Best Point Estimate Of The Population Mean
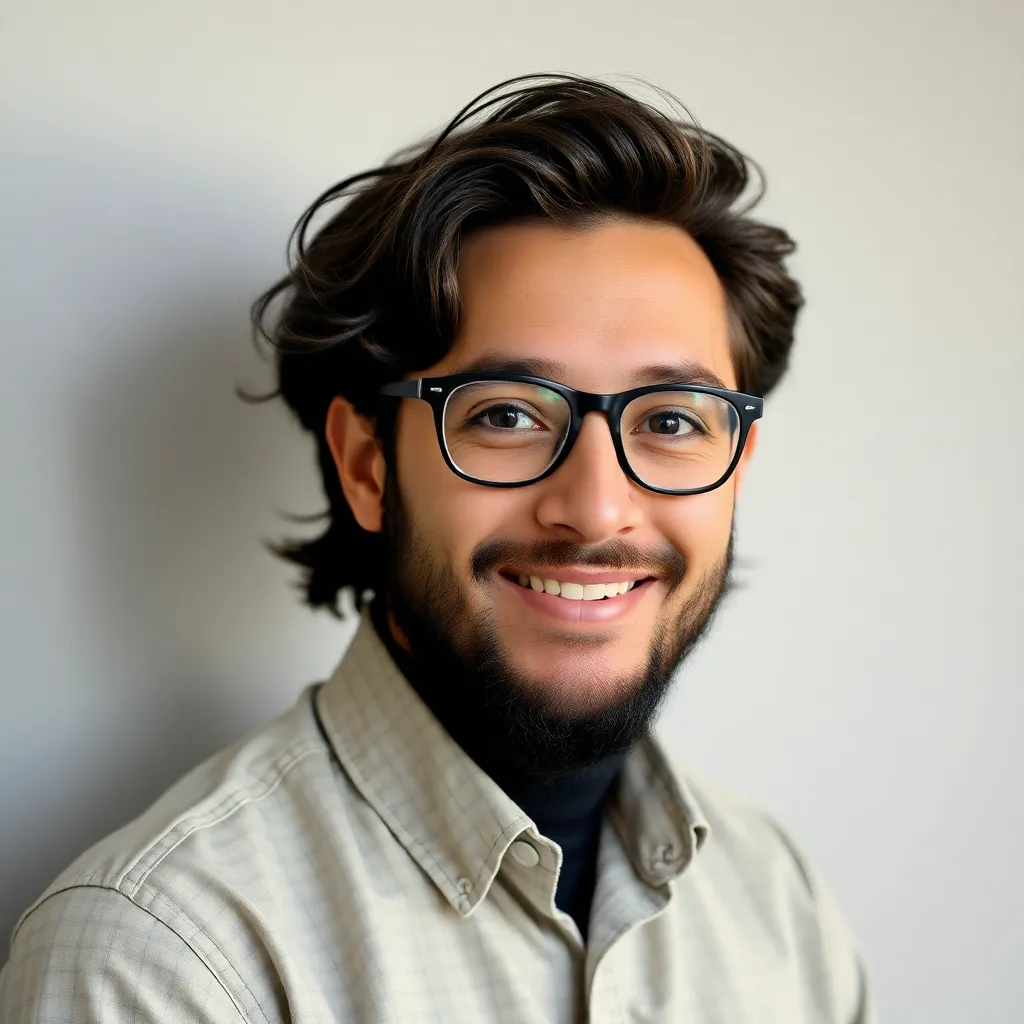
listenit
Apr 06, 2025 · 7 min read

Table of Contents
Best Point Estimate of the Population Mean: A Comprehensive Guide
Understanding and accurately estimating the population mean is a cornerstone of statistical inference. While we rarely have access to the entire population, we can use sample data to make informed estimations. This article delves deep into the best point estimate of the population mean, exploring its properties, underlying assumptions, and practical applications. We'll also examine the conditions under which it provides a reliable and robust estimate.
What is a Point Estimate?
A point estimate is a single value used to estimate an unknown population parameter. In the context of estimating the population mean (µ), the point estimate is a single number derived from sample data that serves as our best guess for the true population mean. It's important to understand that a point estimate is just an educated guess; it's unlikely to be exactly equal to the true population mean, but it aims to be as close as possible.
The Sample Mean as the Best Point Estimate
The best point estimate of the population mean (µ) is the sample mean (x̄). This is a fundamental concept in statistics. The sample mean is calculated by summing all the values in a sample and dividing by the number of observations (n):
x̄ = Σxᵢ / n
where:
- x̄ is the sample mean
- Σxᵢ is the sum of all observations in the sample
- n is the sample size
Why is the Sample Mean the Best?
The sample mean's superiority as a point estimate stems from several key properties:
1. Unbiasedness:
An estimator is unbiased if its expected value (the average of its values over many samples) is equal to the true population parameter. The sample mean is an unbiased estimator of the population mean. This means that, on average, the sample mean will accurately reflect the population mean. While a single sample mean might be off the mark, repeated sampling will yield sample means that center around the true population mean.
2. Efficiency:
Among all unbiased estimators, the sample mean is the most efficient. Efficiency refers to the precision of the estimator. The sample mean has the smallest variance (the measure of spread) among all unbiased estimators of the population mean. This means it tends to produce estimates closer to the true population mean compared to other estimators. A smaller variance implies less variability in the estimates, making the sample mean a more reliable point estimate.
3. Consistency:
The sample mean is a consistent estimator. Consistency means that as the sample size (n) increases, the sample mean converges towards the true population mean. In simpler terms, the larger your sample, the more accurate your estimate of the population mean will likely be. This property is crucial for achieving high accuracy in estimating the population mean.
Assumptions and Considerations
While the sample mean is the best point estimate, its reliability hinges on certain assumptions:
1. Random Sampling:
The sample data must be obtained through a random sampling process. Random sampling ensures that each member of the population has an equal chance of being selected, minimizing bias and increasing the representativeness of the sample. Non-random sampling methods can introduce bias, leading to inaccurate estimates of the population mean.
2. Independence:
The observations in the sample should be independent of each other. This means that the value of one observation doesn't influence the value of another. Violation of independence, such as in time series data with strong autocorrelation, can affect the accuracy and reliability of the sample mean as a point estimate.
3. Normality (for small samples):
For smaller sample sizes (generally n < 30), the underlying population from which the sample is drawn should be approximately normally distributed. While the Central Limit Theorem (CLT) mitigates this concern for larger samples, for small samples, non-normality can significantly impact the accuracy of the sample mean as a point estimate. In cases of non-normality, non-parametric methods might be preferred.
4. Outliers:
The presence of outliers (extreme values) can disproportionately influence the sample mean. Outliers can inflate or deflate the estimate, leading to an inaccurate representation of the population mean. It's crucial to identify and potentially handle outliers appropriately, perhaps through robust statistical techniques or careful examination of the data collection process.
Central Limit Theorem (CLT) and its Significance
The Central Limit Theorem is a cornerstone of statistical inference, particularly when dealing with the sample mean. The CLT states that the distribution of the sample means from repeated random samples of a population will be approximately normally distributed, regardless of the shape of the population distribution, as long as the sample size is sufficiently large (generally n ≥ 30).
This is incredibly important because it means that even if the population distribution is skewed or non-normal, the sample mean will still be a reliable estimate of the population mean, particularly with larger sample sizes. The CLT allows us to use normal distribution theory to make inferences about the population mean, even when we don't know the population distribution. It justifies the use of the sample mean as a robust point estimate in a wider range of scenarios.
Confidence Intervals: Beyond Point Estimates
While the sample mean provides a best point estimate, it's crucial to acknowledge its inherent uncertainty. A point estimate alone doesn't capture the range of plausible values for the population mean. This is where confidence intervals come into play.
A confidence interval provides a range of values within which the true population mean is likely to fall with a certain level of confidence (e.g., 95% confidence interval). The confidence interval incorporates the sample mean and the standard error of the mean (SEM), which quantifies the variability of the sample mean.
Confidence Interval = Sample Mean ± (Critical Value * Standard Error of the Mean)
The standard error of the mean is calculated as:
SEM = Standard Deviation / √n
The critical value depends on the chosen confidence level and the distribution of the sample mean (often approximated as normal due to the CLT).
Confidence intervals provide a more complete picture than point estimates alone, offering a measure of uncertainty associated with the estimation. They allow for a more nuanced interpretation of the results, acknowledging that the point estimate is just one possible value from a range of plausible values.
Practical Applications
The best point estimate of the population mean has wide-ranging applications across numerous fields:
- Market Research: Estimating average customer satisfaction, average purchase amounts, or the average age of a customer base.
- Quality Control: Monitoring the average weight of products or the average defect rate in a manufacturing process.
- Healthcare: Estimating the average effectiveness of a new drug, the average recovery time for a specific treatment, or the average blood pressure of a population.
- Environmental Science: Estimating average pollution levels, average species populations, or average rainfall in a region.
- Social Sciences: Estimating average income levels, average education levels, or average political opinions within a community.
In each of these cases, the sample mean serves as the best point estimate, providing a crucial starting point for further analysis and decision-making. It's important to remember that the reliability of this estimate hinges on proper sampling methods, consideration of assumptions, and, when appropriate, the incorporation of confidence intervals to quantify uncertainty.
Conclusion
The sample mean (x̄) stands as the best point estimate for the population mean (µ) due to its unbiasedness, efficiency, and consistency. However, its reliability depends on key assumptions like random sampling, independence of observations, and, for small samples, approximate normality of the population distribution. The Central Limit Theorem is crucial, allowing for the use of normal distribution theory even with non-normal populations, especially when sample sizes are large. While the sample mean provides a valuable point estimate, it's crucial to augment it with confidence intervals to account for the inherent uncertainty associated with estimating population parameters from sample data. This comprehensive approach ensures a more accurate, reliable, and robust understanding of the population mean. Remember that careful consideration of data quality, outliers, and limitations of the chosen method are paramount for achieving accurate and insightful results. This holistic approach allows for both precise estimation and a clear understanding of the limitations and uncertainties associated with statistical inference.
Latest Posts
Latest Posts
-
What Multiplies To 36 And Adds To
Apr 09, 2025
-
The Correct Order Of Mitosis Is
Apr 09, 2025
-
Is Primary Or Secondary Succession Faster
Apr 09, 2025
-
At What Point During Meiosis Do Homologous Chromosomes Pair Up
Apr 09, 2025
-
A Solution That Is At Equilibrium Must Be
Apr 09, 2025
Related Post
Thank you for visiting our website which covers about Best Point Estimate Of The Population Mean . We hope the information provided has been useful to you. Feel free to contact us if you have any questions or need further assistance. See you next time and don't miss to bookmark.