-
How To Subtract And Add Radicals
May 11, 2025
-
What Is The Percent By Mass Of Magnesium In Mgo
May 11, 2025
-
Percent By Mass Of Each Element In A Compound
May 11, 2025
-
Name Two Categories Used To Classify Properties Of Matter
May 11, 2025
-
What Is A Matched Pairs Design In Statistics
May 11, 2025
Related Post
Thank you for visiting our website which covers about Chi Square Test For Homogeneity Vs Independence . We hope the information provided has been useful to you. Feel free to contact us if you have any questions or need further assistance. See you next time and don't miss to bookmark.