A Factor In An Experiment That Can Change Is A
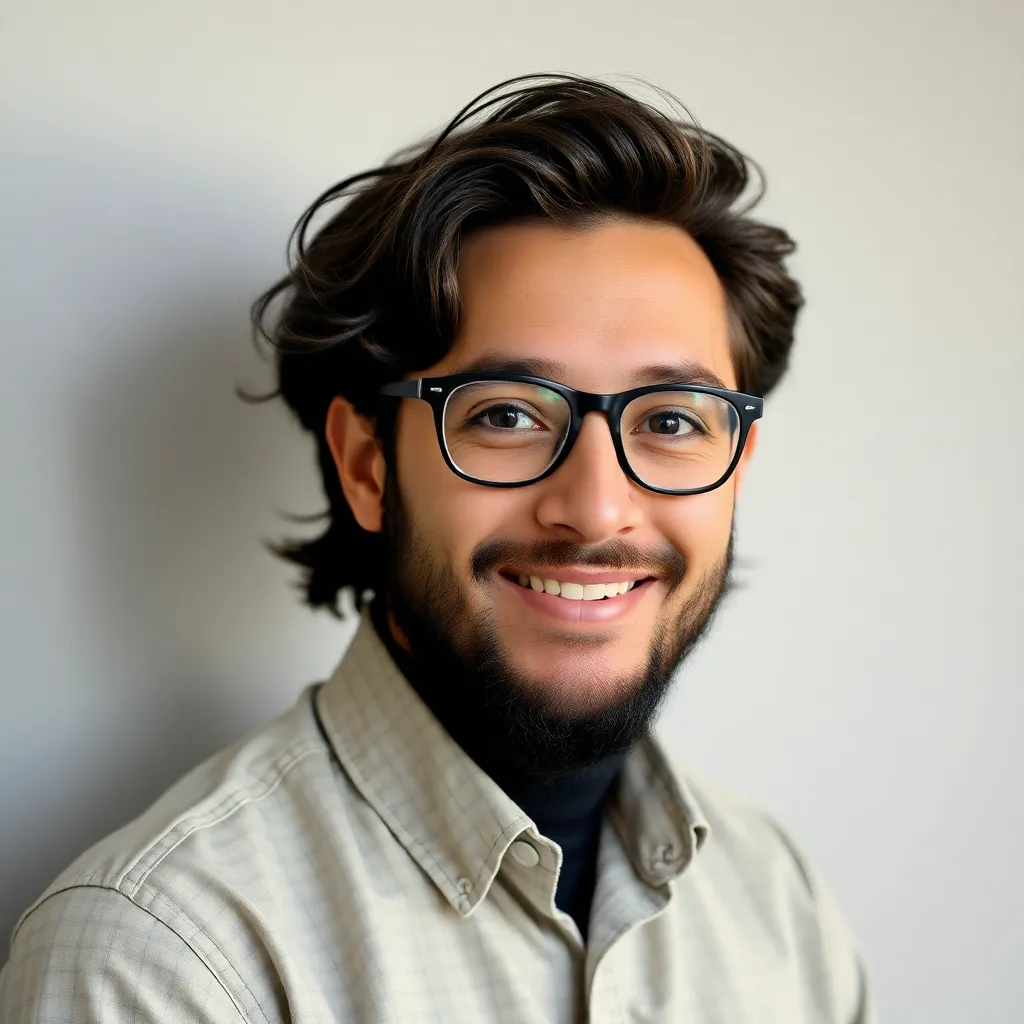
listenit
Apr 27, 2025 · 7 min read

Table of Contents
A Factor in an Experiment That Can Change Is a Variable
In the realm of scientific inquiry, understanding the nuances of experimental design is paramount. A well-structured experiment hinges on the careful identification and manipulation of various elements. Central to this process is the concept of a variable, a factor that can change or be changed. This article delves deep into the definition, types, and crucial role of variables in experiments, providing a comprehensive guide for both beginners and seasoned researchers.
Understanding Variables: The Cornerstones of Experimentation
A variable, at its core, represents any characteristic, number, or quantity that can be measured or counted. Its value can vary across individuals, groups, times, or places. Think of it as a characteristic that's subject to change, exhibiting variation within a defined context. This inherent variability is precisely what makes variables so essential in experimental research. Without them, we wouldn't have anything to measure, compare, or analyze.
The Importance of Defining Variables Clearly
Before embarking on any experiment, meticulously defining your variables is crucial. Ambiguity in variable definition can lead to flawed results and compromised conclusions. This definition must be precise and unambiguous, using operational definitions – that is, definitions that specify exactly how the variable will be measured or manipulated.
For example, if your experiment explores the effect of "stress" on "academic performance," you can't simply rely on vague definitions. You need to operationalize these variables:
- Stress: This might be measured using a standardized stress questionnaire, physiological indicators like heart rate variability, or self-reported stress levels on a numerical scale.
- Academic performance: This could be operationalized as GPA, scores on specific tests, or the number of assignments completed successfully.
This precision ensures that other researchers can replicate your study and obtain similar results, enhancing the reproducibility and reliability of your findings.
Types of Variables: A Categorical Overview
Variables are broadly categorized into several types, each playing a distinct role in the experimental design. Understanding these distinctions is crucial for interpreting results accurately and drawing valid conclusions.
1. Independent Variables (IV): The Manipulated Factor
The independent variable (IV) is the variable that the researcher manipulates or changes. It's the presumed cause in the cause-and-effect relationship being investigated. In a well-designed experiment, the researcher systematically alters the independent variable to observe its impact on the dependent variable. For example, in an experiment testing the effect of fertilizer on plant growth, the type and amount of fertilizer would be the independent variable.
2. Dependent Variables (DV): The Measured Outcome
The dependent variable (DV) is the variable that is measured or observed. It's the presumed effect in the cause-and-effect relationship. The dependent variable is expected to change in response to the manipulations of the independent variable. In the plant growth experiment, the height of the plants or their overall biomass would be the dependent variable.
3. Controlled Variables (CV): Maintaining Consistency
Controlled variables (CVs), also known as constant variables, are factors that are kept constant or controlled throughout the experiment. Their values remain unchanged to prevent them from influencing the relationship between the independent and dependent variables. Controlling these variables enhances the internal validity of the experiment, ensuring that any observed changes in the dependent variable are genuinely due to the manipulation of the independent variable, not confounding factors. In the plant growth experiment, controlled variables might include the amount of sunlight, water, and the type of soil used for all plants.
4. Confounding Variables: The Unwanted Influences
Confounding variables are extraneous variables that are not controlled for and can influence the results of an experiment. They obscure the true relationship between the independent and dependent variables, leading to inaccurate interpretations. For instance, if different groups of plants in the fertilizer experiment received varying amounts of sunlight, sunlight would become a confounding variable, affecting plant growth independently of the fertilizer. Careful experimental design aims to minimize or eliminate the influence of confounding variables.
5. Extraneous Variables: Potential Interferences
Extraneous variables are variables that are not of primary interest but could potentially influence the results of the experiment. They are similar to confounding variables, but unlike confounding variables, they do not necessarily influence the results in this particular experiment. However, they are still important to consider and control, as they could affect the generalizability of the findings to other situations. For example, the ambient temperature in the greenhouse could be an extraneous variable affecting plant growth, but if it remains relatively constant across all plant groups, it is less likely to be a confounding variable.
6. Intervening Variables: The Hidden Mediators
Intervening variables are hypothetical variables that are not directly observable but are believed to mediate the relationship between the independent and dependent variables. They represent the underlying mechanisms or processes that explain how the independent variable influences the dependent variable. These variables are often inferred based on theoretical considerations and are often difficult to directly measure. For example, in the plant growth experiment, an intervening variable might be the plant's internal nutrient uptake efficiency, mediating the relationship between the fertilizer (IV) and plant growth (DV).
7. Categorical Variables: Qualitative Differences
Categorical variables, also known as qualitative variables, represent categories or groups. They describe qualities or characteristics rather than numerical values. Examples include gender (male/female), eye color (blue/brown/green), or type of treatment (drug A/drug B/placebo).
8. Continuous Variables: Numerical Measurements
Continuous variables, also known as quantitative variables, are variables that can take on any value within a given range. They are measured on a continuous scale, such as height, weight, temperature, or time.
9. Discrete Variables: Whole Number Counts
Discrete variables are variables that can only take on specific, separate values. They are often whole numbers, representing counts or categories. Examples include the number of students in a class, the number of cars in a parking lot, or the number of errors made on a test.
The Crucial Role of Variables in Different Research Designs
The types of variables used and how they are manipulated vary across different research designs. Understanding this interplay is vital for interpreting research findings appropriately.
Experimental Research: Manipulating Variables
In experimental research, the researcher actively manipulates the independent variable to observe its effect on the dependent variable while controlling extraneous variables. This approach allows for causal inferences—determining whether changes in the independent variable cause changes in the dependent variable. Random assignment of participants to different experimental conditions is crucial in experimental research to minimize bias and enhance the generalizability of the findings.
Correlational Research: Exploring Relationships
Correlational research explores the relationship between two or more variables without manipulating any of them. It examines the degree to which variables are associated, but it does not establish causality. A correlation merely indicates that two variables tend to vary together, but it does not prove that one variable causes changes in the other. Confounding variables can easily confound correlational studies, making causal interpretations problematic.
Observational Research: Observing and Recording
Observational research involves observing and recording the behavior of individuals or groups without manipulating any variables. It is useful for studying phenomena in natural settings, but it is limited in its ability to establish causal relationships.
Operationalization: The Bridge Between Concept and Measurement
As mentioned earlier, operationalization is the process of defining a variable in terms of how it will be measured or manipulated. This is crucial for ensuring the clarity, reproducibility, and validity of research. A poorly operationalized variable can lead to ambiguous results and hinder the ability to draw meaningful conclusions.
For example, consider the variable "intelligence." This is a broad concept that can be operationalized in many different ways, including IQ scores, academic performance, problem-solving abilities, or creativity tests. The specific operational definition chosen will significantly influence the results obtained.
Conclusion: Variables – The Foundation of Scientific Inquiry
Variables are the fundamental building blocks of scientific inquiry. Understanding the different types of variables, their roles in experimental design, and the importance of careful operationalization is essential for conducting rigorous and meaningful research. By clearly defining and manipulating variables, researchers can gain valuable insights into the world around us, contributing to the advancement of knowledge and informing evidence-based decision-making. The meticulous consideration and manipulation of variables are not merely technicalities; they are the heart and soul of successful scientific investigation. A well-defined experiment, grounded in a thorough understanding of variables, forms the bedrock of credible scientific findings. Therefore, mastering the nuances of variables is a critical step in becoming a proficient researcher.
Latest Posts
Latest Posts
-
How Many Protons Electrons And Neutrons Are In Neon
Apr 27, 2025
-
What Color Is The Combination Of All Colors
Apr 27, 2025
-
Difference Between Percent And Relative Abundance
Apr 27, 2025
-
Matter Is Anything That Occupies Space And Has Mass
Apr 27, 2025
-
Which Monomer Is Used To Build Rna And Dna
Apr 27, 2025
Related Post
Thank you for visiting our website which covers about A Factor In An Experiment That Can Change Is A . We hope the information provided has been useful to you. Feel free to contact us if you have any questions or need further assistance. See you next time and don't miss to bookmark.