A. The Symbol For Sample Standard Deviation Is
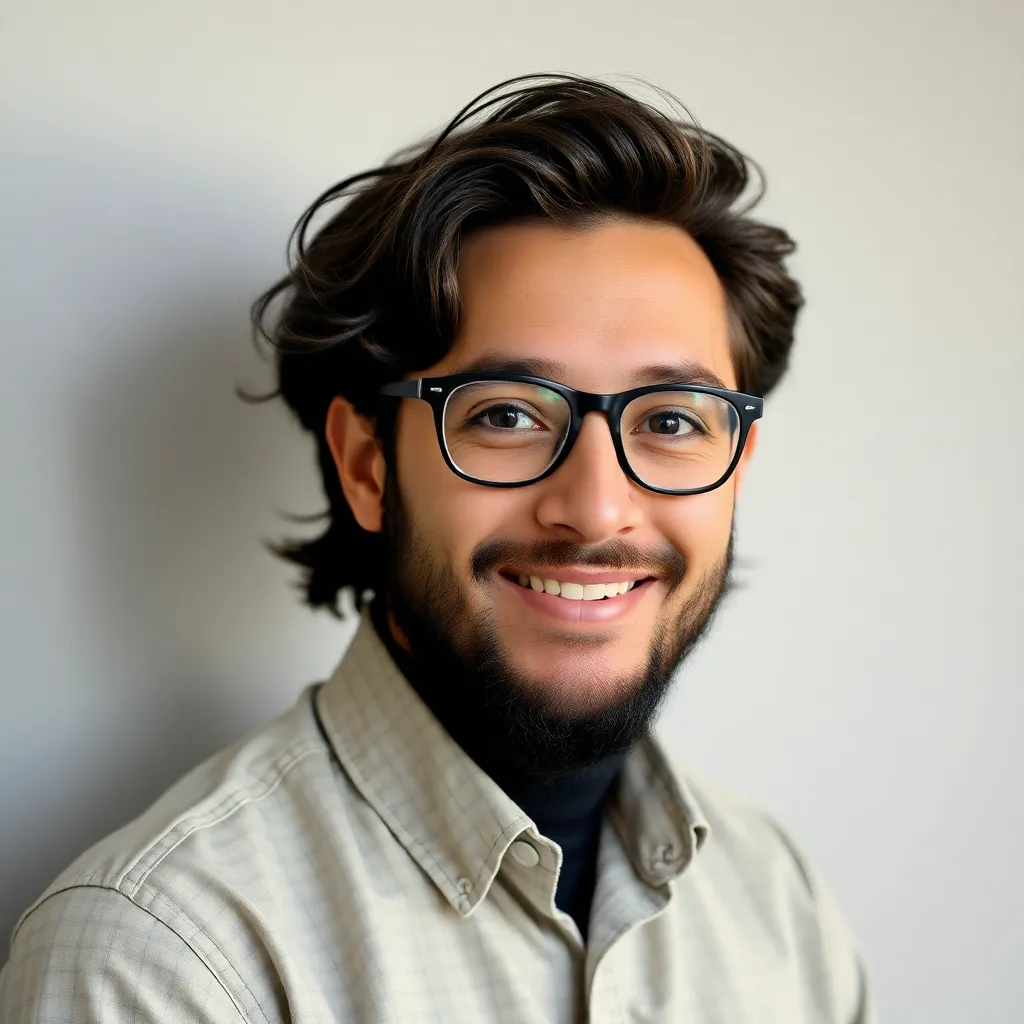
listenit
Apr 13, 2025 · 6 min read

Table of Contents
The Symbol for Sample Standard Deviation: A Deep Dive into Statistical Measures
Understanding statistical measures is crucial in various fields, from finance and healthcare to engineering and social sciences. One such fundamental measure is the standard deviation, which quantifies the amount of variation or dispersion in a dataset. While the population standard deviation uses the Greek letter sigma (σ), the symbol for sample standard deviation is denoted by the lowercase letter 's' or sometimes 'SD'. This seemingly small difference holds significant implications in statistical analysis. This article delves deep into the meaning, calculation, interpretation, and practical applications of the sample standard deviation, along with clarifying the distinction between sample and population standard deviations.
What is Standard Deviation?
Standard deviation measures the spread of a dataset around its mean (average). A low standard deviation indicates that the data points tend to be clustered closely around the mean, while a high standard deviation signifies that the data is more spread out. Understanding the standard deviation is crucial for interpreting data and drawing meaningful conclusions.
Population Standard Deviation vs. Sample Standard Deviation
Before we dive into the details of the sample standard deviation, it's crucial to understand the difference between population and sample standard deviation.
-
Population Standard Deviation (σ): This refers to the standard deviation of an entire population. Calculating the population standard deviation requires data from every member of the population. This is often impractical or impossible, especially when dealing with large populations. The formula for population standard deviation is:
σ = √[Σ(xi - μ)² / N]
Where:
- Σ represents the sum
- xi represents each individual data point
- μ represents the population mean
- N represents the total number of data points in the population
-
Sample Standard Deviation (s): This is an estimate of the population standard deviation, calculated using data from a sample of the population. Since we're working with a sample, we use a slightly different formula to account for the fact that the sample mean might not perfectly represent the population mean. The formula for sample standard deviation is:
s = √[Σ(xi - x̄)² / (n - 1)]
Where:
- Σ represents the sum
- xi represents each individual data point in the sample
- x̄ represents the sample mean
- n represents the total number of data points in the sample
The key difference lies in the denominator: The population standard deviation uses 'N' (the total population size), while the sample standard deviation uses 'n-1' (the sample size minus 1). This 'n-1' is known as Bessel's correction, and it helps to provide a less biased estimate of the population standard deviation when using a sample. Without Bessel's correction, the sample standard deviation would tend to underestimate the population standard deviation, particularly for smaller sample sizes.
Why Use 'n-1' (Bessel's Correction)?
The use of 'n-1' instead of 'n' in the sample standard deviation formula is a crucial aspect that warrants further explanation. This adjustment is known as Bessel's correction, named after Friedrich Bessel, and its purpose is to reduce bias in the estimation of the population standard deviation.
Imagine you have a very small sample, perhaps only two data points. If you use 'n' in the denominator, the sample standard deviation will always be smaller than it should be, leading to an underestimation of the population's variability. The 'n-1' in the denominator increases the value of the standard deviation, providing a more accurate and less biased estimate.
Degrees of Freedom: The use of 'n-1' is also related to the concept of "degrees of freedom." When calculating the sample mean, one degree of freedom is lost because the sample mean is used to calculate the deviations from the mean. This means that only (n-1) of the deviations are independent. Using 'n-1' in the denominator accounts for this loss of a degree of freedom.
Calculating Sample Standard Deviation: A Step-by-Step Guide
Let's illustrate the calculation of the sample standard deviation with a concrete example. Suppose we have the following sample dataset representing the weights (in kg) of five randomly selected individuals: 65, 70, 72, 68, 75.
Step 1: Calculate the sample mean (x̄):
x̄ = (65 + 70 + 72 + 68 + 75) / 5 = 70 kg
Step 2: Calculate the deviations from the mean (xi - x̄):
- 65 - 70 = -5
- 70 - 70 = 0
- 72 - 70 = 2
- 68 - 70 = -2
- 75 - 70 = 5
Step 3: Square the deviations:
- (-5)² = 25
- 0² = 0
- 2² = 4
- (-2)² = 4
- 5² = 25
Step 4: Sum the squared deviations:
25 + 0 + 4 + 4 + 25 = 58
Step 5: Divide the sum of squared deviations by (n - 1):
58 / (5 - 1) = 14.5
Step 6: Take the square root:
√14.5 ≈ 3.81 kg
Therefore, the sample standard deviation (s) for this dataset is approximately 3.81 kg. This means that the weights in the sample are, on average, about 3.81 kg away from the mean weight of 70 kg.
Interpreting the Sample Standard Deviation
The interpretation of the sample standard deviation depends on the context of the data. A larger standard deviation suggests greater variability within the dataset, while a smaller standard deviation indicates that the data points are more tightly clustered around the mean.
For instance, in the weight example above, a sample standard deviation of 3.81 kg indicates moderate variability in the weights of the individuals in the sample. If the standard deviation were much smaller (e.g., 1 kg), it would suggest that the weights are very similar. Conversely, a much larger standard deviation (e.g., 10 kg) would imply significant variability in the weights.
Applications of Sample Standard Deviation
The sample standard deviation is a vital tool in numerous statistical applications, including:
-
Hypothesis testing: It's used to assess the statistical significance of differences between sample means or to test whether a sample mean differs significantly from a hypothesized population mean (t-tests).
-
Confidence intervals: It's used to construct confidence intervals, which provide a range of values within which the true population mean is likely to fall.
-
Quality control: In manufacturing processes, the sample standard deviation is used to monitor the variability of product characteristics and ensure consistency.
-
Risk management: In finance, it is used to measure the volatility of investments. A higher standard deviation indicates greater risk.
-
Data analysis and visualization: It informs the choice of appropriate statistical methods and graphical representations, aiding in understanding data distribution and patterns.
-
Regression analysis: The standard deviation of residuals (the differences between observed and predicted values) is used to assess the goodness of fit of a regression model.
Choosing Between Sample and Population Standard Deviation
The choice between using sample and population standard deviation depends entirely on the nature of the data. If you have data for the entire population, then you should calculate the population standard deviation. However, in most real-world scenarios, you only have data from a sample. In such cases, you must use the sample standard deviation to estimate the population standard deviation.
Conclusion: The Importance of 's' in Statistical Analysis
The symbol 's' for sample standard deviation is not merely a notation; it represents a crucial concept in statistical inference. Understanding its calculation, interpretation, and applications is essential for anyone working with data. The accurate estimation of population parameters from sample data is paramount in various fields, and the sample standard deviation, calculated with Bessel's correction, plays a vital role in achieving this accuracy. By correctly interpreting the sample standard deviation, we can draw meaningful conclusions and make informed decisions based on the data at hand. Remember that the 's' represents more than just a number; it represents the variability inherent in our data, providing critical insights into the phenomenon under investigation. Mastering the concept of sample standard deviation is a cornerstone of sound statistical practice and enhances the validity of your conclusions.
Latest Posts
Latest Posts
-
Which Substance Is An Arrhenius Base
Apr 14, 2025
-
What Is The Fraction Of 23
Apr 14, 2025
-
Water Exhibits All Of The Following Characteristics Except
Apr 14, 2025
-
Which Part Of An Atom Has Most Of Its Mass
Apr 14, 2025
-
Transcribe And Translate The Following Dna Sequence
Apr 14, 2025
Related Post
Thank you for visiting our website which covers about A. The Symbol For Sample Standard Deviation Is . We hope the information provided has been useful to you. Feel free to contact us if you have any questions or need further assistance. See you next time and don't miss to bookmark.